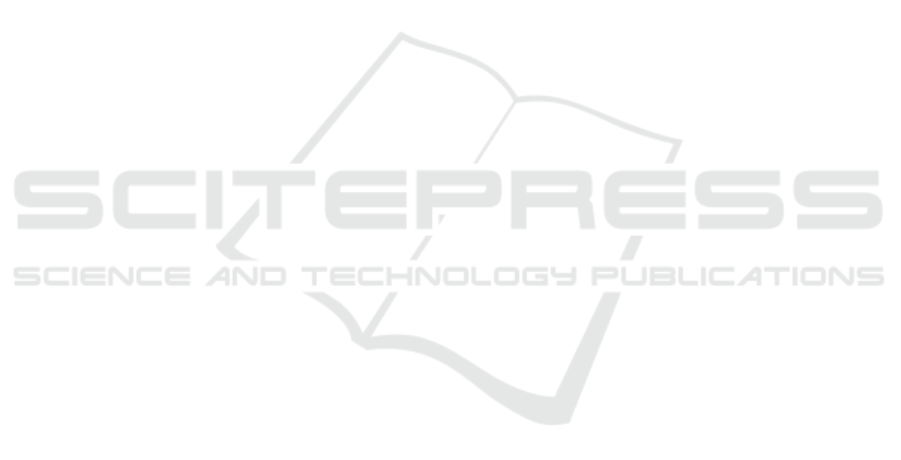
Energy, and has also been supported by by the Nat-
ural Environment Research Council; and Engineer-
ing and Physical Sciences Research Council through
the NEXUSS Centre for Doctoral Training (grant
#NE/RO12156/1).
REFERENCES
Chen, M.-H., Kira, Z., AlRegib, G., Yoo, J., Chen, R., and
Zheng, J. (2019). Temporal attentive alignment for
large-scale video domain adaptation. In Proceedings
of the IEEE/CVF International Conference on Com-
puter Vision (ICCV).
Csurka, G. (2017). A Comprehensive Survey on Do-
main Adaptation for Visual Applications, pages 1–35.
Springer International Publishing, Cham.
French, G., Mackiewicz, M., and Fisher, M. (2018). Self-
ensembling for visual domain adaptation. In Interna-
tional Conference on Learning Representations.
Ganin, Y. and Lempitsky, V. S. (2015). Unsupervised do-
main adaptation by backpropagation. In Proceed-
ings of the 32nd International Conference on Machine
Learning, ICML, pages 1180–1189.
Gorpincenko, A., French, G., and Mackiewicz, M. (2020).
Virtual adversarial training in feature space to improve
unsupervised video domain adaptation.
Haris, M., Shakhnarovich, G., and Ukita, N. (2018). Deep
back-projection networks for super-resolution. In
2018 IEEE/CVF Conference on Computer Vision and
Pattern Recognition, pages 1664–1673.
Jamal, A., Namboodiri, V. P., Deodhare, D., and Venkatesh,
K. (2018). Deep domain adaptation in action space.
In BMVC.
Karras, T., Laine, S., Aittala, M., Hellsten, J., Lehtinen, J.,
and Aila, T. (2020). Analyzing and improving the im-
age quality of stylegan.
Mao, X., Ma, Y., Yang, Z., Chen, Y., and Li, Q. (2019).
Virtual mixup training for unsupervised domain adap-
tation.
Miyato, T., Maeda, S., Koyama, M., and Ishii, S. (2019).
Virtual adversarial training: A regularization method
for supervised and semi-supervised learning. IEEE
Transactions on Pattern Analysis and Machine Intel-
ligence, 41(8):1979–1993.
Pan, S. J. and Yang, Q. (2010). A survey on transfer learn-
ing. IEEE Transactions on Knowledge and Data En-
gineering, 22(10):1345–1359.
Safdarnejad, S. M., Liu, X., Udpa, L., Andrus, B., Wood,
J., and Craven, D. (2015). Sports videos in the wild
(svw): A video dataset for sports analysis. In Proc.
International Conference on Automatic Face and Ges-
ture Recognition, Ljubljana, Slovenia.
Shimodaira, H. (2000). Improving predictive inference
under covariate shift by weighting the log-likelihood
function. Journal of Statistical Planning and Infer-
ence, 90(2):227 – 244.
Shu, R., Bui, H., Narui, H., and Ermon, S. (2018). A DIRT-
T approach to unsupervised domain adaptation. In In-
ternational Conference on Learning Representations.
Soomro, K., Zamir, A. R., and Shah, M. (2012). Ucf101:
A dataset of 101 human actions classes from videos in
the wild.
Sultani, W. and Saleemi, I. (2014). Human action recog-
nition across datasets by foreground-weighted his-
togram decomposition. In 2014 IEEE Conference on
Computer Vision and Pattern Recognition, pages 764–
771.
Sun, B. and Saenko, K. (2014). From virtual to reality: Fast
adaptation of virtual object detectors to real domains.
In Proceedings of the British Machine Vision Confer-
ence. BMVA Press.
Szegedy, C., Ioffe, S., Vanhoucke, V., and Alemi, A. A.
(2017). Inception-v4, inception-resnet and the impact
of residual connections on learning. In Proceedings of
the Thirty-First AAAI Conference on Artificial Intelli-
gence, AAAI’17, page 4278–4284. AAAI Press.
Tian, C., Xu, Y., Li, Z., Zuo, W., Fei, L., and Liu, H. (2020).
Attention-guided cnn for image denoising. Neural
Networks, 124:117 – 129.
Yun, S., Han, D., Chun, S., Oh, S. J., Yoo, Y., and
Choe, J. (2019). Cutmix: Regularization strategy to
train strong classifiers with localizable features. 2019
IEEE/CVF International Conference on Computer Vi-
sion (ICCV), pages 6022–6031.
Zhu, X. and Goldberg, A. (2009). Introduction to Semi-
Supervised Learning.
SVW-UCF Dataset for Video Domain Adaptation
111