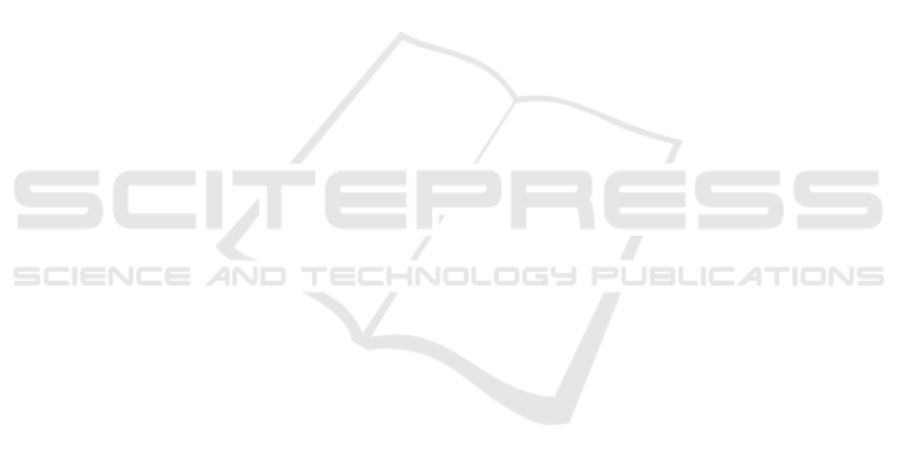
5.2 Future Work
Following on this work, the prototypical implemen-
tation will be enhanced, so that it not only supports
the requirements of the Coscine platform, but can be
used in general. With work looking into different
change indicators and implementing them, it will be
seen how well they perform and which produce the
best results in performance, accuracy and applicabil-
ity. Even new developed methods, which could focus
on machine learning and fueling a change indicator
based on a trained model, could be interesting in this
evaluation. Furthermore, the paper is focused on data
entities however an interesting area to look into is how
to reflect this research on collection of data entities
and collection of collections. Since a change indicator
is proposed, another area of future interest is to see if
the difference between data entities in two collections
could tell the similarity between them. Especially
by looking into the descriptive metadata as well, the
defining topics which are similar could be detected by
such a method and most importantly which topics are
not the same.
REFERENCES
Ametepe, W., Wang, C., Ocansey, S., Li, X., and Hussain,
F. (2018). Data provenance collection and security
in a distributed environment: a survey. International
Journal of Computers and Applications, pages 1–15.
Belhajjame, K., Cheney, J., Corsar, D., Garijo, D., Soiland-
Reyes, S., Zednik, S., and Zhao, J. (2012). Prov-o:
The prov ontology.
Bensberg, S. (2020). An efficient semantic search en-
gine for research data in an RDF-based knowledge
graph. Masterarbeit, RWTH Aachen University,
Aachen. Ver
¨
offentlicht auf dem Publikationsserver
der RWTH Aachen University; Masterarbeit, RWTH
Aachen University, 2020.
Brickley, D. and Miller, L. (2014). Foaf vocabulary specifi-
cation. http://xmlns.com/foaf/spec/.
Cruz, S., Campos, M., and Mattoso, M. (2009). Towards a
taxonomy of provenance in scientific workflow man-
agement systems. SERVICES 2009 - 5th 2009 World
Congress on Services.
Cyganiak, R., Lanthaler, M., and Wood, D. (2014). RDF
1.1 concepts and abstract syntax. W3C recommenda-
tion, W3C. http://www.w3.org/TR/2014/REC-rdf11-
concepts-20140225/.
Davidson, S., Cohen-Boulakia, S., Eyal, A., Lud
¨
ascher, B.,
McPhillips, T., Bowers, S., Anand, M., and Freire, J.
(2007). Provenance in scientific workflow systems.
IEEE Data Eng. Bull., 30:44–50.
Davidson, S. and Freire, J. (2008). Provenance and scien-
tific workflows: Challenges and opportunities. pages
1345–1350.
E. Stephan, B. Raju, T. Elsethagen, L. Pouchard, and C.
Gamboa (2017). A scientific data provenance har-
vester for distributed applications. In 2017 New York
Scientific Data Summit (NYSDS), pages 1–9.
European Organization For Nuclear Research and Ope-
nAIRE (2013). Zenodo.
Foster, E. D. and Deardorff, A. (2017). Open science frame-
work (osf). Journal of the Medical Library Associa-
tion : JMLA, 105(2):203–206.
Guha, R. V., Brickley, D., and Macbeth, S. (2016).
Schema.org: Evolution of structured data on the web.
Commun. ACM, 59(2):44–51.
Heinrichs, B. and Politze, M. (2020). Moving towards a
general metadata extraction solution for research data
with state-of-the-art methods.
Herschel, M., Diestelk
¨
amper, R., and Ben Lahmar, H.
(2017). A survey on provenance: What for? what
form? what from? The VLDB Journal, 26.
Hu, R., Yan, Z., Ding, W., and Yang, L. T. (2020). A
survey on data provenance in iot. World Wide Web,
23(2):1441–1463.
Interlandi, M., Ekmekji, A., Shah, K., Gulzar, M. A., Tetali,
S. D., Kim, M., Millstein, T., and Condie, T. (2018).
Adding data provenance support to apache spark. The
VLDB Journal, 27(5):595–615.
Mufti, Z. and Elkhodr, M. (2018). Data Provenance in the
Internet of Things: Views and Challenges.
P
´
erez, B., Rubio, J., and S
´
aenz-Ad
´
an, C. (2018). A system-
atic review of provenance systems. Knowledge and
Information Systems, 57(3):495–543.
Politze, M., Claus, F., Brenger, B. D., Yazdi, M. A., Hein-
richs, B., and Schwarz, A. (2020). How to manage
it resources in research projects? towards a collab-
orative scientific integration environment. European
journal of higher education IT, 1(2020/1):5.
Schmitz, D. and Politze, M. (2018). Forschungsdaten man-
agen – bausteine f
¨
ur eine dezentrale, forschungsnahe
unterst
¨
utzung. o-bib. Das offene Bibliotheksjournal /
Herausgeber VDB, 5(3):76–91.
Schwardmann, U. (2015). epic persistent identifiers for ere-
search. In Presentation at the joint DataCite-ePIC
workshop Persistent Identifiers: Enabling Services for
Data Intensive Research, Paris, volume 21.
Smith, W., Moyer, T., and Munson, C. (2018). Curator:
Provenance management for modern distributed sys-
tems. In Proceedings of the 10th USENIX Confer-
ence on Theory and Practice of Provenance, TaPP’18,
page 5, USA. USENIX Association.
Talia, D., Thramboulidis, K., Lai, B. C., and Cao, J. (2013).
Workflow systems for science: Concepts and tools.
ISRN Software Engineering, 2013:404525.
Wilkinson, M. D., Dumontier, M., Aalbersberg, I. J. J.,
Appleton, G., Axton, M., Baak, A., Blomberg, N.,
Boiten, J.-W., da Silva Santos, L. B., Bourne, P. E.,
Bouwman, J., Brookes, A. J., Clark, T., Crosas, M.,
Dillo, I., Dumon, O., Edmunds, S., and Evelo, Chris
T. ... Mons, B. (2016). The fair guiding principles for
scientific data management and stewardship. Scientific
data, 3:160018.
Asynchronous Data Provenance for Research Data in a Distributed System
367