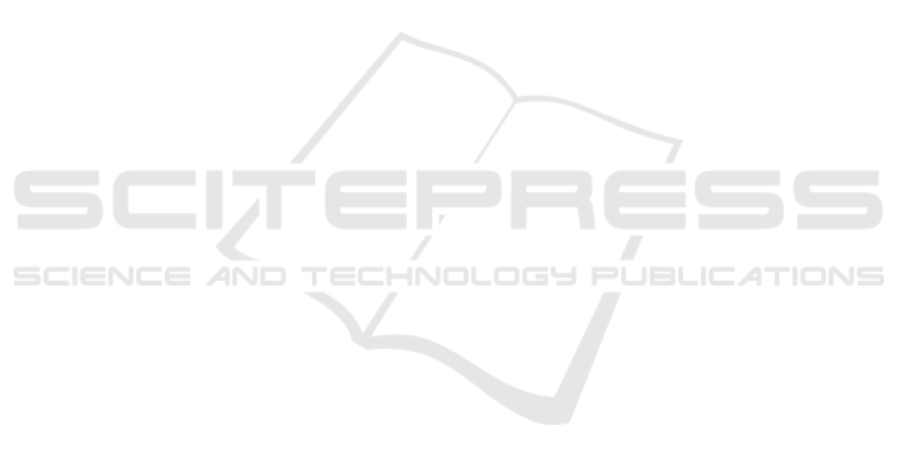
served is due to the reduction in the number of classes
analyzed from seven to two, resulting in higher accu-
racies. Even so, the results obtained by this approach
are equivalent to those from the approach with prior
knowledge.
Finally, when analyzing the results obtained, we
concluded that it was not possible to identify an in-
dividual’s personality traits from the typing rhythm
using the approaches described in this work. The ex-
clusive use of keystroke dynamics characteristics may
not provide enough information to map the personal-
ity traits of an individual.
Even knowing the limitations of a conventional
computer keyboard, as a source of information on
characteristics capable of differentiating individuals
from each other, the usage of the keyboard as an ap-
proach is encouraged by Solanki and Shukla (Solanki
and Shukla, 2014), Nahin (Nahin et al., 2014) and Ko-
lakowska (Kołakowska et al., 2013). They confirm
the benefits of using the typing rhythm from a con-
ventional computer keyboard, which is inexpensive
and already widely used in most computer systems,
in addition to being a non-intrusive approach and eas-
ily adaptable to different computer systems, including
smartphones with touchscreens.
Due to the unsatisfactory results on extracting per-
sonality traits, we believe that it is not possible to
clearly map an individual’s personality traits through
the keystroke dynamics. On the other hand, we be-
lieve in the possibility of success in the development
of studies aimed at new approaches and experiments
focused on mapping information with a greater rela-
tionship with human motor functions, such as emo-
tions and emotional state, as presented by Zimmer-
mann (Zimmermann et al., 2003). Such work can be
performed using an adaptation of the data acquisition
tool already developed, using the same data acquisi-
tion process, adapting only the self-assessment ques-
tionnaire to map emotions and emotional states.
REFERENCES
Allport, G. W. (1961). Pattern and growth in personality.
Buker, A. A., Roffo, G., and Vinciarelli, A. (2019). Type
like a man! inferring gender from keystroke dynamics
in live-chats. IEEE Intelligent Systems, 34(6):53–59.
Carver, C. S. Scheier, M. F. (2000). Personality is a dy-
namic organization, inside the person, of psychophys-
ical systems that create the person’s characteristic pat-
terns of behavior, thoughts and feelings.. (Boston).
Perspectives on personality.
Epp, C., Lippold, M., and Mandryk, R. L. (2011). Identi-
fying emotional states using keystroke dynamics. In
Proceedings of the sigchi conference on human fac-
tors in computing systems, pages 715–724.
Gosling, S. D., Rentfrow, P. J., and Swann Jr, W. B. (2003).
A very brief measure of the big-five personality do-
mains. Journal of Research in personality, 37(6):504–
528.
Khan, I. A., Brinkman, W.-P., Fine, N., and Hierons, R. M.
(2008). Measuring personality from keyboard and
mouse use. pages 1–8.
Khan, I. A., Khalid, O., Jadoon, W., Shan, R. U., and Nasir,
A. N. (2015). Predicting programmers’ personality
via interaction behaviour with keyboard and mouse.
PeerJ PrePrints, 3:e1183v1.
Khanna, P. and Sasikumar, M. (2010). Recognising emo-
tions from keyboard stroke pattern. International jour-
nal of computer applications, 11(9):1–5.
Kołakowska, A., Landowska, A., Szwoch, M., Szwoch, W.,
and Wr
´
obel, M. R. (2013). Emotion recognition and
its application in software engineering. pages 532–
539.
Likert, R. (1932). A technique for the measurement of atti-
tudes. Archives of psychology.
Montalv
˜
ao Filho, J. R. and Freire, E. O. (2006). On the
equalization of keystroke timing histograms. Pattern
Recognition Letters, 27(13):1440–1446.
Nahin, A. N. H., Alam, J. M., Mahmud, H., and Hasan, K.
(2014). Identifying emotion by keystroke dynamics
and text pattern analysis. Behaviour & Information
Technology, 33(9):987–996.
Nunes, M., Bezerra, J. S., Reinert, D., Moraes, D., Silva,
´
E. P., and Pereira, A. J. (2010). Computac¸
˜
ao afetiva
e sua influ
ˆ
encia na personalizac¸
˜
ao de ambientes edu-
cacionais: gerando equipes compat
´
ıveis para uso em
avas na ead. Educac¸
˜
ao E Ciberespac¸o: Estudos, Pro-
postas E Desafios. Aracaju: Virtus Editora, 1:308–
347.
Pervin, L. A., Cervone, D., and John, O. P. (2004). Person-
ality: Theory and Research. Wiley, 9th edition.
Plank, B. (2018). Predicting authorship and author traits
from keystroke dynamics. In Proceedings of the Sec-
ond Workshop on Computational Modeling of Peo-
ple’s Opinions, Personality, and Emotions in Social
Media, pages 98–104.
Shepherd, S. (1995). Continuous authentication by analysis
of keyboard typing characteristics.
Solanki, R. and Shukla, P. (2014). Estimation of the user’s
emotional state by keystroke dynamics. International
Journal of Computer Applications, 94(13).
Stathopoulou, I.-O., Alepis, E., Tsihrintzis, G. A., and
Virvou, M. (2010). On assisting a visual-facial af-
fect recognition system with keyboard-stroke pattern
information. Knowledge-Based Systems, 23(4):350–
356.
Zimmermann, P., Guttormsen, S., Danuser, B., and Gomez,
P. (2003). Affective computing—a rationale for mea-
suring mood with mouse and keyboard. Interna-
tional journal of occupational safety and ergonomics,
9(4):539–551.
ICEIS 2021 - 23rd International Conference on Enterprise Information Systems
482