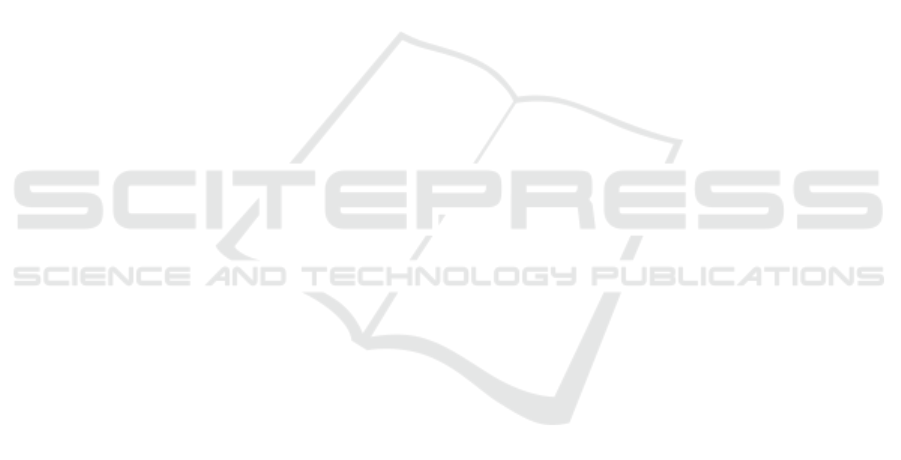
on computer vision and pattern recognition, pages
1125–1134.
Jain, S. D., Xiong, B., and Grauman, K. (2017). Pixel ob-
jectness. arXiv preprint arXiv:1701.05349.
Kendall, A., Badrinarayanan, V., and Cipolla, R. (2015).
Bayesian segnet: Model uncertainty in deep convolu-
tional encoder-decoder architectures for scene under-
standing. arXiv preprint arXiv:1511.02680.
Kendall, A. and Gal, Y. (2017). What uncertainties do
we need in bayesian deep learning for computer vi-
sion? In Advances in neural information processing
systems, pages 5574–5584.
Kuhn, C., Hofbauer, M., Petrovic, G., and Steinbach, E.
(2020). Introspective black box failure prediction for
autonomous driving. In 31st IEEE Intelligent Vehicles
Symposium.
Lee, K., Lee, H., Lee, K., and Shin, J. (2017).
Training confidence-calibrated classifiers for detect-
ing out-of-distribution samples. arXiv preprint
arXiv:1711.09325.
Li, C., Song, D., Tong, R., and Tang, M. (2018). Multispec-
tral pedestrian detection via simultaneous detection
and segmentation. arXiv preprint arXiv:1808.04818.
Liang, S., Li, Y., and Srikant, R. (2017). Enhancing the reli-
ability of out-of-distribution image detection in neural
networks. arXiv preprint arXiv:1706.02690.
Lin, T.-Y., Goyal, P., Girshick, R., He, K., and Doll
´
ar, P.
(2017). Focal loss for dense object detection. In
Proceedings of the IEEE international conference on
computer vision, pages 2980–2988.
Lin, T.-Y., Maire, M., Belongie, S., Hays, J., Perona, P.,
Ramanan, D., Doll
´
ar, P., and Zitnick, C. L. (2014).
Microsoft coco: Common objects in context. In Euro-
pean conference on computer vision, pages 740–755.
Springer.
Liu, W., Anguelov, D., Erhan, D., Szegedy, C., Reed, S.,
Fu, C.-Y., and Berg, A. C. (2016). Ssd: Single shot
multibox detector. In European conference on com-
puter vision, pages 21–37. Springer.
Rahman, Q. M., S
¨
underhauf, N., and Dayoub, F. (2019).
Did you miss the sign? a false negative alarm system
for traffic sign detectors. In 2019 IEEE/RSJ Interna-
tional Conference on Intelligent Robots and Systems
(IROS), pages 3748–3753.
Ramanagopal, M. S., Anderson, C., Vasudevan, R., and
Johnson-Roberson, M. (2018). Failing to learn: au-
tonomously identifying perception failures for self-
driving cars. IEEE Robotics and Automation Letters,
3(4):3860–3867.
Reda, F., Pottorff, R., Barker, J., and Catanzaro, B. (2017).
flownet2-pytorch: Pytorch implementation of flownet
2.0: Evolution of optical flow estimation with deep
networks.
Redmon, J., Divvala, S., Girshick, R., and Farhadi, A.
(2016). You only look once: Unified, real-time object
detection. In Proceedings of the IEEE conference on
computer vision and pattern recognition, pages 779–
788.
Redmon, J. and Farhadi, A. (2017). Yolo9000: better, faster,
stronger. In Proceedings of the IEEE conference on
computer vision and pattern recognition, pages 7263–
7271.
Redmon, J. and Farhadi, A. (2018). Yolov3: An incremental
improvement. arXiv preprint arXiv:1804.02767.
Ren, S., He, K., Girshick, R., and Sun, J. (2015). Faster
r-cnn: Towards real-time object detection with region
proposal networks. In Advances in neural information
processing systems, pages 91–99.
Ronneberger, O., Fischer, P., and Brox, T. (2015). U-net:
Convolutional networks for biomedical image seg-
mentation. In International Conference on Medical
image computing and computer-assisted intervention,
pages 234–241. Springer.
Sakaridis, C., Dai, D., and Gool, L. V. (2019). Guided cur-
riculum model adaptation and uncertainty-aware eval-
uation for semantic nighttime image segmentation. In
Proceedings of the IEEE International Conference on
Computer Vision, pages 7374–7383.
Vertens, J., Z
¨
urn, J., and Burgard, W. (2020). Heat-
net: Bridging the day-night domain gap in seman-
tic segmentation with thermal images. arXiv preprint
arXiv:2003.04645.
Yu, F., Xian, W., Chen, Y., Liu, F., Liao, M., Madhavan, V.,
and Darrell, T. (2018). Bdd100k: A diverse driving
video database with scalable annotation tooling. arXiv
preprint arXiv:1805.04687.
Zhang, L., Zhu, X., Chen, X., Yang, X., Lei, Z., and Liu, Z.
(2019). Weakly aligned cross-modal learning for mul-
tispectral pedestrian detection. In Proceedings of the
IEEE International Conference on Computer Vision,
pages 5127–5137.
Zhang, P., Wang, J., Farhadi, A., Hebert, M., and Parikh, D.
(2014). Predicting failures of vision systems. In Pro-
ceedings of the IEEE Conference on Computer Vision
and Pattern Recognition, pages 3566–3573.
Zhu, J.-Y., Park, T., Isola, P., and Efros, A. A. (2017).
Unpaired image-to-image translation using cycle-
consistent adversarial networks. In Proceedings of
the IEEE international conference on computer vi-
sion, pages 2223–2232.
VEHITS 2021 - 7th International Conference on Vehicle Technology and Intelligent Transport Systems
210