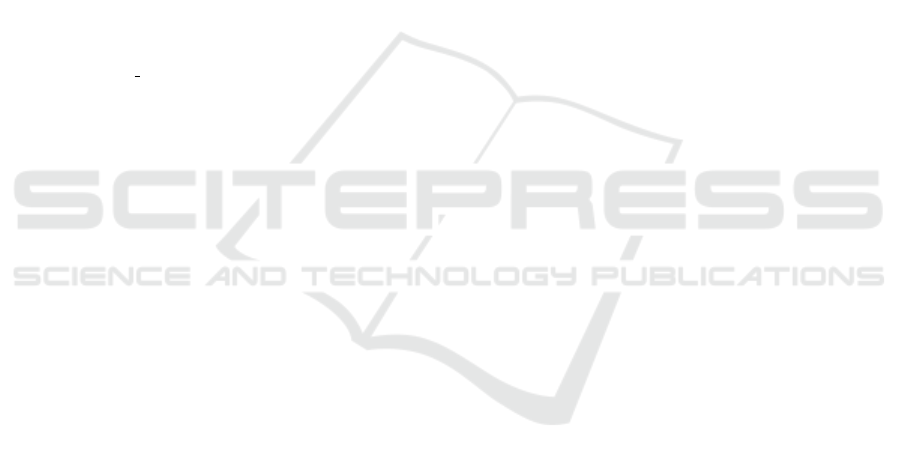
Grubwinkler, S., Hirschvogel, M., and Lienkamp, M.
(2014). Driver- and situation-specific impact factors
for the energy prediction of EVs based on crowd-
sourced speed profiles. IEEE Intelligent Vehicles Sym-
posium, Proceedings, (Iv):1069–1076.
Grubwinkler, S., Kugler, M., and Lienkamp, M. (2013). A
system for cloud-based deviation prediction of propul-
sion energy consumption for EVs. Proceedings of
2013 IEEE International Conference on Vehicular
Electronics and Safety, ICVES 2013, pages 99–104.
Guo, Q., Wu, W., Massart, D. L., Boucon, C., and De Jong,
S. (2002). Feature selection in principal component
analysis of analytical data. Chemometrics and Intelli-
gent Laboratory Systems, 61(1-2):123–132.
Guyon, I. and Elisseeff, A. (2006). Feature Extraction,
Foundations and Applications: An introduction to fea-
ture extraction. Studies in Fuzziness and Soft Comput-
ing, 207:1–25.
Guyon, I., Gunn, S., Nikravesh, M., and Zadeh, L.
(2006). Feature Extraction Foundations and Applica-
tions. pages 1–8.
HERE (2021). Guide - HERE Routing API - HERE De-
veloper. https://developer.here.com/documentation/
routing/dev{ }guide/topics/resource-type-route-link.
html [Online; accessed 17. Jan. 2021].
Huang, X., Tan, Y., and He, X. (2011). An intelligent mul-
tifeature statistical approach for the discrimination of
driving conditions of a hybrid electric vehicle. IEEE
Transactions on Intelligent Transportation Systems,
12(2):453–465.
Iora, P. and Tribioli, L. (2019). Effect of ambient tem-
perature on electric vehicles’ energy consumption and
range: Model definition and sensitivity analysis based
on Nissan Leaf data. World Electric Vehicle Journal,
10(1):1–16.
Jasinski, M. G. and Baldo, F. (2017). A Method to Iden-
tify Aggressive Driver Behaviour Based on Enriched
GPS Data Analysis. GEOProcessing 2017 : The
Ninth International Conference on Advanced Geo-
graphic Information Systems, Applications, and Ser-
vices, (March 2017):97–102.
Kamble, S. H., Mathew, T. V., and Sharma, G. K. (2009).
Development of real-world driving cycle: Case study
of Pune, India. Transportation Research Part D:
Transport and Environment, 14(2):132–140.
Kawano, H. (1997). Knowledge Discovery and Data Min-
ing. Journal of Japan Society for Fuzzy Theory and
Systems, 9(6):851–860.
Khalid, S., Khalil, T., and Nasreen, S. (2014). A survey of
feature selection and feature extraction techniques in
machine learning. Proceedings of 2014 Science and
Information Conference, SAI 2014, pages 372–378.
Knowles, M., Scott, H., and Baglee, D. (2012). The effect
of driving style on electric vehicle performance, econ-
omy and perception. International Journal of Electric
and Hybrid Vehicles, 4(3):228–247.
Kodjak, D. (2015). Policies To Reduce Fuel Consumption,
Air Pollution, and Carbon Emissions From Vehicles
in G20 Nations. The International Council on Clean
Transportation - ICCT, (May):22.
Lal, T. N., Chapelle, O., and Weston, J. (2006). Chapter 5
Embedded Methods. 165:137–165.
Langner, J., Grolig, H., Otten, S., Holz
¨
apfel, M., and Sax,
E. (2019). Logical scenario derivation by clustering
dynamic-length-segments extracted from real-world-
driving-data. VEHITS 2019 - Proceedings of the 5th
International Conference on Vehicle Technology and
Intelligent Transport Systems, pages 458–467.
Larsson, H. and Ericsson, E. (2009). The effects of an ac-
celeration advisory tool in vehicles for reduced fuel
consumption and emissions. Transportation Research
Part D: Transport and Environment, 14(2):141–146.
Le Thi, H. A., Nguyen, V. V., and Ouchani, S. (2008). Gene
selection for cancer classification using DCA. Lecture
Notes in Computer Science (including subseries Lec-
ture Notes in Artificial Intelligence and Lecture Notes
in Bioinformatics), 5139 LNAI:62–72.
Li, W., Stanula, P., Egede, P., Kara, S., and Herrmann, C.
(2016). Determining the Main Factors Influencing the
Energy Consumption of Electric Vehicles in the Usage
Phase. Procedia CIRP, 48:352–357.
Li, W., Wu, G., Zhang, Y., and Barth, M. J. (2017). A
comparative study on data segregation for mesoscopic
energy modeling. Transportation Research Part D:
Transport and Environment, 50:70–82.
Liu, K., Yamamoto, T., and Morikawa, T. (2017). Impact of
road gradient on energy consumption of electric vehi-
cles. Transportation Research Part D: Transport and
Environment, 54:74–81.
Loulizi, A., Bichiou, Y., and Rakha, H. (2019). Steady-
State Car-Following Time Gaps: An Empirical Study
Using Naturalistic Driving Data. Journal of Advanced
Transportation, 2019.
Mahmoudzadeh Andwari, A., Pesiridis, A., Rajoo, S.,
Martinez-Botas, R., and Esfahanian, V. (2017). A re-
view of Battery Electric Vehicle technology and readi-
ness levels. Renewable and Sustainable Energy Re-
views, 78(May):414–430.
Marcano-Cede
˜
no, A., Quintanilla-Dom
´
ınguez, J., Cortina-
Januchs, M. G., and Andina, D. (2010). Feature selec-
tion using Sequential Forward Selection and classifi-
cation applying Artificial Metaplasticity Neural Net-
work. IECON Proceedings (Industrial Electronics
Conference), (May 2016):2845–2850.
Marina Martinez, C., Heucke, M., Wang, F. Y., Gao, B.,
and Cao, D. (2018). Driving Style Recognition for
Intelligent Vehicle Control and Advanced Driver As-
sistance: A Survey. IEEE Transactions on Intelligent
Transportation Systems, 19(3):666–676.
Murphey, Y. L., Milton, R., and Kiliaris, L. (2009). Driver’s
style classification using jerk analysis. 2009 IEEE
Workshop on Computational Intelligence in Vehicles
and Vehicular Systems, CIVVS 2009 - Proceedings,
pages 23–28.
Prabhu, P. (2011). Improving the Performance of K-
Means Clustering For High Dimensional Data Set.
3(6):2317–2322.
Pysolar (2021). Pysolar: staring directly at the sun since
2007.
Feature-based Analysis of the Energy Consumption of Battery Electric Vehicles
233