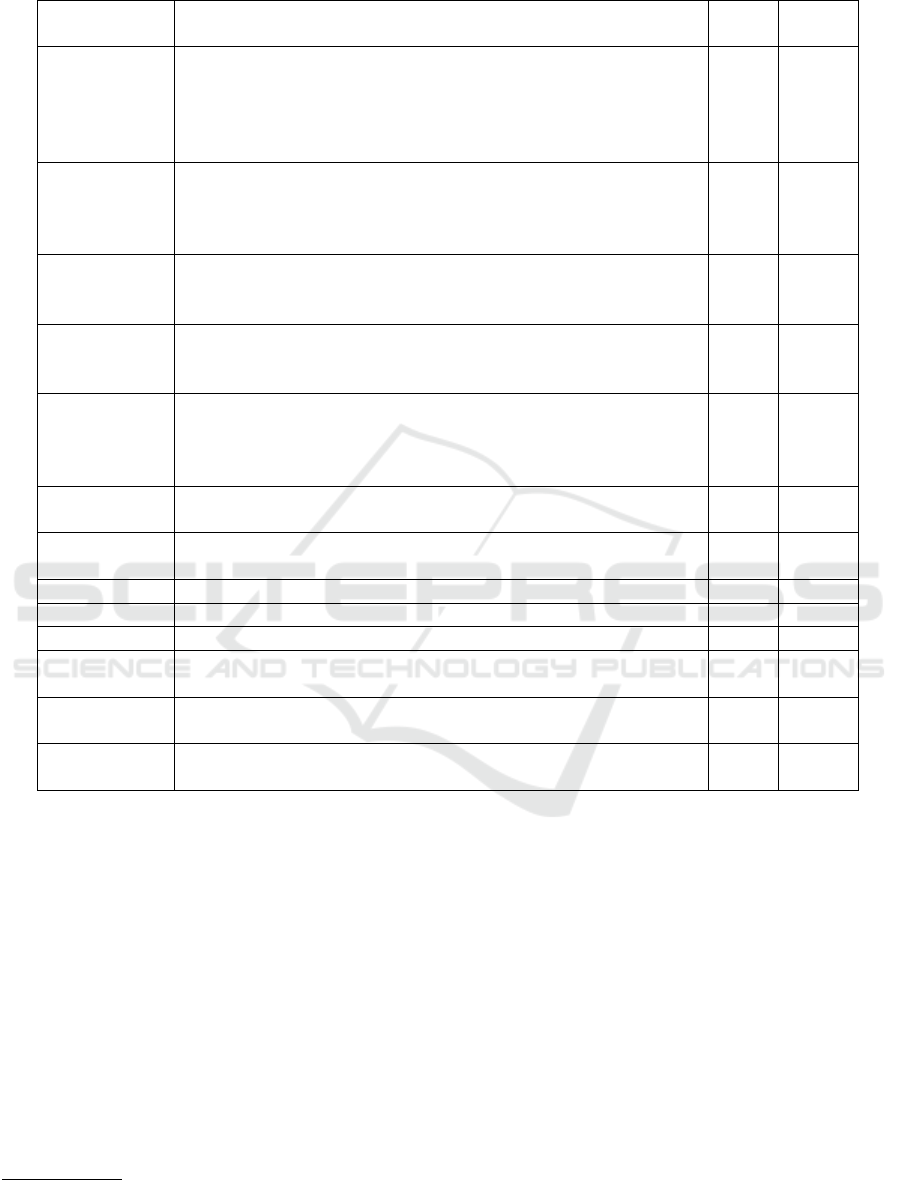
Table 5: Studies by algorithm families.
Algorithm
Family
Study ID Freq. %
Decision Tree 1, 2, 4, 5, 6, 7, 8, 9, 10, 13, 14, 16, 17, 19, 21, 23, 24, 25, 26, 27,
28, 29, 30, 31, 32, 33, 34, 35, 36, 37, 38, 39, 40, 41, 42, 43, 44,
45, 46, 48, 49, 52, 56, 57, 58, 59, 60, 62, 63, 64, 66, 68, 69, 74,
76, 77, 78, 79, 81, 83, 84, 86, 87, 88, 89, 90, 91, 92, 97, 99, 100,
101, 103, 105, 106, 108, 109, 110, 111, 112, 117, 118
82 69.49%
Ensemble 2, 3, 5, 6, 8, 11, 12, 13, 16, 17, 22, 23, 24, 29, 32, 37, 43, 46, 49,
51, 54, 55, 57, 58, 59, 61, 62, 65, 66, 67, 68, 69, 71, 72, 73, 75,
80, 82, 83, 84, 85, 87, 88, 89, 90, 91, 93, 94, 95, 96, 98, 99, 101,
102, 105, 108, 109, 112, 113, 114, 116
61 51.69%
Regression 2, 3, 6, 8, 12, 13, 15, 16, 17, 18, 19, 22, 29, 37, 44, 46, 47, 49,
51, 53, 54, 56, 64, 68, 69, 70, 83, 89, 90, 93, 94, 96, 101, 106,
107, 110, 112, 117
38 32.20%
Bayesian 2, 4, 6, 8, 12, 13, 15, 16, 21, 22, 23, 24, 26, 31, 32, 33, 34, 37,
43, 57, 58, 72, 77, 83, 84, 87, 89, 90, 91, 95, 97, 99, 100, 105,
115, 118
36 30.51%
Neural Net-
work/Deep
Neural Net-
work
2, 3, 11, 24, 29, 32, 33, 34, 37, 43, 44, 46, 50, 52, 53, 55, 56, 59,
60, 68, 69, 78, 81, 83, 84, 87, 89, 90, 101, 106, 110, 111, 112
33 27.97%
Support Vec-
tor Machine
1, 3, 12, 15, 17, 18, 22, 26, 32, 34, 37, 46, 56, 58, 59, 62, 65, 67,
68, 69, 87, 89, 90, 91, 93, 94, 95, 105, 110, 112
30 25.42%
Instance-
Based
1, 2, 6, 12, 16, 26, 32, 33, 56, 58, 59, 62, 68, 87, 89, 95, 96, 99,
101, 106, 113
21 17.80%
Rule-Based 23, 26, 30, 32, 38, 41, 43, 63, 77, 79, 84, 103, 115 13 11.01%
Clustering 14, 27, 29, 35, 45, 47, 104 7 5.93%
Association 12, 35, 45, 52, 104 5 4.24%
Discriminant
Analysis
57, 67, 106 3 2.54%
Nature-
Inspired
62 1 0.85%
Sequential
Pattern
20 1 0.85%
(33.90%, 40)
13
, f-measure (27.97%, 33), area under
curve (AUC) (25.42%, 30, including ROC curve),
true positive (20.34%, 24), true negative (16.10%,
19) false positive and sensitivity (10.17%, 12 each),
false negative (8.47%, 10), specificity (7.63%, 9),
kappa (5.93%, 7), absolute average error and geo-
metric mean (4.24%, 5 each), confidence and gini
(3.39%, 4 each), root mean square error (2.54%, 3),
relative absolute error (1.69%, 2) and support, un-
weighted average recall (UAR), permutation decrease
importance (0.85%, 1 each).
3.7.2 Analysis and Discussion
As the classification task was the one that stood out,
measures referring to the confusion matrix were fre-
13
Recall and sensitivity are the same measures. However,
we preferred to use both to keep the way it was cited.
quently used. Accuracy was the most prevalent mea-
sure. However, as mentioned in (Fern
´
andez et al.,
2018, p.47-49), accuracy is not an adequate measure
to be applied when unbalanced data are used, which
usually occurs when working in the dropout domain.
Thus, the results do not necessarily express clearly the
validity of the obtained model, as it can correctly pre-
dict the examples of the majority class (dropout) and
incorrectly those of the minority class (non-dropout).
However, it is important to mention that 74 (77.08%)
of the 96 studies that used accuracy also applied other
assessment measures. Finally, it was observed that,
as in the algorithm families, the reason for the choice
was not mentioned.
A Systematic Mapping on the Use of Data Mining for the Face-to-Face School Dropout Problem
45