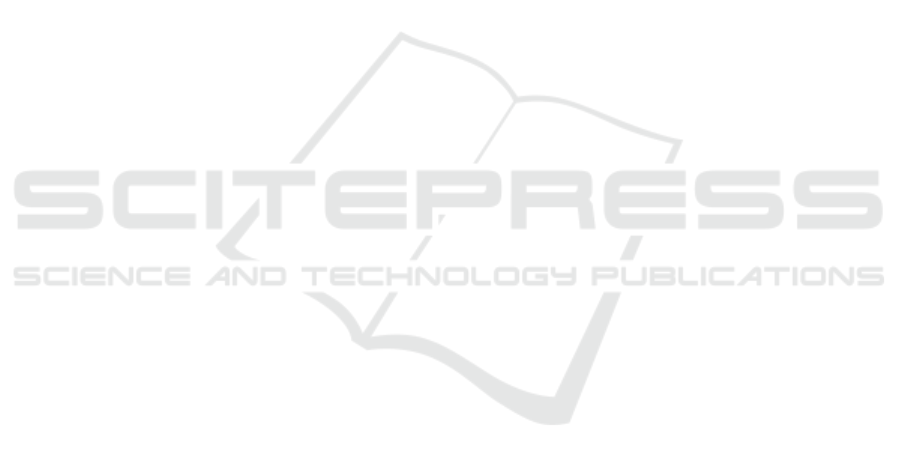
de amostras ultrafinas. Revista Engenharia de Inter-
esse Social, 1(3).
Januzzi, A. (2008). An
´
alise da aglomerac¸
˜
ao a frio no pro-
cesso hps (hybrid pelletized sinter) com
ˆ
enfase nas
mat
´
erias-primas envolvidas.
Karras, K., Pallis, E., Mastorakis, G., Nikoloudakis, Y.,
Batalla, J. M., Mavromoustakis, C. X., and Markakis,
E. (2020). A hardware acceleration platform for ai-
based inference at the edge. Circuits, Systems, and
Signal Processing, 39(2):1059–1070.
Keresztes, B., Abdelghafour, F., Randriamanga, D.,
da Costa, J.-P., and Germain, C. (2018). Real-time
fruit detection using deep neural networks. In 14th
International Conference on Precision Agriculture.
Kinnunen, P. H.-M. and Kaksonen, A. H. (2019). Towards
circular economy in mining: Opportunities and bot-
tlenecks for tailings valorization. Journal of Cleaner
Production, 228:153–160.
Klippel, E., Oliveira, R., Maslov, D., Bianchi, A., Silva,
S. E., and Garrocho, C. (2020). Towards to an embed-
ded edge ai implementation for longitudinal rip de-
tection in conveyor belt. In Anais Estendidos do X
Simp
´
osio Brasileiro de Engenharia de Sistemas Com-
putacionais, pages 97–102, Porto Alegre, RS, Brasil.
SBC.
LeCun, Y., Bengio, Y., and Hinton, G. (2015). Deep learn-
ing. nature, 521(7553):436–444.
Li, E., Zeng, L., Zhou, Z., and Chen, X. (2019). Edge
ai: On-demand accelerating deep neural network in-
ference via edge computing. IEEE Transactions on
Wireless Communications, 19(1):447–457.
Lin, X., Li, J., Wu, J., Liang, H., and Yang, W. (2019). Mak-
ing knowledge tradable in edge-ai enabled iot: A con-
sortium blockchain-based efficient and incentive ap-
proach. IEEE Transactions on Industrial Informatics,
15(12):6367–6378.
Mardonova, M. and Choi, Y. (2018). Review of wearable
device technology and its applications to the mining
industry. Energies, 11(3):547.
Mazzia, V., Khaliq, A., Salvetti, F., and Chiaberge, M.
(2020). Real-time apple detection system using em-
bedded systems with hardware accelerators: An edge
ai application. IEEE Access, 8:9102–9114.
Ohbuchi, E. (2018). Low power ai hardware platform
for deep learning in edge computing. In 2018 IEEE
CPMT Symposium Japan (ICSJ), pages 89–90. IEEE.
Passos, L. A. S., Moreira, J. L., Jorge, A., and Cavalcante,
M. V. S. (2014). Melhoria no desempenho do processo
de produc¸
˜
ao de pelotas de min
´
erio de ferro em discos
de pelotizac¸
˜
ao pela utilizac¸
˜
ao de sistemas otimizantes
com l
´
ogica nebulosa. In ABM Proceedings. Editora
Blucher.
Robben, C. and Wotruba, H. (2019). Sensor-based ore sort-
ing technology in mining—past, present and future.
Minerals, 9(9):523.
Saleem, M. H., Potgieter, J., and Arif, K. M. (2019). Plant
disease detection and classification by deep learning.
Plants, 8(11):468.
Shibuta, Y., Ohno, M., and Takaki, T. (2018). Com-
putational metallurgy: Advent of cross-scale mod-
eling: High-performance computing of solidification
and grain growth (adv. theory simul. 9/2018). Ad-
vanced Theory and Simulations, 1(9):1870020.
Sinoviev, V. V., Okolnishnikov, V. V., Starodubov, A. N.,
and Dorofeev, M. U. (2016). Approach to effective-
ness evaluation of robotics technology in mining us-
ing discrete event simulation. International Journal of
Mathematics and Computers in Simulation, 10:123–
128.
Wang, X., Han, Y., Wang, C., Zhao, Q., Chen, X., and Chen,
M. (2019). In-edge ai: Intelligentizing mobile edge
computing, caching and communication by federated
learning. IEEE Network, 33(5):156–165.
Zhang, Q., Liu, Y., Gong, C., Chen, Y., and Yu, H. (2020).
Applications of deep learning for dense scenes analy-
sis in agriculture: A review. Sensors, 20(5):1520.
Zhou, X., Fang, B., Qian, J., Xie, G., Deng, B., and Qian,
J. (2019a). Data driven faster r-cnn for transmission
line object detection. In Cyberspace Data and In-
telligence, and Cyber-Living, Syndrome, and Health,
pages 379–389. Springer.
Zhou, Z., Chen, X., Li, E., Zeng, L., Luo, K., and Zhang, J.
(2019b). Edge intelligence: Paving the last mile of ar-
tificial intelligence with edge computing. Proceedings
of the IEEE, 107(8):1738–1762.
Zobnin, N. N., Torgovets, A. K., Pikalova, I. A., Yussupova,
Y. S., and Atakishiyev, S. A. (2018). Influence of ther-
mal stability of quartz and the particle size distribution
of burden materials on the process of electrothermal
smelting of metallurgical silicon. Oriental Journal of
Chemistry, 34(2):1120–1125.
Edge Deep Learning Applied to Granulometric Analysis on Quasi-particles from the Hybrid Pelletized Sinter (HPS) Process
535