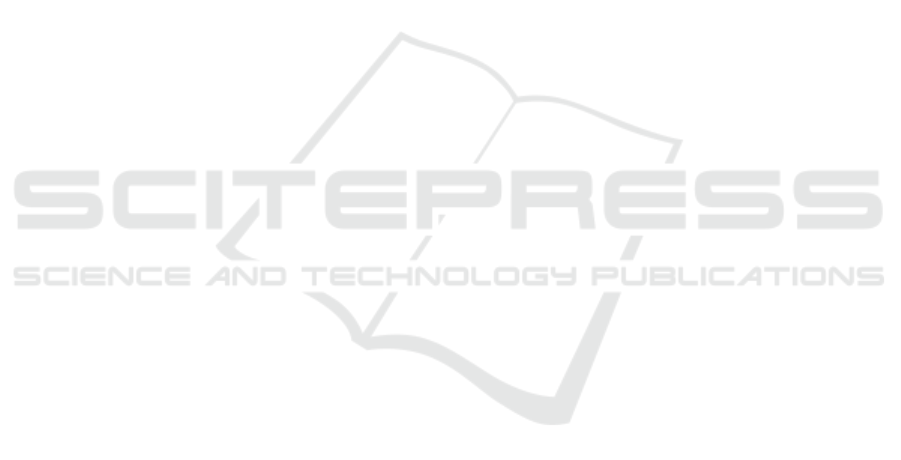
5 DISCUSSION AND
CONCLUSION
The use of CATs has become increasingly popular, es-
pecially during the covid pandemic, where due to the
need for social distance the tests are done via com-
puter. Given this, we emphasize the importance of
discussing the best stopping criteria for fairer exams,
as these directly influence the final result.
The proposed stop criterion, VVAP, presents a
similar performance to the majority of other crite-
ria, however it is worse when compared to the FL
for having a greater standard deviation in the number
of questions. This is also an advantage of the Fixed
Length criterion for all the other criteria considered.
Although many works use mixed stop criteria, it
was observed that it do not seem to improve the mean
RMSE when the full population is considered.
We conclude in favor of the FI criterion, as long as
it can be tuned to the item bank at hand. The FL shows
a competitive precision-efficiency trade-off curve in
every scenario while presenting zero variance in test
length.
The threshold definition methods presented were
important to compare in a fair way all the criteria on
every item bank.
The limitation of the current research is to fix only
the ML3 of the IRT for the calculation of the correct
score probability and to make tests only in simulated
item banks. Future works can be developed using
other models of IRT and using actual item data.
The research was very important for being able to
compare the stop criteria in several scenarios: using
the ML and EAP method, several distributions for the
parameter b of the IRT model, with and without shifts,
and to analyze a large number of trade-offs.
REFERENCES
Babcock, B. and Weiss, D. (2009). Termination criteria in
computerized adaptive tests: Variable-length cats are not
biased. In Proceedings of the 2009 GMAC conference on
computerized adaptive testing, volume 14.
Birnbaum, A. L. (1968). Some latent trait models and their
use in inferring an examinee’s ability. Statistical theories
of mental test scores.
Chang, H.-H. and Ying, Z. (1996). A global information
approach to computerized adaptive testing. Applied Psy-
chological Measurement, 20(3):213–229.
de Andrade, D. F., Tavares, H. R., and da Cunha Valle,
R. (2000). Teoria da resposta ao item: conceitos e
aplicac¸
˜
oes. ABE, S
˜
ao Paulo.
Hambleton, R. K. and Swaminathan, H. (2013). Item re-
sponse theory: Principles and applications. Springer
Science & Business Media.
Kreitzberg, C. B., Stocking, M. L., and Swanson, L. (1978).
Computerized adaptive testing: Principles and direc-
tions. Computers & Education, 2(4):319–329.
Lord, F. M. (1980). Applications of item response theory to
practical testing problems. Routledge.
Morris, S. B., Bass, M., Howard, E., and Neapolitan, R. E.
(2020). Stopping rules for computer adaptive testing
when item banks have nonuniform information. Inter-
national journal of testing, 20(2):146–168.
Sari, H. I. and Raborn, A. (2018). What information works
best?: A comparison of routing methods. Applied psy-
chological measurement, 42(6):499–515.
Segall, D. O. (2005). Computerized adaptive testing. Ency-
clopedia of social measurement, 1:429–438.
Spenassato, D., Bornia, A., and Tezza, R. (2015). Comput-
erized adaptive testing: A review of research and tech-
nical characteristics. IEEE Latin America Transactions,
13(12):3890–3898.
Stafford, R. E., Runyon, C. R., Casabianca, J. M., and
Dodd, B. G. (2019). Comparing computer adaptive test-
ing stopping rules under the generalized partial-credit
model. Behavior research methods, 51(3):1305–1320.
van der Linden, W. J. (1998). Bayesian item selection crite-
ria for adaptive testing. Psychometrika, 63(2):201–216.
van der Linden, W. J. and Glas, C. A. (2000). Computerized
Adaptive Testing: Theory and Practice. Springer Science
& Business Media, Boston, MA.
Veerkamp, W. J. and Berger, M. P. (1997). Some new item
selection criteria for adaptive testing. Journal of Educa-
tional and Behavioral Statistics, 22(2):203–226.
Wainer, H., Dorans, N. J., Flaugher, R., Green, B. F., and
Mislevy, R. J. (2000). Computerized adaptive testing: A
primer. Routledge.
Wang, C., Chang, H.-H., and Huebner, A. (2011). Restric-
tive stochastic item selection methods in cognitive diag-
nostic computerized adaptive testing. Journal of Educa-
tional Measurement, 48(3):255–273.
Comprehensive Empirical Analysis of Stop Criteria in Computerized Adaptive Testing
57