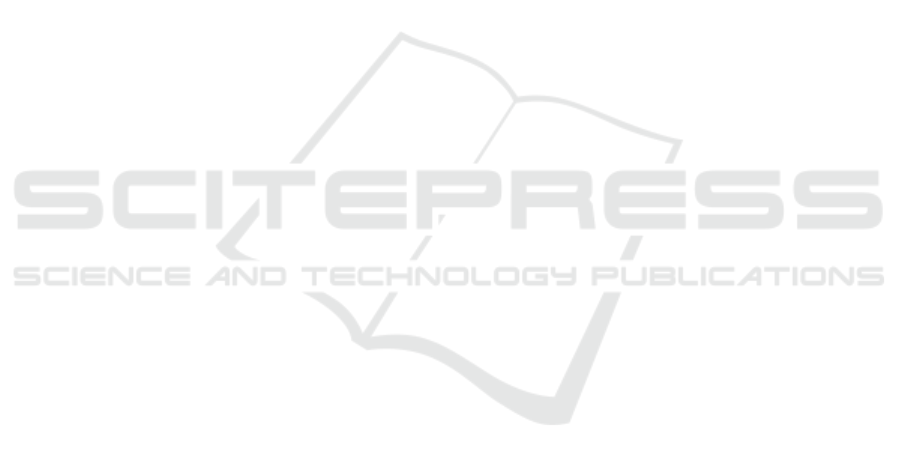
Moreover, we will take a deeper look at the
optimization problem itself and especially the
potential of multi-objective optimisation in the
context of RL and MPC, since for complex energy
systems, different and more conflicting goals will
arise.
REFERENCES
Bundesministerium für Wirtschaft und Energie (BMWi).
(2019). Energieeffizienz in Zahlen.
Renaldi, R., Kiprakis, A., Friedrich, D., 2017. An
optimisation framework for thermal energy storage
integration in a residential heat pump heating system.
In Applied Energy 186, 520–529.
Oldewurtel, F., Parisio, A., Colin, N. J., et al., 2012. Use of
model predictive control and weather forecasts for
energy efficient building climate control. In Energy and
Buildings 45, 15–27.
Chen, Y., Norford, L. K., Samuelson, H. W., et al., 2018.
Optimal control of HVAC and window systems for
natural ventilation through reinforcement learning. In
Energy and Buildings 169, 195 – 205.
Mason, K., Grijalva, S., 2019. A Review of Reinforcement
Learning for Autonomous Building Energy
Management. In Computers & Electrical Engineering
78, 300-312.
Palensky, P., Dietrich, D., 2011. Demand Side
Management: Demand Response, Intelligent Energy
Systems, and Smart Loads. In: IEEE Trans. Ind. Inf. 7
(3), S. 381–388.
Paschke, F., Franke, M., Haufe, J., 2016. Modell-prädiktive
Einzelraumregelung auf Basis empirischer Modelle, In
Central European Symposium on Building Physics
2016 / Bausim 2016.
Paschke, F., 2020. PEM-Identification of a block-oriented
nonlinear stochastic model with application to room
temperature modeling, In 28th Mediterranean
Conference on Control and Automation (MED2020),
2020, 351-356.
Blochwitz, T., Otter, M., Arnold, M., Bausch, C., Clauss,
C., Elmqvist, H., et al., 2011. The Functional Mockup
Interface for Tool independent Exchange of Simulation
Models. In Proceedings 8th Modelica Conf., 105-114.
Dassault Systems, 2017. Fmpy. URL: https://git
hub.com/CATIA-Systems/FMPy, last access 2.5.2020.
Mynttinen, I., Hoffmann, A., Runge, E., Li, P., 2015.
Smoothing and regularization strategies for
optimization of hybrid dynamic systems. In: Optim Eng
16 (3), S. 541–569.
Wigstrom, O., Lennartson, B., 2012. Scheduling model for
systems with complex alternative behaviour. In 2012
IEEE International Conference on Automation Science
and Engineering (CASE), 587–593.
Wigström, O., Lennartson, B., 2014. An Integrated CP/OR
Method for Optimal Control of Modular Hybrid
Systems. In: IFAC Proceedings Volumes 47 (2), S.
485–491. DOI: 10.3182/20140514-3-FR-4046.00130.
Schlüter, M., Egea, J. A., Bangam J. R., 2009: Extended
ant colony optimization for non-convex mixed integer
nonlinear programming. In: Computers & Operations
Research 36 (7), S. 2217–2229
Jordan, M. I., & Mitchell, T. M., 2015. Machine learning:
Trends, perspectives, and prospects. In Science,
349(6245), 255-260.
Sutton, R. S., & Barto, A. G., 2018. Reinforcement
learning: An introduction. MIT press.
Gao, G., Li, J., & Wen, Y., 2019. Energy-efficient thermal
comfort control in smart buildings via deep
reinforcement learning. In arXiv preprint
arXiv:1901.04693.
Szepesvári, C., 2010. Algorithms for reinforcement
learning. In Synthesis lectures on artificial intelligence
and machine learning, 4(1), 1-103.
Mason, K., & Grijalva, S., 2019. A review of reinforcement
learning for autonomous building energy management.
In Computers & Electrical Engineering, 78, 300-312.
Seidel, S., Huang, C., Mayer, D., et al., 2020,
Kostenoptimale Steuerung eines multivaltenten
Gebäudeenergiesystems mittels modellprädiktivem
Ansatz und Reinforcement Learning, In AUTMATION
2020 – 21. Leitkongress der Mess- und Automa-
tisierungstechnik, VDI-Berichte 2375, 43-56.
Seidel, S., Clauß, C., Majetta, K., et al., 2015, Modelica
based design and optimisation of control systems for
solar heat systems and low energy buildings, In 11th
International Modelica Conference 2015. Proceedings :
Versailles, France, September 21-23, 2015, 401-410.
SMARTGREENS 2021 - 10th International Conference on Smart Cities and Green ICT Systems
66