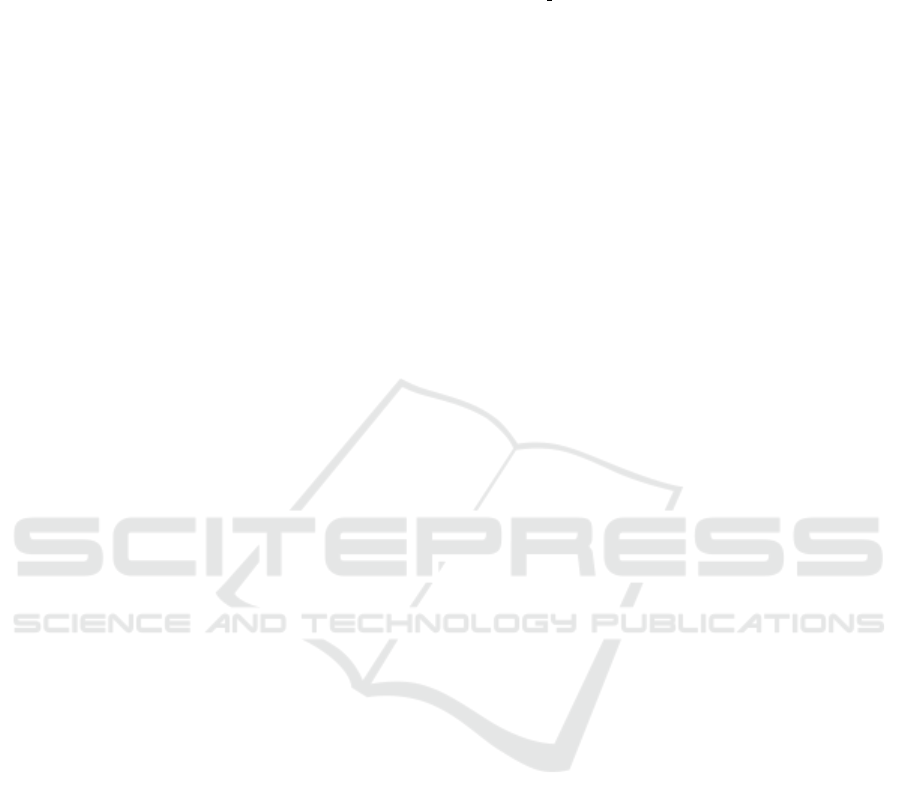
REFERENCES
Abbott, D. (2014). Applied predictive analytics: principles
and techniques for the professional data analyst. Wi-
ley, Indianapolis (IN).
Addo-Tenkorang, R. and Helo, P. T. (2016). Big data ap-
plications in operations/supply-chain management: A
literature review. Computers & Industrial Engineer-
ing, 101:528 – 543.
Ahlemeyer-Stubbe, A. and Coleman, S. (2014). A practical
guide to data mining for business and industry. John
Wiley & Sons.
Arias, M. B. and Bae, S. (2016). Electric vehicle charging
demand forecasting model based on big data technolo-
gies. Applied energy, 183:327–339.
Balliu, A., Olivetti, D., Babaoglu,
¨
O., Marzolla, M., and
S
ˆ
ırbu, A. (2016). A big data analyzer for large trace
logs. Computing, 98(12):1225–1249.
Bari, A., Chaouchi, M., and Jung, T. (2014). Predictive
Analytics for Dummies. Wiley, [s.l].
Cady, F. (2017). The data science handbook. John Wiley &
Sons, Inc., Hoboken, NJ.
Capalbo, S. M., Antle, J. M., and Seavert, C. (2017). Next
generation data systems and knowledge products to
support agricultural producers and science-based pol-
icy decision making. Agricultural systems, 155:191–
199.
Corea, F. (2016). Big data analytics: a management per-
spective, volume 21. Springer, [s.l].
Fisher, J. B., Melton, F., Middleton, E., Hain, C., Ander-
son, M., Allen, R., McCabe, M. F., Hook, S., Baldoc-
chi, D., Townsend, P. A., et al. (2017). The future of
evapotranspiration: Global requirements for ecosys-
tem functioning, carbon and climate feedbacks, agri-
cultural management, and water resources. Water Re-
sources Research, 53(4):2618–2626.
Forte, R. M. (2015). Mastering predictive analytics with R:
master the craft of predictive modeling by developing
strategy, intuition, and a solid foundation in essential
concepts. Packt Publishing, Birmingham, UK.
Hurwitz, J., Kaufman, M., and Bowles, A. (2015). Cogni-
tive Computing and Big Data Analytics. Wiley Pub-
lishing, [s.l], 1st edition.
Imenda, S. (2014). Is there a conceptual difference between
theoretical and conceptual frameworks? Journal of
Social Sciences, 38(2):185–195.
Jabareen, Y. (2009). Building a conceptual framework:
philosophy, definitions, and procedure. International
journal of qualitative methods, 8(4):49–62.
Lei, Y., Jia, F., Lin, J., Xing, S., and Ding, S. X. (2016). An
intelligent fault diagnosis method using unsupervised
feature learning towards mechanical big data. IEEE
Transactions on Industrial Electronics, 63(5):3137–
3147.
Lu, H. and Li, Y. (2017). Artificial intelligence and com-
puter vision. Springer.
Lubchenco, J. and Grorud-Colvert, K. (2015). Making
waves: The science and politics of ocean protection.
Science, 350(6259):382–383.
Luis, T. (2017). Data mining with R: learning with case
studies. CRC Press, Taylor and Francis Group, Boca
Raton.
Maciejewski, M. (2017). To do more, better, faster and
more cheaply: Using big data in public administra-
tion. International Review of Administrative Sciences,
83(1 suppl):120–135.
Maimon, O. and Rokach, L. (2010). Data mining and
knowledge discovery handbook. Springer, New York.
Miles, M. B. and Huberman, A. M. A. M. (1994). Quali-
tative data analysis: an expanded sourcebook. Sage
Publications, Thousand Oaks, Calif., 2nd ed. edition.
Murthy, P., Bharadwaj, A., Subrahmanyam, P. A., Roy, A.,
and Rajan, S. (2014). Big data taxonomy.
Pinto, V. A. and Parreiras, F. S. (2020). Ontodive: An ontol-
ogy for representing data science initiatives upon big
data technologies. In ICEIS (1), pages 42–51.
Roy, S., Ray, R., Roy, A., Sinha, S., Mukherjee, G., Pyne,
S., Mitra, S., Basu, S., and Hazra, S. (2017). Iot, big
data science & analytics, cloud computing and mo-
bile app based hybrid system for smart agriculture.
In 2017 8th Annual Industrial Automation and Elec-
tromechanical Engineering Conference (IEMECON),
pages 303–304. IEEE.
Santoro, G., Fiano, F., Bertoldi, B., and Ciampi, F. (2018).
Big data for business management in the retail indus-
try. Management Decision.
Seele, P. (2017). Predictive sustainability control: A review
assessing the potential to transfer big data driven ‘pre-
dictive policing’ to corporate sustainability manage-
ment. Journal of Cleaner Production, 153(C):673–
686.
Sharma, K. (2017). Overview of Industrial Process Automa-
tion. Elsevier Science.
Sivarajah, U., Kamal, M. M., Irani, Z., and Weerakkody, V.
(2017). Critical analysis of big data challenges and
analytical methods. Journal of Business Research,
70:263 – 286.
Stoet, G. and Geary, D. C. (2018). The gender-
equality paradox in science, technology, engineering,
and mathematics education. Psychological science,
29(4):581–593.
Takurta, D., Ericson, G., Martens, J., and Severtson, B.
(2017). Deployment stage of the team data science
process lifecycle - azure.
Tal
´
on-Ballestero, P., Gonz
´
alez-Serrano, L., Soguero-Ruiz,
C., Mu
˜
noz-Romero, S., and Rojo-
´
Alvarez, J. L.
(2018). Using big data from customer relationship
management information systems to determine the
client profile in the hotel sector. Tourism Management,
68:187–197.
Van Der Aalst, W. (2016). Data science in action. In Process
Mining, pages 3–23. Springer.
Xu, L. D., He, W., and Li, S. (2014). Internet of things in
industries: A survey. IEEE Transactions on Industrial
Informatics, 10(4):2233–2243.
Zheng, X., Chen, W., Wang, P., Shen, D., Chen, S., Wang,
X., Zhang, Q., and Yang, L. (2015). Big data for so-
cial transportation. IEEE Transactions on Intelligent
Transportation Systems, 17(3):620–630.
Zhou, K., Fu, C., and Yang, S. (2016). Big data driven smart
energy management: From big data to big insights.
Renewable and Sustainable Energy Reviews, 56:215–
225.
InfoMINDS: An Interdisciplinary Framework for Leveraging Data Science upon Big Data in Surface Mining Industry
791