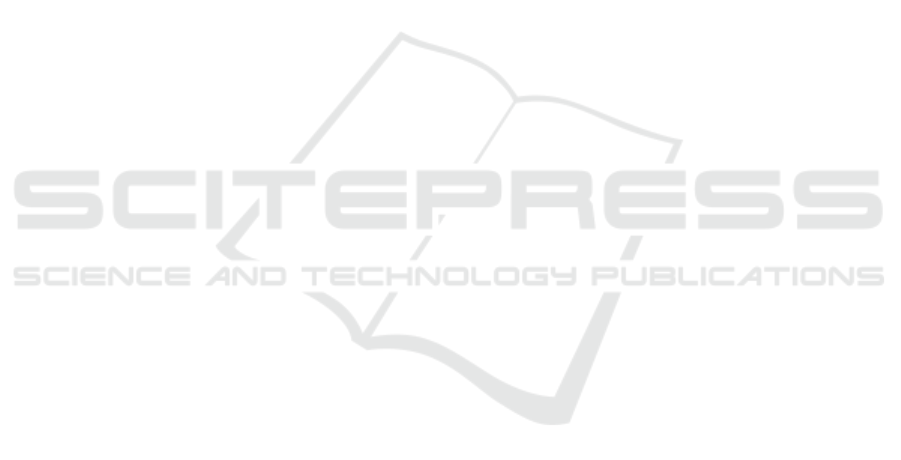
REFERENCES
Bobadilla, J., Ortega, F., Hernando, A., and Gutierrez, A.
(2013). Recommender systems survey. Knowledge-
Based Systems, 46:109 – 132.
Carchiolo, V., Grassia, M., Longheu, A., Malgeri, M., and
Mangioni, G. (2020). A network-based analysis to un-
derstand food-habits of a canteen clients. In Proceed-
ings of the 22nd International Conference on Informa-
tion Integration and Web-based Applications Services
iiWAS ’20.
Carchiolo, V., Grassia, M., Longheu, A., Malgeri, M., and
Mangioni, G. (2021). A network-based analysis of
a worksite canteen dataset. Big Data and Cognitive
Computing, 5(1).
Carchiolo, V., Longheu, A., Barbera, S., and Tommaso, P.
(2019). Personalized wellness in smart offices. In Pro-
ceedings of IADIS International Conference on Ap-
plied Computing 2019, pages 97–104.
Elsweiler, D., Trattner, C., and Harvey, M. (2017). Ex-
ploiting food choice biases for healthier recipe rec-
ommendation. In Proceedings of the 40th Interna-
tional ACM SIGIR Conference on Research and De-
velopment in Information Retrieval, SIGIR ’17, page
575–584, New York, NY, USA. Association for Com-
puting Machinery.
Freyne, J. and Berkovsky, S. (2010). Intelligent food plan-
ning: Personalized recipe recommendation. In Pro-
ceedings of the 15th International Conference on In-
telligent User Interfaces, IUI ’10, page 321–324, New
York, NY, USA. Association for Computing Machin-
ery.
Freyne, J., Berkovsky, S., and Smith, G. (2011). Recipe
recommendation: Accuracy and reasoning. In Pro-
ceedings of the 19th International Conference on User
Modeling, Adaption, and Personalization, UMAP’11,
page 99–110, Berlin, Heidelberg. Springer-Verlag.
Ge, M., Elahi, M., Ferna
´
andez-Tob
´
ıas, I., Ricci, F., and
Massimo, D. (2015). Using tags and latent factors in a
food recommender system. In Proceedings of the 5th
International Conference on Digital Health 2015, DH
’15, page 105–112, New York, NY, USA. Association
for Computing Machinery.
Harvey, M. and Elsweiler, D. (2015). Automated recom-
mendation of healthy, personalised meal plans. In Pro-
ceedings of the 9th ACM Conference on Recommender
Systems, RecSys ’15, page 327–328, New York, NY,
USA. Association for Computing Machinery.
Harvey, M. and Elsweiler, D. (2017). You are what you eat:
Learning user tastes for rating prediction. In Springer,
C., editor, Kurland O., Lewenstein M., Porat E. (eds)
String Processing and Information Retrieval. SPIRE
2013., Lecture Notes in Computer Science.
HBS-Digital-Initiative (2020). Caviar - premium bites at
your doorstep. last-access: 2020.
Iwendi, C., Khan, S., Anajemba, J. H., Bashir, A. K., and
Noor, F. (2020). Realizing an efficient iomt-assisted
patient diet recommendation system through machine
learning model. IEEE Access, 8:28462–28474.
Jiang, H., Wang, W., Liu, M., Nie, L., Duan, L.-Y., and
Xu, C. (2019). Market2dish: A health-aware food
recommendation system. In Proceedings of the 27th
ACM International Conference on Multimedia, MM
’19, page 2188–2190, New York, NY, USA. Associa-
tion for Computing Machinery.
Loria, M. P., Toja, M., Carchiolo, V., and Malgeri, M.
(2017). An efficient real-time architecture for col-
lecting iot data. In 2017 Federated Conference on
Computer Science and Information Systems (FedC-
SIS), pages 1157–1166.
Merler, M., Wu, H., Uceda-Sosa, R., Nguyen, Q.-B., and
Smith, J. R. (2016). Snap, eat, repeat: A food recog-
nition engine for dietary logging. In Proceedings of
the 2nd International Workshop on Multimedia As-
sisted Dietary Management, MADiMa ’16, page 31–
40, New York, NY, USA. Association for Computing
Machinery.
Min, W., Jiang, S., Liu, L., Rui, Y., and Jain, R. (2019).
A survey on food computing. ACM Comput. Surv.,
52(5).
Mohamed, M., Khafagy, M., and Ibrahim, M. (2019). Rec-
ommender systems challenges and solutions survey.
Nations, U. (2015). Transforming our world: the 2030
agenda for sustainable development. Technical report.
https://sustainabledevelopment.un.org/resourcelibrary.
Runo, M. and Wattenhofer, R. (2011). Foodroid: A food
recommendation app for university canteens. Master’s
thesis, ETH Zurich, Zurich, Switzerland.
Torreggiani, S., Mangioni, G., Puma, M. J., and Fagiolo,
G. (2018). Identifying the community structure of the
food-trade international multi-network. Environmen-
tal Research Letters, 13(5):054026.
Yang, L., Hsieh, C.-K., Yang, H., Pollak, J. P., Dell, N.,
Belongie, S., Cole, C., and Estrin, D. (2017). Yum-
me: A personalized nutrient-based meal recommender
system. ACM Trans. Inf. Syst., 36(1).
Zhang, M., Tian, G., Zhang, Y., and Duan, P. (2021). Rein-
forcement learning for logic recipe generation: Bridg-
ing gaps from images to plans. IEEE Transactions on
Multimedia, pages 1–1.
Zhang, W., Yu, Q., Siddiquie, B., Divakaran, A., and Sawh-
ney, H. (2015). Snap-n-eat: Food recognition and nu-
trition estimation on a smartphone. Journal of dia-
betes science and technology, 9(3).
Zhang, X., Luo, X., and Chen, B. (2020). Multi-view visual
bayesian personalized ranking for restaurant recom-
mendation. Applied Intelligence.
COMPLEXIS 2021 - 6th International Conference on Complexity, Future Information Systems and Risk
124