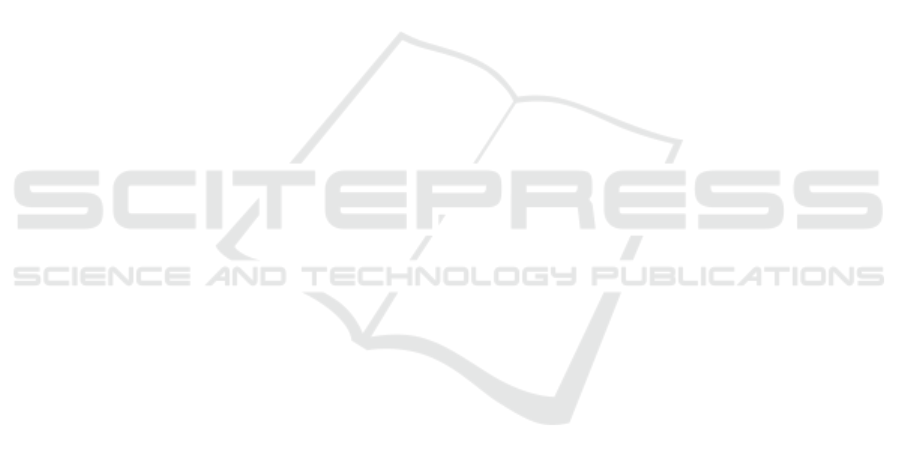
of patient through iot. In 2017 international confer-
ence on I-SMAC (IoT in social, mobile, analytics and
cloud)(I-SMAC), pages 551–556. IEEE.
Kuo, K.-M., Ma, C.-C., and Alexander, J. W. (2014). How
do patients respond to violation of their information
privacy? Health Information Management Journal,
43(2):23–33.
Lampropoulos, G., Siakas, K., and Anastasiadis, T. (2019).
Internet of things in the context of industry 4.0: An
overview. International Journal of Entrepreneurial
Knowledge, 7(1):4–19.
Li, Y. and Xia, L. (2020). Coronavirus disease 2019 (covid-
19): role of chest ct in diagnosis and management.
American Journal of Roentgenology, 214(6):1280–
1286.
Lin, Y.-J., Chuang, C.-W., Yen, C.-Y., Huang, S.-H., Huang,
P.-W., Chen, J.-Y., and Lee, S.-Y. (2019). Artificial
intelligence of things wearable system for cardiac dis-
ease detection. In 2019 IEEE International Confer-
ence on Artificial Intelligence Circuits and Systems
(AICAS), pages 67–70. IEEE.
Madhav, N., Oppenheim, B., Gallivan, M., Mulembakani,
P., Rubin, E., and Wolfe, N. (2017). Pandemics: risks,
impacts, and mitigation.
Mohammed, M., Hazairin, N. A., Al-Zubaidi, S., AK, S.,
Mustapha, S., and Yusuf, E. (2020). Toward a novel
design for coronavirus detection and diagnosis system
using iot based drone technology. International Jour-
nal of Psychosocial Rehabilitation, 24(7):2287–2295.
Nazirun, N. N. N., Shakhih, M. F. M., Ren, P. C., Zaini,
M. A. A., Nordin, N., Empaling, S., Amran, D. I., and
Supriyanto, E. (2017). User acceptance analysis of
hospital asset management system. In 2017 Interna-
tional Conference on Robotics, Automation and Sci-
ences (ICORAS), pages 1–5. IEEE.
Organization, W. H. et al. (2019). Who guideline: recom-
mendations on digital interventions for health system
strengthening: web supplement 2: summary of find-
ings and grade tables. Technical report, World Health
Organization.
Organization, W. H. et al. (2020). Covid-19 weekly epi-
demiological update, 24 november 2020.
Oshitani, H., Kamigaki, T., and Suzuki, A. (2008). Major
issues and challenges of influenza pandemic prepared-
ness in developing countries. Emerging infectious dis-
eases, 14(6):875.
Pang, Z., Yang, G., Khedri, R., and Zhang, Y.-T. (2018).
Introduction to the special section: convergence of
automation technology, biomedical engineering, and
health informatics toward the healthcare 4.0. IEEE
Reviews in Biomedical Engineering, 11:249–259.
Pardeshi, V., Sagar, S., Murmurwar, S., and Hage, P.
(2017). Health monitoring systems using iot and rasp-
berry pi—a review. In 2017 international conference
on innovative mechanisms for industry applications
(ICIMIA), pages 134–137. IEEE.
Poniszewska-Maranda, A. and Kaczmarek, D. (2015). Se-
lected methods of artificial intelligence for internet of
things conception. In 2015 Federated Conference on
Computer Science and Information Systems (FedC-
SIS), pages 1343–1348. IEEE.
Qadir, Q. M., Rashid, T. A., Al-Salihi, N. K., Ismael, B.,
Kist, A. A., and Zhang, Z. (2018). Low power wide
area networks: a survey of enabling technologies, ap-
plications and interoperability needs. IEEE Access,
6:77454–77473.
Raveendran, A. and Jayadevan, R. (2020). Reverse quaran-
tine and covid-19. Diabetes & Metabolic Syndrome:
Clinical Research & Reviews, 14(5):1323–1325.
Richardson, P., Griffin, I., Tucker, C., Smith, D., Oechsle,
O., Phelan, A., and Stebbing, J. (2020). Baricitinib
as potential treatment for 2019-ncov acute respiratory
disease. Lancet (London, England), 395(10223):e30.
Rodrigues, J. J., Segundo, D. B. D. R., Junqueira, H. A.,
Sabino, M. H., Prince, R. M., Al-Muhtadi, J., and
De Albuquerque, V. H. C. (2018). Enabling tech-
nologies for the internet of health things. Ieee Access,
6:13129–13141.
Serrano, M., Dang, H. N., and Nguyen, H. M. Q. (2018).
Recent advances on artificial intelligence and internet
of things convergence for human-centric applications:
internet of things science. In Proceedings of the 8th
International Conference on the Internet of Things,
pages 1–5.
Singh, R. P., Javaid, M., Haleem, A., and Suman, R. (2020).
Internet of things (iot) applications to fight against
covid-19 pandemic. Diabetes & Metabolic Syndrome:
Clinical Research & Reviews.
Tang, Y.-W., Schmitz, J. E., Persing, D. H., and Stratton,
C. W. (2020). Laboratory diagnosis of covid-19: cur-
rent issues and challenges. Journal of clinical micro-
biology, 58(6).
Tanne, J. H. (2020). Covid-19: Fda panel votes to approve
pfizer biontech vaccine.
Tarouco, L. M. R., Bertholdo, L. M., Granville, L. Z., Ar-
biza, L. M. R., Carbone, F., Marotta, M., and De San-
tanna, J. J. C. (2012). Internet of things in healthcare:
Interoperatibility and security issues. In 2012 IEEE
international conference on communications (ICC),
pages 6121–6125. IEEE.
Thayyil, J., Kuniyil, V., and Cherumanalil, J. M. (2020).
Covid-19: digital contact tracing technologies and
ethical challenges. International Journal of Commu-
nity Medicine and Public Health, 7(7):2854.
Thilakarathne, N. N., Kagita, M. K., Gadekallu, T. R., and
Maddikunta, P. K. R. (2020). The adoption of ict pow-
ered healthcare technologies towards managing global
pandemics. arXiv preprint arXiv:2009.05716.
Williams, P. A. and McCauley, V. (2016). Always con-
nected: The security challenges of the healthcare in-
ternet of things. In 2016 IEEE 3rd World Forum on
Internet of Things (WF-IoT), pages 30–35. IEEE.
Zhang, Y., Qiu, M., Tsai, C.-W., Hassan, M. M., and
Alamri, A. (2015). Health-cps: Healthcare cyber-
physical system assisted by cloud and big data. IEEE
Systems Journal, 11(1):88–95.
Zhu, X. (2019). Case vi: Children’s hospital of shanghai: A
pioneer in smart healthcare. In Emerging Champions
in the Digital Economy, pages 195–210. Springer.
IoTBDS 2021 - 6th International Conference on Internet of Things, Big Data and Security
236