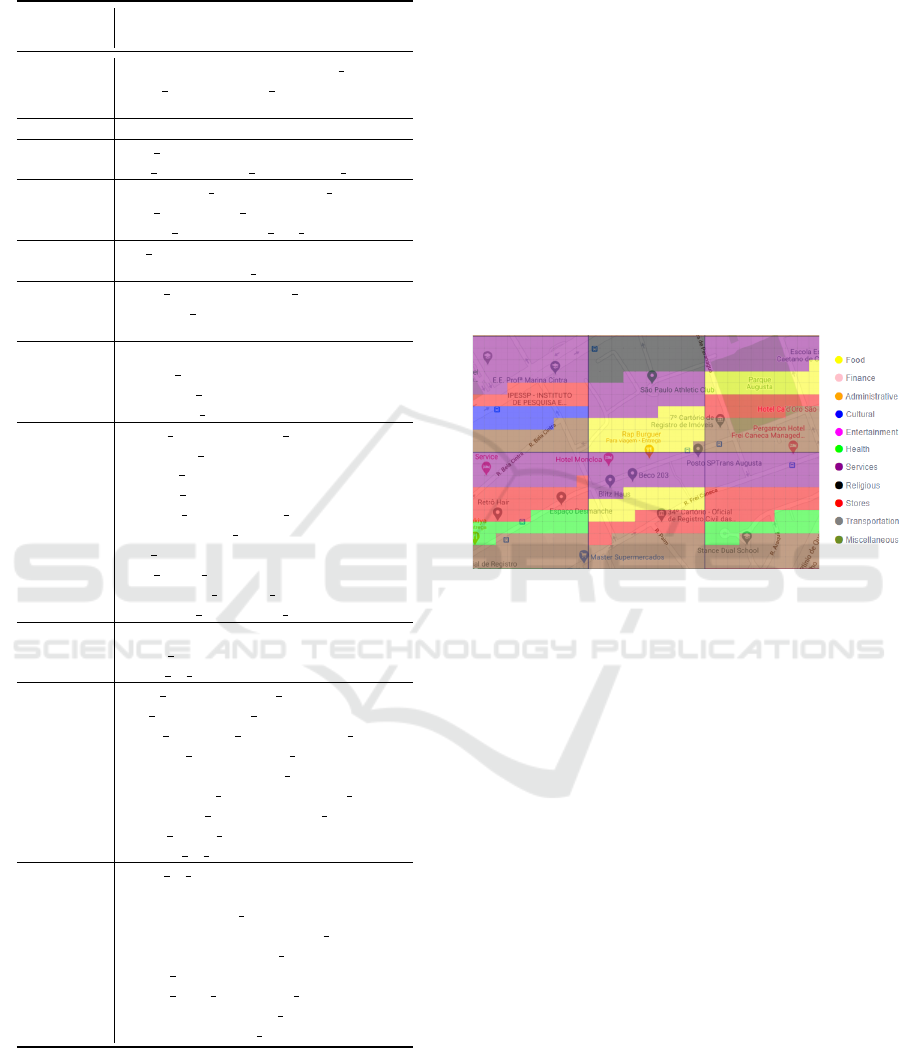
Table 2: Clustering Google Maps categories into macro cat-
egories (Macro Cat.).
Macro
Category Category from Google Maps
Food
bakery, bar, cafe, food, liquor store,
meal delivery, meal takeaway,
restaurant
Finance accounting, atm, bank, finance
Admin.
city hall, courthouse, embassy, police,
fire station, local government office
Transport.
airport, bus station, subway station,
taxi stand, train station,
transit station, light rail station
Cultural
art gallery, library, school,
university, movie theater, museum
Entert.
night club, amusement park,
bowling lley, campground, zoo,
aquarium, stadium, casino
Health
pharmacy, physiotherapist,
beauty salon, dentist, doctor,
gym, hair care, hospital,
veterinary care, health, spa
Services
travel agency, funeral home,
park, post office, parking,
roofing contractor, locksmith,
general contractor, lodging,
moving company, car repair,
electrician, car rental, laundry,
gas station, plumber, painter,
real estate agency, lawyer,
recreational vehicle park,
insurance agency, car wash
Religious
mosque, cemetery, church,
hindu temple, synagogue,
place of worship
Stores
shoe store, shopping mall,
pet store, bicycle store,
book store, car dealer, , movie rental
clothing store, jewelry store,
florist, store, furniture store,
convenience store, department store,
electronics store, hardware store,
home goods store, storage,
grocery or supermarket
Misc.
point of interest, establishment,
country, floor, intersection,
locality, natural feature, geocode,
colloquial, area, room, post box,
neighborhood, postal code,
postal town, political,
postal code prefix and suffix,
premise, route, street address,
subpremise, street number
occurrence in the cell (b). The most prominent POI
macro category appears at the top of the cell, then the
second highlighted macro category, the third macro
category highlighted comes next, and the fourth repre-
sents all the remaining macro categories aggregated.
The result of dividing cells according to their most
common macro category is shown in Figure 4 for the
six cells presented in Figure 3. Notice that each color
represents a macro category and the color occupation
in each cell represents the proportion of activities re-
lated to that macro category. Finally, the sum of all
the remaining macro categories is shown in propor-
tion and represented by the brown color. In this exam-
ple, the purple color represents the services macro cat-
egory, red is related to stores, yellow represents food,
green represents health, blue represents cultural, and
brown represents all other macro categories present in
the cell. The analyst can notice the categories that are
not predominant in each cell by looking at the legend
that describes each macro category employed in the
visualization.
Figure 4: Using colors to encode the most common macro
category in a cell. This region is particularly represented by
services and stores macro categories.
In the cluster analysis view, each cell is labeled with
a different color for each data group partitioned by
the clustering algorithm. Figure 5 shows the result of
cluster analysis on the same six cells highlighted in
Figure 3. Notice that five out of the six cells belong to
the same cluster (cells in red) and one cell has differ-
ent features (cell in green). The user can understand
the pattern adopted by the clustering algorithm to sep-
arate groups of data. We must highlight that the colors
in the cluster analysis do not relate to the colors used
for encoding the macro categories.
To complement the analysis of macro categories
and assist in the interpretation of clustering results,
PlaceProfile also uses a Radar Chart visualisation to
help users to perceive predominant activities for each
cell. Interactivity is present in this view, allowing the
user to select cells of interest to analyse and make
comparisons. Figure 6 shows the differences between
the two groups, the red cells stand out for macro cat-
egories of services and store activities while in the
green cell the highlight is for stores, services, and
health.
ICEIS 2021 - 23rd International Conference on Enterprise Information Systems
510