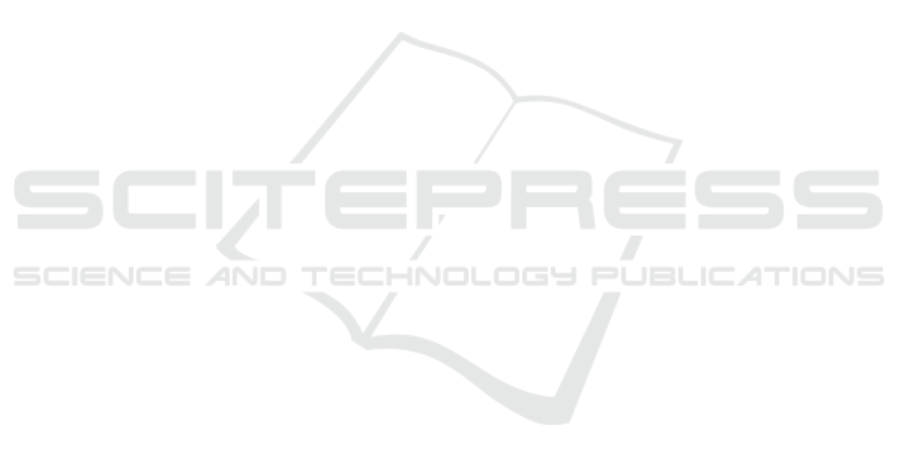
separate him from the students, a friendly interface
and an efficient search filter to search for questions
are among the most relevant answers. Thus, this re-
search was of great value for a brief validation of the
current state of the platform and targeting future fea-
tures.
The data collected endorse the hypotheses that
guided the conception of Probatio. With the use of
Probatio, the time used in preparing a test can be dras-
tically reduced. This is due to the ease of handling and
retrieving questions, the reliability of the stored ques-
tions, due to the revisions that the questions could un-
dergo by educators, in addition to the use of the rec-
ommendation system added to the platform. In addi-
tion to the main recommendation feature, other fea-
tures provided for in Probatio also appear to be on
the teachers’ “wish list”. The questions stored in the
bank could be reviewed and evaluated by the teachers
who use it, thus increasing the reliability in using that
particular question.
6 CONCLUSIONS
We see Probatio as an innovative tool. In its cur-
rent state, Probatio allows questions to be stored in its
question bank and retrieved both by a search process
and by recommendation. Tags and keywords are used
to recommend items through a content-based filter
and later, as a knowledge-based recommender. Users
can retrieve items to prepare their assignments using
a simple interface where they state “what they want”
(criteria for the recommendations) and select, amount
the retrieved items, the ones he or she wants in the
assignment.
Our recommendation system is already able to
deal with several metadata, but by now, metadata are
manually associated to items. As future work, we
intend to implement automatic extraction of relevant
metadata from the items’ text. We are currently eval-
uating the use of machine learning to categorize ques-
tions in the cognitive levels of Bloom’s taxonomy.
Many works already investigated such categorization
of questions written in the English language (Bindra
et al., 2017; Sangodiah et al., 2016; Sangodiah et al.,
2014). As the system is being used in Brazil, a clas-
sifier for Portuguese language is needed. Each new
metadata made available in Probatio must be explic-
itly incorporated in the interface, so that the link be-
tween the chosen criteria and educational objectives
is always in focus.
Automatic feedback could be used in the future to
improve semantic information. For instance, the col-
lection of the percentage of students who correctly an-
swered each question could help to classify the ques-
tions as ”easy”, ”medium” or ”difficult”, or even in
more sophisticated categories in case the type of mis-
take could be automatically identified.
It is also in our agenda the creation of simple dash-
boards where users of the system can have a glimpse
of the tags associated to each item, the most relevant
tags in an assignment, and the tags most used by an
educator.
It is believed that the application of an information
system to support assignment creation and the man-
agement of assignment items has a positive effect not
only for educators, but also for students and educa-
tional institutions. The item bank itself, storing items,
educational metadata and relations among them is a
valuable asset for the institution. Users of the system
are expected to improve their educational skills with
time. Our most ambitious goal is for teachers to learn
more about educational objectives and criteria for set-
ting up assignments, and to make better use of the
feedback that an assessment can provide. In the long
run, it is expected that the continued use of the system
will provide a maturation of educators’ understanding
and perception of the results of his evaluations.
REFERENCES
AIMEUR, H. H. E. (2005). Exam question recommender
system. Artificial Intelligence in Education: Sup-
porting Learning Through Intelligent and Socially In-
formed Technology, 125:249.
Bindra, S. K., Girdhar, A., and Bamrah, I. S. (2017). Out-
come based predictive analysis of automatic question
paper using data mining. In 2017 2nd International
Conference on Communication and Electronics Sys-
tems (ICCES), pages 629–634. IEEE.
Boulis, C. and Ostendorf, M. (2005). Text classification
by augmenting the bag-of-words representation with
redundancy-compensated bigrams. In Proc. of the
International Workshop in Feature Selection in Data
Mining, pages 9–16. Citeseer.
Ferraz, A., Belhot, R. V., et al. (2010). Taxonomia de
bloom: revis
˜
ao te
´
orica e apresentac¸
˜
ao das adequac¸
˜
oes
do instrumento para definic¸
˜
ao de objetivos instru-
cionais. Gest. Prod., S
˜
ao Carlos, 17(2):421–431.
Jayakodi, K., Bandara, M., and Perera, I. (2015). An au-
tomatic classifier for exam questions in engineering:
A process for bloom’s taxonomy. In 2015 IEEE In-
ternational Conference on Teaching, Assessment, and
Learning for Engineering (TALE), pages 195–202.
IEEE.
Kaburlasos, V. G., Marinagi, C. C., and Tsoukalas, V. T.
(2004). Pares: A software tool for computer-based
testing and evaluation used in the greek higher ed-
ucation system. In IEEE International Conference
on Advanced Learning Technologies, 2004. Proceed-
ings., pages 771–773. IEEE.
CSEDU 2021 - 13th International Conference on Computer Supported Education
374