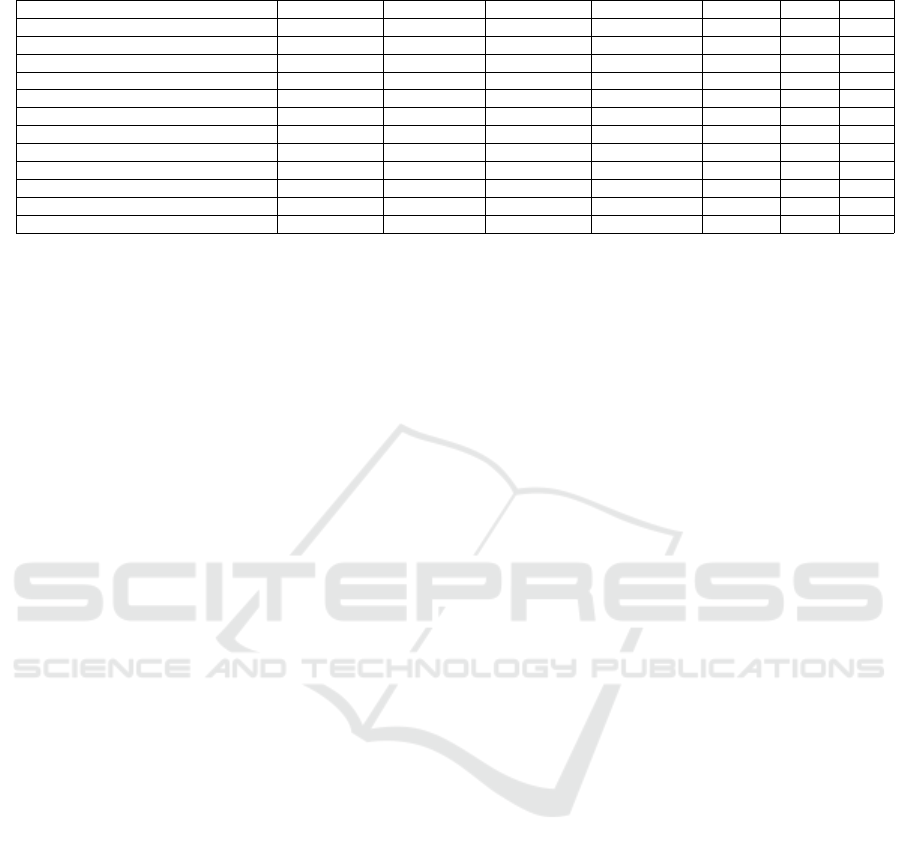
Table 4: Average Precision and Recall of Individual Objects on HHCC Point Cloud.
Name Ground Truth True Positive False Positive False Negative Precision Recall F1
building entrance-exit 14 7 54 7 0.115 0.500 0.187
door 69 69 224 0 0.235 1.000 0.381
elevator 2 2 7 0 0.222 1.000 0.364
fire alarm 64 61 31 3 0.663 0.953 0.782
fire alarm switch 14 7 41 7 0.146 0.500 0.226
fire suppression systems - extinguisher 20 19 6 1 0.760 0.950 0.844
server equipment 2 0 13 2 0.000 0.000 0.000
sign exit 37 37 38 0 0.493 1.000 0.661
smoke detector 4 0 10 4 0.000 0.000 0.000
stairway 1 1 51 0 0.019 1.000 0.038
utility shut offs - electric 49 49 14 0 0.778 1.000 0.875
utility shut offs - water 3 1 6 2 0.143 0.333 0.200
couraging despite our limited training dataset. For
the next step, we plan to improve the annotation per-
formance by addressing the following issues: (a) in-
creasing the number of annotated images for the ob-
jects that are lacking in both our dataset and public
datasets; and (b) improving the recognition accuracy
of small objects such as sprinklers and smoke detec-
tors. We also plan to apply machine learning models
directly to point clouds as a complementary process
to improve the overall accuracy and confidence.
ACKNOWLEDGMENTS
This work was performed under the financial assis-
tance award 70NANB18H247 from U.S. Department
of Commerce, National Institute of Standards and
Technology. We are thankful to the City of Memphis,
especially Cynthia Halton, Wendy Harris, Gertrude
Moeller, and Joseph R. Roberts, for assisting us in
collecting data from city buildings, testing our 3D
models, and hosting our data for public access. We
would also like to acknowledge the hard work of our
undergraduate students: Madeline Cychowski, Marg-
eret Homeyer, Abigail Jacobs, and Jonathan Wade,
who helped us scan the buildings and manually an-
notate the image data.
REFERENCES
Armeni, I., Sax, S., Zamir, A. R., and Savarese, S. (2017).
Joint 2d-3d-semantic data for indoor scene under-
standing. arXiv preprint arXiv:1702.01105.
Armeni, I., Sener, O., Zamir, A. R., Jiang, H., Brilakis, I.,
Fischer, M., and Savarese, S. (2016). 3D semantic
parsing of large-scale indoor spaces. In Proceedings
of the IEEE Conference on Computer Vision and Pat-
tern Recognition, pages 1534–1543.
CDC/National Institute for Occupational Safety and Health
(NIOSH) website (2020). Fire fighter fatality investi-
gation and prevention program. https://www.cdc.gov/
niosh/fire/default.html.
Chang, A., Funkhouser, T., Guibas, L., Hanrahan,
P., Huang, Q., Li, Z., Savarese, S., Savva, M.,
Song, S., Su, H., et al. (2015). ShapeNet: An
information-rich 3D model repository. arXiv preprint
arXiv:1512.03012.
CloudCompare v2.10.0 (2019). CloudCompare (version
2.10.0) [GPL software]. http://www.cloudcompare.
org/.
ESRI (2020). ArcGIS Pro (v2.5.0): Next-generation desk-
top GIS. https://www.esri.com/en-us/arcgis/products/
arcgis-pro/overview.
Ester, M., Kriegel, H.-P., Sander, J., Xu, X., et al. (1996).
A density-based algorithm for discovering clusters in
large spatial databases with noise. In KDD, pages
226–231.
Evarts, B. (2019). NFPA report: Fire loss in the
United States during 2018. https://www.nfpa.
org/News-and-Research/Data-research-and-tools/
US-Fire-Problem/Fire-loss-in-the-United-States.
Fahy, R. and Molis, J. (2019). NFPA report: Fire-
fighter fatalities in the US - 2018. https:
//www.nfpa.org//-/media/Files/News-and-Research/
Fire-statistics-and-reports/Emergency-responders/
osFFF.pdf.
GVI (2018). GreenValley International LiBackpack 50.
https://greenvalleyintl.com/hardware/libackpack/.
He, K., Gkioxari, G., Doll
´
ar, P., and Girshick, R. (2017).
Mask R-CNN. In Proceedings of the IEEE interna-
tional conference on computer vision, pages 2961–
2969.
He, K., Zhang, X., Ren, S., and Sun, J. (2016). Deep resid-
ual learning for image recognition. In 2016 IEEE Con-
ference on Computer Vision and Pattern Recognition
(CVPR), pages 770–778.
Hertz, M. (2018). PointCloud XR virtual reality. http://
www.rslab.se/pointcloud-xr/.
Huang, J., Rathod, V., Sun, C., Zhu, M., Korattikara, A.,
Fathi, A., Fischer, I., Wojna, Z., Song, Y., Guadar-
rama, S., et al. (2017). Speed/accuracy trade-offs for
modern convolutional object detectors. In Proceed-
ings of the IEEE conference on computer vision and
pattern recognition, pages 7310–7311.
Insta360 Pro 2 Camera (2018). Insta360 Pro 2 Camera.
https://www.insta360.com/product/insta360-pro2.
Building Indoor Point Cloud Datasets with Object Annotation for Public Safety
55