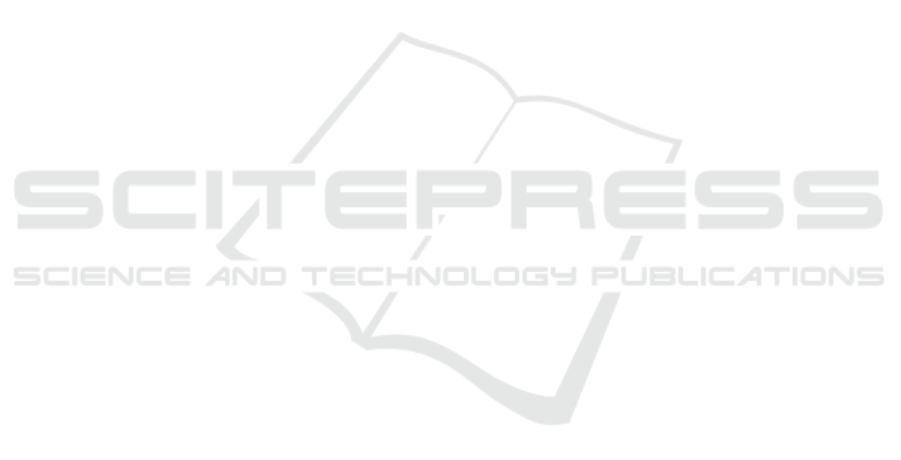
replication. IEEE Transactions on Parallel and Dis-
tributed Systems, 25(7):1787–1796.
Calzarossa, M. C., Della Vedova, M. L., and Tessera, D.
(2019). A methodological framework for cloud re-
source provisioning and scheduling of data parallel
applications under uncertainty. Future Generation
Computer Systems, 93:212–223.
Coutinho, R. d. C., Drummond, L. M., Frota, Y., and
de Oliveira, D. (2015). Optimizing virtual machine al-
location for parallel scientific workflows in federated
clouds. Future Generation Computer Systems, 46:51–
68.
Deb, K. (2015). Multi-objective evolutionary algorithms.
In Springer handbook of computational intelligence,
pages 995–1015. Springer.
Diwekar, U. M. (2020). Introduction to applied optimiza-
tion, volume 22. Springer Nature.
Fard, H. M., Prodan, R., and Fahringer, T. (2014). Multi-
objective list scheduling of workflow applications in
distributed computing infrastructures. Journal of Par-
allel and Distributed Computing, 74(3):2152–2165.
Ferdaus, M. H., Murshed, M., Calheiros, R. N., and Buyya,
R. (2017). An algorithm for network and data-aware
placement of multi-tier applications in cloud data cen-
ters. Journal of Network and Computer Applications,
98:65–83.
Heilig, L., Lalla-Ruiz, E., and Voß, S. (2016). A cloud bro-
kerage approach for solving the resource management
problem in multi-cloud environments. Computers &
Industrial Engineering, 95:16–26.
Heilig, L., Lalla-Ruiz, E., and Voß, S. (2020). Model-
ing and solving cloud service purchasing in multi-
cloud environments. Expert systems with applica-
tions, 147:113165.
Hu, H., Li, Z., Hu, H., Chen, J., Ge, J., Li, C., and Chang,
V. (2018). Multi-objective scheduling for scientific
workflow in multicloud environment. Journal of Net-
work and Computer Applications, 114:108–122.
Li, Z., Ge, J., Yang, H., Huang, L., Hu, H., Hu, H., and
Luo, B. (2016). A security and cost aware scheduling
algorithm for heterogeneous tasks of scientific work-
flow in clouds. Future Generation Computer Systems,
65:140–152.
Madni, S. H. H., Abd Latiff, M. S., Coulibaly, Y., et al.
(2016). Resource scheduling for infrastructure as a
service (iaas) in cloud computing: Challenges and op-
portunities. Journal of Network and Computer Appli-
cations, 68:173–200.
Mao, M. and Humphrey, M. (2011). Auto-scaling to min-
imize cost and meet application deadlines in cloud
workflows. In SC’11: Proceedings of 2011 Interna-
tional Conference for High Performance Computing,
Networking, Storage and Analysis, pages 1–12. IEEE.
Mitra, K. (2013). Chance constrained programming to han-
dle uncertainty in nonlinear process models. Multi-
Objective Optimization in Chemical Engineering: De-
velopments and Applications, pages 183–215.
Mohammadi, S., Pedram, H., and PourKarimi, L. (2018).
Integer linear programming-based cost optimization
for scheduling scientific workflows in multi-cloud
environments. The Journal of Supercomputing,
74(9):4717–4745.
Ning, C. and You, F. (2019). Optimization under uncer-
tainty in the era of big data and deep learning: When
machine learning meets mathematical programming.
Computers & Chemical Engineering, 125:434–448.
Odetayo, B., Kazemi, M., MacCormack, J., Rosehart,
W. D., Zareipour, H., and Seifi, A. R. (2018). A
chance constrained programming approach to the in-
tegrated planning of electric power generation, natural
gas network and storage. IEEE Transactions on Power
Systems, 33(6):6883–6893.
Pandey, S., Wu, L., Guru, S. M., and Buyya, R. (2010).
A particle swarm optimization-based heuristic for
scheduling workflow applications in cloud computing
environments. In 2010 24th IEEE international con-
ference on advanced information networking and ap-
plications, pages 400–407. IEEE.
Ramamurthy, A., Saurabh, S., Gharote, M., and Lodha, S.
(2020). Selection of cloud service providers for host-
ing web applications in a multi-cloud environment.
In 2020 IEEE International Conference on Services
Computing (SCC), pages 202–209. IEEE.
Rodriguez, M. A. and Buyya, R. (2014). Deadline based re-
source provisioningand scheduling algorithm for sci-
entific workflows on clouds. IEEE transactions on
cloud computing, 2(2):222–235.
Tchernykh, A., Schwiegelsohn, U., Talbi, E.-g., and
Babenko, M. (2019). Towards understanding uncer-
tainty in cloud computing with risks of confidentiality,
integrity, and availability. Journal of Computational
Science, 36:100581.
Wang, X. and Ning, Y. (2017). Uncertain chance-
constrained programming model for project schedul-
ing problem. Journal of the operational research so-
ciety, pages 1–9.
Wen, Y., Chen, Z., Chen, T., Liu, J., and Kang, G. (2012). A
particle swarm optimization algorithm for batch pro-
cessing workflow scheduling. In 2012 Second Inter-
national Conference on Cloud and Green Computing,
pages 645–649. IEEE.
Zeng, L., Veeravalli, B., and Zomaya, A. Y. (2015). An
integrated task computation and data management
scheduling strategy for workflow applications in cloud
environments. Journal of Network and Computer Ap-
plications, 50:39–48.
Zhu, Z., Zhang, G., Li, M., and Liu, X. (2015). Evolu-
tionary multi-objective workflow scheduling in cloud.
IEEE Transactions on parallel and distributed Sys-
tems, 27(5):1344–1357.
Multi-objective Optimization for Virtual Machine Allocation in Computational Scientific Workflow under Uncertainty
247