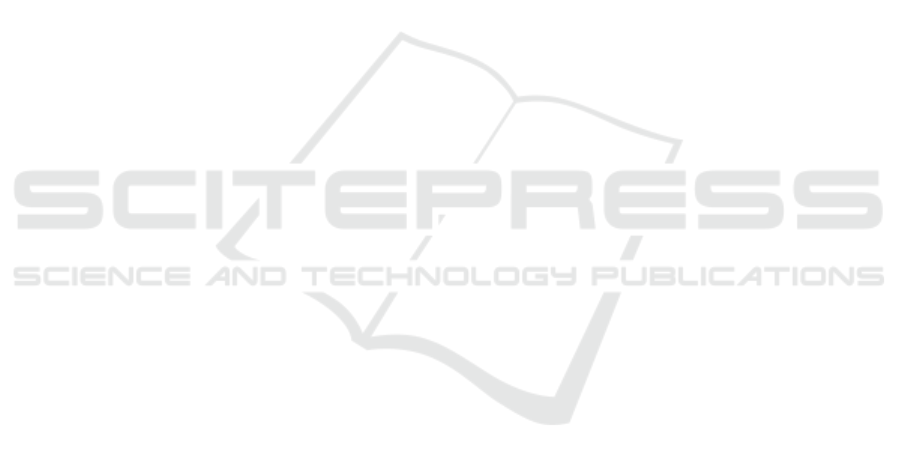
Gulati, P. (2020). Hybrid resampling technique to tackle the
imbalanced classification problem.
Hall, M., Frank, E., Holmes, G., Pfahringer, B., Reutemann,
P., and Witten, I. H. (2009). The weka data min-
ing software: an update. ACM SIGKDD explorations
newsletter, 11(1):10–18.
Hasim, N. and Haris, N. A. (2015). A study of open-source
data mining tools for forecasting. In Proceedings of
the 9th International Conference on Ubiquitous Infor-
mation Management and Communication, pages 1–4.
Hirakata, V. N., Mancuso, A. C. B., and Castro, S. M. d. J.
(2019). Teste de hip
´
oteses: perguntas que voc
ˆ
e sem-
pre quis fazer, mas nunca teve coragem. Teste de
hip
´
oteses: perguntas que voc
ˆ
e sempre quis fazer, mas
nunca teve coragem. Vol. 39, n. 2, 2019, p. 181-185.
Hubert, M. and Vandervieren, E. (2008). An adjusted box-
plot for skewed distributions. Computational statistics
& data analysis, 52(12):5186–5201.
James, G., Witten, D., Hastie, T., and Tibshirani, R. (2013).
An introduction to statistical learning, volume 112.
Springer.
Jovic, A., Brkic, K., and Bogunovic, N. (2014). An
overview of free software tools for general data min-
ing. In 2014 37th International Convention on In-
formation and Communication Technology, Electron-
ics and Microelectronics (MIPRO), pages 1112–1117.
IEEE.
Kotsiantis, S., Kanellopoulos, D., Pintelas, P., et al. (2006).
Handling imbalanced datasets: A review. GESTS In-
ternational Transactions on Computer Science and
Engineering, 30(1):25–36.
Ladha, L. and Deepa, T. (2011). Feature selection methods
and algorithms. International Journal on Computer
Science and Engineering.
Li, H., Li, J., Chang, P.-C., and Sun, J. (2013). Paramet-
ric prediction on default risk of chinese listed tourism
companies by using random oversampling, isomap,
and locally linear embeddings on imbalanced sam-
ples. International Journal of Hospitality Manage-
ment, 35:141–151.
Lilliefors, H. W. (1967). On the kolmogorov-smirnov
test for normality with mean and variance un-
known. Journal of the American statistical Associa-
tion, 62(318):399–402.
Liu, H., Shah, S., and Jiang, W. (2004). On-line outlier
detection and data cleaning. Computers & Chemical
Engineering, 28(9):1635 – 1647.
Liu, X.-Y., Wu, J., and Zhou, Z.-H. (2008). Exploratory
undersampling for class-imbalance learning. IEEE
Transactions on Systems, Man, and Cybernetics, Part
B (Cybernetics), 39(2):539–550.
Longadge, R. and Dongre, S. (2013). Class imbal-
ance problem in data mining review. arXiv preprint
arXiv:1305.1707.
Luo, W., Phung, D., Tran, T., Gupta, S., Rana, S., Kar-
makar, C., Shilton, A., Yearwood, J., Dimitrova, N.,
Ho, T. B., et al. (2016). Guidelines for developing
and reporting machine learning predictive models in
biomedical research: a multidisciplinary view. Jour-
nal of medical Internet research, 18(12):e323.
Melo, C. S., da Cruz, M. M. L., Martins, A. D. F., Matos,
T., da Silva Monteiro Filho, J. M., and de Cas-
tro Machado, J. (2019). A practical guide to sup-
port change-proneness prediction. In ICEIS (2), pages
269–276.
Olorisade, B. K., Brereton, P., and Andras, P. (2017). Re-
producibility in machine learning-based studies: An
example of text mining.
Ozdemir, S. (2016). Principles of data science. Packt Pub-
lishing Ltd.
Pearson, K. (1895). Notes on regression and inheritance in
the case of two parents proceedings of the royal soci-
ety of london, 58, 240-242.
Pedregosa, F., Varoquaux, G., Gramfort, A., Michel, V.,
Thirion, B., Grisel, O., Blondel, M., Prettenhofer, P.,
Weiss, R., Dubourg, V., et al. (2011). Scikit-learn:
Machine learning in python. the Journal of machine
Learning research, 12:2825–2830.
Provost, F. and Fawcett, T. (2013). Data science and its rela-
tionship to big data and data-driven decision making.
Big data, 1(1):51–59.
Sandve, G. K., Nekrutenko, A., Taylor, J., and Hovig, E.
(2013). Ten simple rules for reproducible computa-
tional research. PLoS Comput Biol, 9(10):e1003285.
Shapiro, S. S. and Wilk, M. B. (1965). An analysis
of variance test for normality (complete samples).
Biometrika, 52(3/4):591–611.
Smirnov, N. (1948). Table for estimating the goodness of fit
of empirical distributions. The annals of mathematical
statistics, 19(2):279–281.
Spearman, C. (1961). The proof and measurement of asso-
ciation between two things.
van Rossum, G. (1995). Python tutorial. Technical Report
CS-R9526, Centrum voor Wiskunde en Informatica
(CWI), Amsterdam.
Veerabhadrappa and Rangarajan, L. (2010). Bi-level di-
mensionality reduction methods using feature selec-
tion and feature extraction. International Journal of
Computer Applications, 4.
Venkatesh, B. and Anuradha, J. (2019). A review of feature
selection and its methods. Cybernetics and Informa-
tion Technologies, 19(1):3–26.
Yap, B. W., Abd Rani, K., Abd Rahman, H. A., Fong, S.,
Khairudin, Z., and Abdullah, N. N. (2014). An appli-
cation of oversampling, undersampling, bagging and
boosting in handling imbalanced datasets. In Pro-
ceedings of the first international conference on ad-
vanced data and information engineering (DaEng-
2013), pages 13–22. Springer.
A Practical Guide to Support Predictive Tasks in Data Science
255