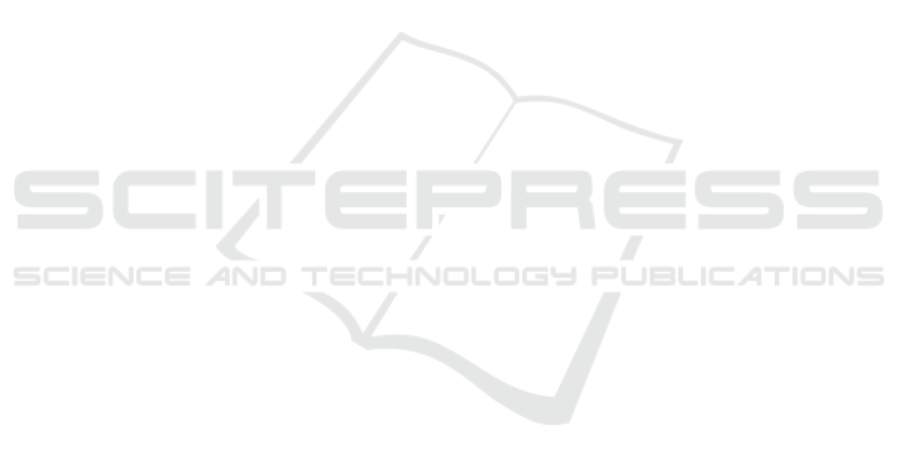
ACKNOWLEDGEMENTS
This work has been supported, in part, by Hestim,
CNRST Morocco, and the Universit
´
e du Littoral C
ˆ
ote
d’Opale, Calais France.
REFERENCES
Baldi, P., Sadowski, P., and Whiteson, D. (2014). Searching
for exotic particles in high-energy physics with deep
learning. Nature communications, 5(1):1–9.
Benkedjouh, T., Medjaher, K., Zerhouni, N., and Rechak, S.
(2015). Health assessment and life prediction of cut-
ting tools based on support vector regression. Journal
of Intelligent Manufacturing, 26(2):213–223.
Bilalli, B., Abell
´
o, A., Aluja-Banet, T., Munir, R. F.,
and Wrembel, R. (2018). Presistant: data pre-
processing assistant. In International Conference on
Advanced Information Systems Engineering, pages
57–65. Springer.
Bilalli, B., Abell
´
o, A., Aluja-Banet, T., and Wrembel, R.
(2016). Automated data pre-processing via meta-
learning. In International Conference on Model and
Data Engineering, pages 194–208. Springer.
Bohanec, M., Bor
ˇ
stnar, M. K., and Robnik-
ˇ
Sikonja, M.
(2017). Explaining machine learning models in
sales predictions. Expert Systems with Applications,
71:416–428.
Brazdil, P., Carrier, C. G., Soares, C., and Vilalta, R. (2008).
Metalearning: Applications to data mining. Springer
Science & Business Media.
Cohen-Shapira, N., Rokach, L., Shapira, B., Katz, G., and
Vainshtein, R. (2019). Autogrd: Model recommenda-
tion through graphical dataset representation. In Pro-
ceedings of the 28th ACM International Conference
on Information and Knowledge Management, pages
821–830.
Costa, C. F. and Nascimento, M. A. (2016). Ida 2016 indus-
trial challenge: Using machine learning for predicting
failures. In International Symposium on Intelligent
Data Analysis, pages 381–386. Springer.
De, T., Giri, P., Mevawala, A., Nemani, R., and Deo, A.
(2020). Explainable ai: A hybrid approach to gener-
ate human-interpretable explanation for deep learning
prediction. Procedia Computer Science, 168:40–48.
Dyrmishi, S., Elshawi, R., and Sakr, S. (2019). A deci-
sion support framework for automl systems: A meta-
learning approach. In 2019 International Conference
on Data Mining Workshops (ICDMW), pages 97–106.
IEEE.
Feurer, M., Klein, A., Eggensperger, K., Springenberg,
J. T., Blum, M., and Hutter, F. (2019). Auto-
sklearn: efficient and robust automated machine learn-
ing. In Automated Machine Learning, pages 113–134.
Springer, Cham.
Laadan, D., Vainshtein, R., Curiel, Y., Katz, G., and
Rokach, L. (2019). Rankml: a meta learning-based
approach for pre-ranking machine learning pipelines.
arXiv preprint arXiv:1911.00108.
Lemke, C., Budka, M., and Gabrys, B. (2015). Metalearn-
ing: a survey of trends and technologies. Artificial
intelligence review, 44(1):117–130.
Mazumder, R. K., Salman, A. M., and Li, Y. Failure risk
analysis of pipelines using data-driven machine learn-
ing algorithms. Structural Safety, 89:102047.
Nargesian, F., Samulowitz, H., Khurana, U., Khalil, E. B.,
and Turaga, D. S. (2017). Learning feature engineer-
ing for classification. In IJCAI, pages 2529–2535.
Samek, W. and M
¨
uller, K.-R. (2019). Towards explainable
artificial intelligence. In Explainable AI: interpreting,
explaining and visualizing deep learning, pages 5–22.
Springer.
Saravanamurugan, S., Thiyagu, S., Sakthivel, N., and Nair,
B. B. (2017). Chatter prediction in boring process us-
ing machine learning technique. International Journal
of Manufacturing Research, 12(4):405–422.
Shin, D. The effects of explainability and causability
on perception, trust, and acceptance: Implications
for explainable ai. International Journal of Human-
Computer Studies, 146:102551.
Tao, F., Qi, Q., Liu, A., and Kusiak, A. (2018). Data-driven
smart manufacturing. Journal of Manufacturing Sys-
tems, 48:157–169.
Thoben, K.-D., Wiesner, S., and Wuest, T. (2017). “indus-
trie 4.0” and smart manufacturing-a review of research
issues and application examples. International jour-
nal of automation technology, 11(1):4–16.
Tian, Y., Fu, M., and Wu, F. (2015). Steel plates fault diag-
nosis on the basis of support vector machines. Neuro-
computing, 151:296–303.
Vilalta, R., Giraud-Carrier, C. G., Brazdil, P., and Soares, C.
(2004). Using meta-learning to support data mining.
IJCSA, 1(1):31–45.
Wang, J., Ma, Y., Zhang, L., Gao, R. X., and Wu, D. (2018).
Deep learning for smart manufacturing: Methods
and applications. Journal of Manufacturing Systems,
48:144–156.
Wolf, H., Lorenz, R., Kraus, M., Feuerriegel, S., and Net-
land, T. H. (2019). Bringing advanced analytics to
manufacturing: A systematic mapping. In IFIP Inter-
national Conference on Advances in Production Man-
agement Systems, pages 333–340. Springer.
Wuest, T., Weimer, D., Irgens, C., and Thoben, K.-D.
(2016). Machine learning in manufacturing: advan-
tages, challenges, and applications. Production and
Manufacturing Research, 4(1):23–45.
Zacarias, A. G. V., Reimann, P., and Mitschang, B. (2018).
A framework to guide the selection and configuration
of machine-learning-based data analytics solutions in
manufacturing. Procedia CIRP, 72:153–158.
ICEIS 2021 - 23rd International Conference on Enterprise Information Systems
716