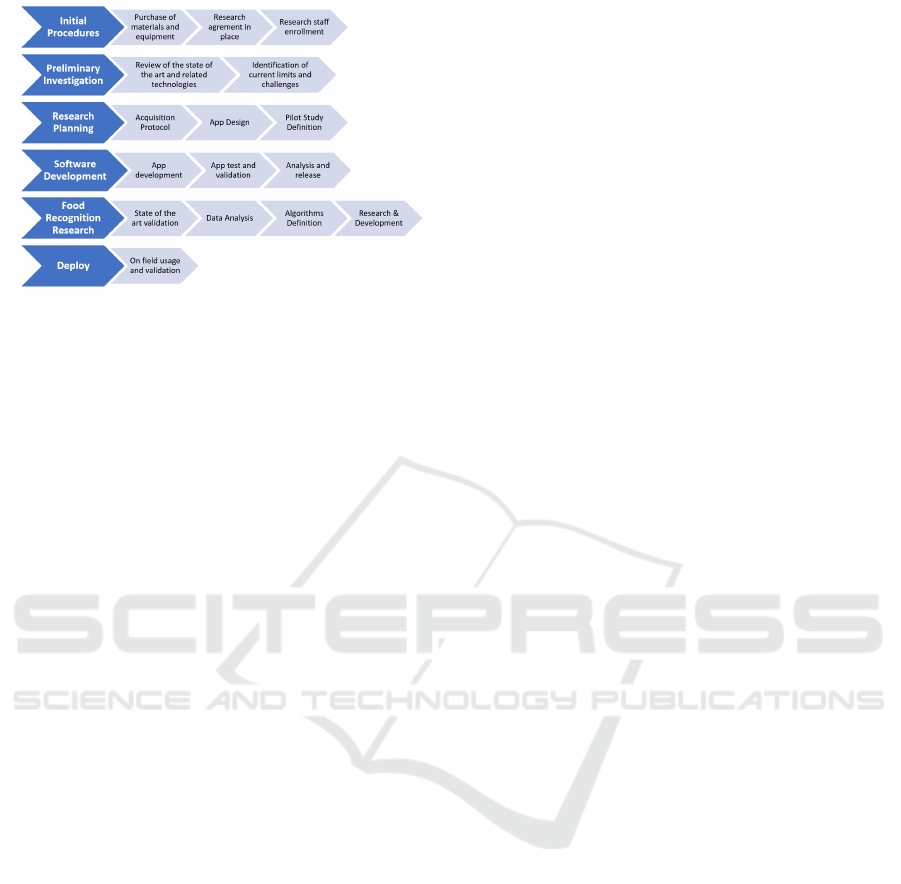
Figure 1: FoodREC project’s phases.
dietary monitoring. Section 4 concludes the paper by
describing the current state of the project and propos-
ing future directions.
2 FoodRec: RESEARCH PLAN
The project involves several phases, which are
sketched by the chart shown in Figure 1. The dia-
gram shows five main phases, the first ones are re-
lated to preliminary studies and research, whereas the
last ones regard the development of algorithms and
software, toward the final deploy of the obtained so-
lutions. With respect to the diagram in Figure 1, we
can group the project’s phases into two main macro-
tasks, which are detailed in the following paragraphs.
2.1 State of the Art Evaluation
After initial procedures (see Figure 1) have been com-
pleted, the research staff focused on the preliminary
investigation of the tasks and research problems re-
lated to the project purposes. This included the study
of the state of the art related to food recognition and
dietary monitoring technologies. As a result, a report
concerning the existing products and approaches in
terms of algorithms and smartphone apps has been
produced and published in (Allegra et al., 2020), de-
tailing the features and performances of each evalu-
ated solution. The results of the study in (Allegra
et al., 2020), revealed that modern food recognition
techniques can support the traditional self-reporting
approaches for eating diary, however, more efforts
should be devoted to the definition of large scale la-
belled image datasets. The new dataset design should
focus on the quality of annotations related to the type
of food, areas, quantities and calories of each food
item depicted in an image. So far, state-of-the-art fo-
cused on specific tasks performed in controlled con-
ditions. The extreme variability of food appearance
makes this task challenging. Especially for ingredi-
ents inference and, hence, for nutritional values es-
timation. The study concludes that food recognition
for dietary monitoring is still an in-progress technol-
ogy, and more efforts are needed to reach standards
for reliable medical protocols, such as smoke quitting
programmes.
2.2 Applied Research and Development
After the study of the state of the art, and conse-
quent analysis and definition of current limits and
challenges, the research moved to the applied research
and development phase. This phase has a dual objec-
tive. One is related to the development of the tech-
nological aspects of the framework, the other one is
related to the development of analysis algorithms.
The iOS/Android FoodRec smartphone app for
image acquisition and analysis, and dietary monitor-
ing has been released, and is currently under testing
by selected users. The mobile app FoodRec has been
designed with the objective of providing a smart and
accessible system for the daily eating habits monitor-
ing of the users, with the definition of a dietary diary.
The innovation that characterize the FoodRec app is
the automatisms related to the food analysis and asso-
ciated inferences. Indeed, the user just uploads a pic-
ture on the system, then all inferences are performed
automatically, by means of Computer Vision and Ar-
tificial Intelligence technologies.
Figure 2 shows the main interface screens of the
FoodRec app. First, a meal over four possibilities is
chosen (a), then the app requires to state the mood
associated to the meal (b), then the picture is taken
(c) and uploaded (d). The app automatically learns
the daytimes associated to food intake, and sends a
notification to the user if the meal has not been in-
serted yet at the expected time. After the image is
uploaded to the server, the recognition algorithms are
applied, and the resulting inferences are shown in the
app interface, as in the example shown in Figure 2.
At this step, the user can edit the results (if needed)
and confirm the new record for the eating diary. The
information about user corrections are exploited for
the further improvements of the algorithms, as well
as their specialization with respect to the specific user
habits.
FoodRec developed features also include water in-
take and weight tracker. Moreover, the user can in-
spect the statistics related to his/her eating habits, in-
cluding the dominant food categories, ingredients, as
well as temporal visualizations of specific parame-
ters (see Figure 3).
Food Recognition for Dietary Monitoring during Smoke Quitting
161