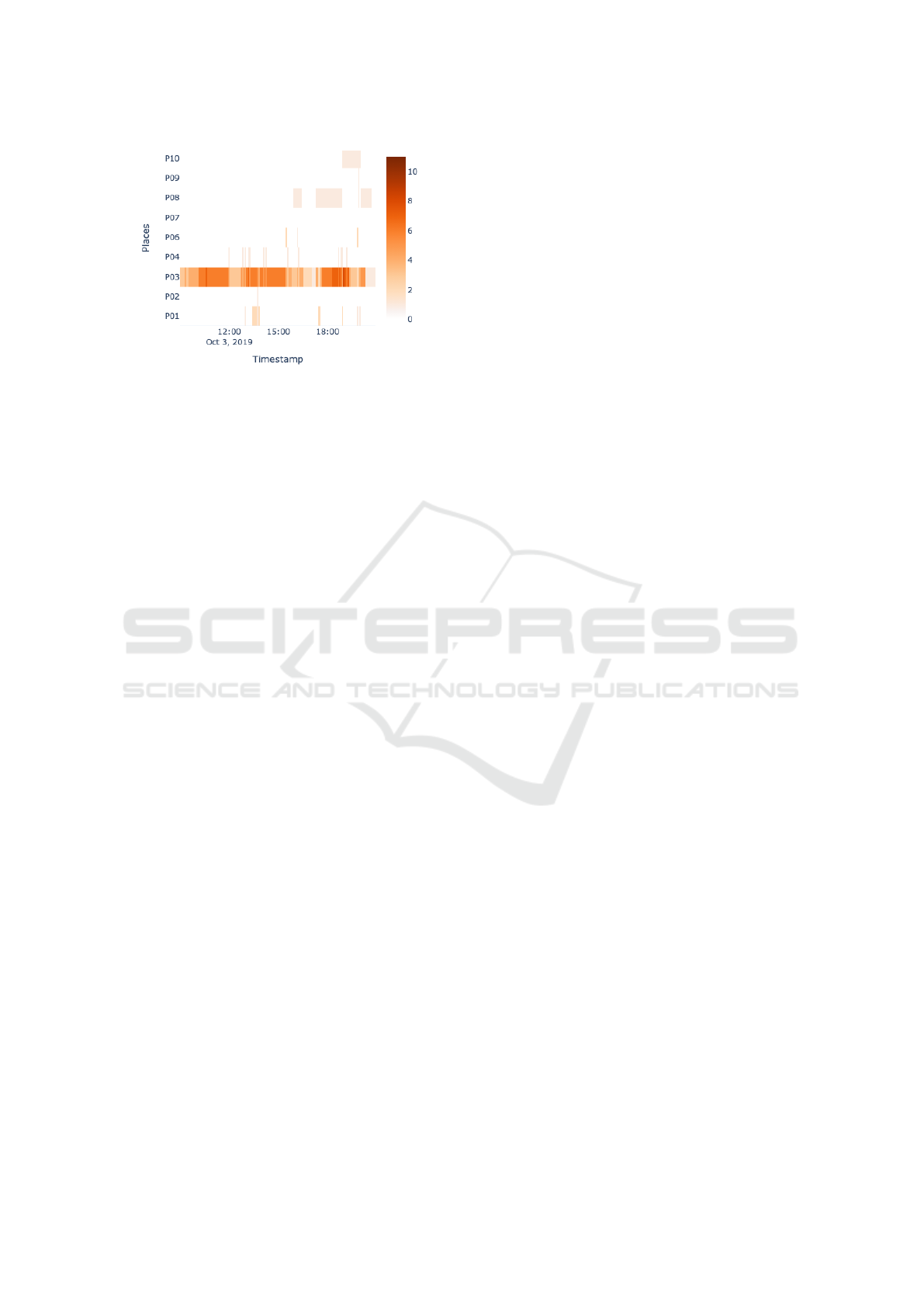
Figure 10: Occupancy of individuals in places over time.
5 CONCLUSIONS
We proposed a visual tool prototype and demon-
strated it with some charts and animations to visualize
a real-case scenario of an indoor movement dataset
generated in two university buildings used by faculty,
staff, and students. In this set, we explored the data
visually to answer some questions about individuals’
movements. We visualized the relations between in-
dividuals and places and the inter-relations with in-
dividuals × individuals and places × places. The
contribution is how we adapted the charts and ani-
mations to answer questions in this context and to
enhance analysts’ experience by reducing the time
spent on the analysis. Our future work aimed to in-
corporate more chart integration, filters, and function-
alities such as event thresholds in the dash tool to
(a) to facilitate environmental automation or monitor-
ing for social-distance control for COVID-19, to ad-
just the air-conditioning temperature, and to estimate
resources available; (b) to identify outliers and predict
movement online for indoor environments; and (c) to
make it more usable for human operators to derive in-
sights about the indoor movements. Finally, we plan
to test the tool by using another dataset and to conduct
an empirical study to evaluate its efficiency and ease
of use.
ACKNOWLEDGEMENTS
This work was partially supported by CNPq - Con-
selho Nacional de Desenvolvimento Cient
´
ıfico e Tec-
nol
´
ogico.
REFERENCES
Andrienko, N. and Andrienko, G. (2013). Visual analyt-
ics of movement: An overview of methods, tools and
procedures. Information Visualization, 12(1):3–24.
Curran, K. (2018). Hybrid passive and active approach to
tracking movement within indoor environments. IET
Communications, 12(10):1188–1194.
Dardari, D., Closas, P., and Djuri
´
c, P. M. (2015). Indoor
tracking: Theory, methods, and technologies. IEEE
Transactions on Vehicular Technology, 64(4):1263–
1278.
Dogan, O. (2020). Discovering customer paths from loca-
tion data with process mining. European Journal of
Teaching and Education, 2(1):55–60.
Dogan, O., Bayo-Monton, J.-L., Fernandez-Llatas, C., and
Oztaysi, B. (2019a). Analyzing of gender behaviors
from paths using process mining: a shopping mall ap-
plication. Sensors, 19(3):557.
Dogan, O., Martinez-Millana, A., Rojas, E., Sep
´
ulveda, M.,
Munoz-Gama, J., Traver, V., and Fernandez-Llatas, C.
(2019b). Individual behavior modeling with sensors
using process mining. Electronics, 8(7):766.
Holten, D. (2006). Hierarchical edge bundles: Visualiza-
tion of adjacency relations in hierarchical data. IEEE
Transactions on visualization and computer graphics,
12(5):741–748.
Kaplan, E. and Hegarty, C. (2005). Understanding GPS:
principles and applications. Artech house.
Krueger, R., Heimerl, F., Han, Q., Kurzhals, K., Koch, S.,
and Ertl, T. (2015). Visual analysis of visitor behavior
for indoor event management. In 2015 48th Hawaii
International Conference on System Sciences, pages
1148–1157. IEEE.
Marini, G. (2019). Towards indoor localisation analytics
for modelling flows of movements. In Adjunct Pro-
ceedings of the 2019 ACM International Joint Con-
ference on Pervasive and Ubiquitous Computing and
Proceedings of the 2019 ACM International Sympo-
sium on Wearable Computers, pages 377–382.
Martella, C., Miraglia, A., Frost, J., Cattani, M., and van
Steen, M. (2017). Visualizing, clustering, and predict-
ing the behavior of museum visitors. Pervasive and
Mobile Computing, 38:430–443.
Melamed, R. (2016). Indoor localization: Challenges and
opportunities. In Proceedings of the International
Conference on Mobile Software Engineering and Sys-
tems, pages 1–2.
Oppermann, M. and Munzner, T. (2020). Ocupado: Visu-
alizing location-based counts over time across build-
ings. In Computer Graphics Forum, volume 39, pages
127–138. Wiley Online Library.
Schneider, F. A., Branco, A., Rodrigues, A. M., Carvalho,
F., Endler, M., and Lopes, H. (2021). Yet another ble
technology based tracking system. In SAC 2021, page
771. ACM Digital Library.
Tableau (2003). Tableau software. http://www.
tableausoftware.com/, last accessed on July 2020.
Van Der Aalst, W. (2011). Process mining: discovery,
conformance and enhancement of business processes,
volume 2. Springer.
Van Rossum, G. and Drake, F. L. (2011). The python lan-
guage reference manual. Network Theory Ltd.
Visual Analysis Tool for a BLE Technology based Tracking Data
239