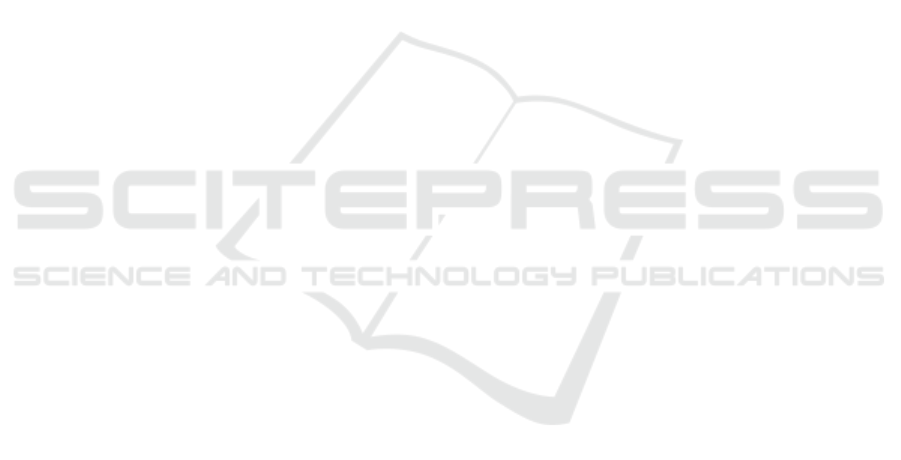
REFERENCES
Agency, U. E. P. (2012). Epa and nhtsa set standards to
reduce greenhouse gases and improve fuel economy
for model years 2017–2025 cars and light trucks.
Ahn, K. and Rakha, H. A. (2013). Network-wide impacts of
eco-routing strategies: a large-scale case study. Trans-
portation Research Part D: Transport and Environ-
ment, 25:119–130.
Centobelli, P., Cerchione, R., and Esposito, E. (2017).
Environmental sustainability in the service indus-
try of transportation and logistics service providers:
Systematic literature review and research directions.
Transportation Research Part D: Transport and Envi-
ronment, 53:454–470.
Chen, T. and Guestrin, C. (2016). Xgboost: A scalable
tree boosting system. In Proceedings of the 22nd
acm sigkdd international conf. on knowledge discov-
ery and data mining, pages 785–794.
Clewlow, R. R. and Mishra, G. S. (2017). Disruptive trans-
portation: The adoption, utilization, and impacts of
ride-hailing in the united states.
Cramer, J. and Krueger, A. B. (2016). Disruptive change
in the taxi business: The case of uber. American Eco-
nomic Review, 106(5):177–82.
DPU (2019). Massachusetts rideshare data report. Techni-
cal report, Department of Public Utilities (DPU), Mas-
sachusetts.
EPA, U. (2017). Vehicle fuel economy estimates, 1984-
2017. https://www.kaggle.com/epa/fuel-economy.
Accessed: 2020-10-02.
EPA, U. (2020). Greenhouse gas equivalences calcu-
lator. https://www.epa.gov/energy/greenhouse -gas-
equivalencies-calculator. Accessed: 2020-10-02.
Erhardt, G. D., Roy, S., Cooper, D., Sana, B., Chen, M.,
and Castiglione, J. (2019). Do transportation network
companies decrease or increase congestion? Science
advances, 5(5):eaau2670.
Feigon, S. and Murphy, C. (2016). Shared mobility and the
transformation of public transit. Number Project J-11,
Task 21.
Henao, A. and Marshall, W. E. (2019). The impact of
ride-hailing on vehicle miles traveled. Transportation,
46(6):2173–2194.
Ho, S. C., Szeto, W. Y., Kuo, Y.-H., Leung, J. M., Peter-
ing, M., and Tou, T. W. (2018). A survey of dial-a-
ride problems: Literature review and recent develop-
ments. Transportation Research Part B: Methodolog-
ical, 111:395–421.
Hochreiter, S. and Schmidhuber, J. (1997). Long short-term
memory. Neural computation, 9(8):1735–1780.
Hockstad, L. and Hanel, L. (2018). Inventory of us green-
house gas emissions and sinks. Technical report, En-
vironmental System Science Data Infrastructure for a
Virtual Ecosystem.
Hou, Y., Garikapati, V., Sperling, J., Henao, A., and Young,
S. E. (2019). A deep learning approach for tnc trip
demand prediction considering spatial-temporal fea-
tures. Technical report, National Renewable Energy
Lab.(NREL), Golden, CO (United States).
Jin, G., Cui, Y., Zeng, L., Tang, H., Feng, Y., and Huang, J.
(2020a). Urban ride-hailing demand prediction with
multiple spatio-temporal information fusion network.
Transportation Research Part C: Emerging Technolo-
gies, 117:102665.
Jin, Y., Ye, X., Ye, Q., Wang, T., Chen, J., and Yan, X.
(2020b). Demand forecasting of online car-hailing
with stacking ensemble learning approach and large-
scale datasets. IEEE Access.
Ke, J., Yang, H., Zheng, H., Chen, X., Jia, Y., Gong, P.,
and Ye, J. (2018). Hexagon-based convolutional neu-
ral network for supply-demand forecasting of ride-
sourcing services. IEEE Transactions on Intelligent
Transportation Systems, 20(11):4160–4173.
Komanduri, A., Wafa, Z., Proussaloglou, K., and Jacobs, S.
(2018). Assessing the impact of app-based ride share
systems in an urban context: Findings from austin.
Transportation Research Record, 2672(7):34–46.
Kontou, E., Garikapati, V., and Hou, Y. (2020). Reducing
ridesourcing empty vehicle travel with future travel
demand prediction. Transportation Research Part C:
Emerging Technologies, 121:102826.
RideAustin (2017). Rideaustin data (2017). http://www.
rideaustin.com/.
Rousseeuw, P. J. (1987). Silhouettes: a graphical aid to
the interpretation and validation of cluster analysis.
Journal of computational and applied mathematics,
20:53–65.
Schaller, B. (2018). The new automobility: Lyft, uber and
the future of american cities.
Wang, C., Hou, Y., and Barth, M. (2019). Data-driven
multi-step demand prediction for ride-hailing services
using convolutional neural network. In Science and
Information Conference, pages 11–22. Springer.
Wang, H. and Yang, H. (2019). Ridesourcing systems: A
framework and review. Transportation Research Part
B: Methodological, 129:122–155.
Wenzel, T., Rames, C., Kontou, E., and Henao, A. (2019).
Travel and energy implications of ridesourcing ser-
vice in austin, texas. Transportation Research Part
D: Transport and Environment, 70:18–34.
WorldWeatherOnline (2020). Historical weather api. http:
//www.worldweatheronline.com/developer/api/.
VEHITS 2021 - 7th International Conference on Vehicle Technology and Intelligent Transport Systems
200