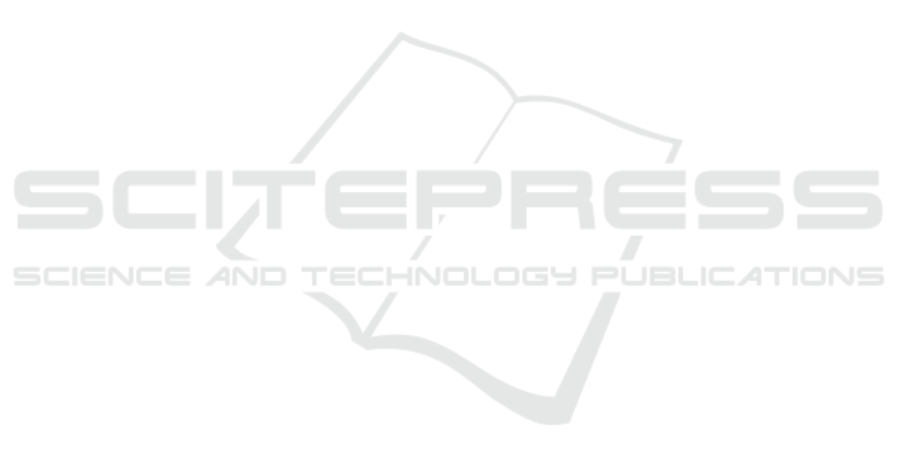
calculation result demonstrates that the average util-
ity value for the big data readiness index is 0.29955,
which lies between the unified utility value of readi-
ness of level 2 and 3.
4 CONCLUSIONS
In this paper, we presented a five-dimensional Big
data readiness assessment framework and a Demp-
ster–Shafer big data readiness assessment model. We
also presented a numerical example of the framework
and the model on an organization that we have eval-
uated prior. We have found that there will always
be uncertainty when assessing organizations’ big data
readiness, as illustrated clearly on the score in the ta-
bles above. It could be from to incomplete informa-
tion or various background knowledge of each asses-
sor. Our framework and model wield the uncertainty
into a more practical big data readiness standing for
the organization.
In our numerical example, we were able to cal-
culate a big data readiness assessment standing of
0.29985 for the organization. This calculation pro-
vides the organization with a more concrete standing
that can be used as a baseline score. The computed
readiness standing score puts the organization in be-
tween level 2 and level 3 standing. It indicates that
the organization is moving toward an analytic aspi-
ration organization, but it still has a couple of levels
to improve toward being considered a big data ready
organization.
REFERENCES
Bappy, M. M., Ali, S. M., Kabir, G., and Paul, S. K. (2019).
Supply chain sustainability assessment with dempster-
shafer evidence theory: Implications in cleaner pro-
duction. Journal of Cleaner Production, 237:117771.
Berndtsson, M., Forsberg, D., Stein, D., and Svahn, T.
(2018). Becoming a data-driven organisation.
Buitelaar, R. (2018). Building the data-driven organization:
a maturity model and assessment. Master’s thesis,
Leiden Institute of Advanced Computer Science (LI-
ACS), The Netherlands.
Hafeez, M. (2011). Application of dempster shafer theory to
assess the status of sealed fire in a cole mine. Master’s
thesis, , School of Engineering.
Kolbjørnsrud, V., Andersen, E., Johnson, J., and Ragnvald,
S. (2018). The data-driven organization: Intelligence
at SCALE, pages 23–42.
Li, L., Chang, Q., and Ni, J. (2009). Data driven bottle-
neck detection of manufacturing systems. Interna-
tional Journal of Production Research, 47(18):5019–
5036.
Lusher, S. J., McGuire, R., [van Schaik], R. C., Nicholson,
C. D., and [de Vlieg], J. (2014). Data-driven medicinal
chemistry in the era of big data. Drug Discovery To-
day, 19(7):859 – 868. Theme sections: • Huntington’s
Disease. Guest Editor: Craig Blackstone • Exercise
Physiology. Guest Editor: Pontus Almer Bostrom.
Pongpech, W. A. (2018). On application of learning to rank
for assets management: Warehouses ranking. In Yin,
H., Camacho, D., Novais, P., and Tall
´
on-Ballesteros,
A. J., editors, Intelligent Data Engineering and Au-
tomated Learning - IDEAL 2018 - 19th International
Conference, Madrid, Spain, November 21-23, 2018,
Proceedings, Part I, volume 11314 of Lecture Notes
in Computer Science, pages 336–343. Springer.
Pongpech, W. A. (2019). Modeling data driven interac-
tions on property graph. In Yin, H., Camacho, D.,
Ti
˜
no, P., Tall
´
on-Ballesteros, A. J., Menezes, R., and
Allmendinger, R., editors, Intelligent Data Engineer-
ing and Automated Learning - IDEAL 2019 - 20th
International Conference, Manchester, UK, Novem-
ber 14-16, 2019, Proceedings, Part I, volume 11871
of Lecture Notes in Computer Science, pages 68–75.
Springer.
Tehrany, M. and Kumar, L. (2018). The application of
a dempster–shafer-based evidential belief function in
flood susceptibility mapping and comparison with fre-
quency ratio and logistic regression methods. Environ-
mental Earth Sciences, 77.
Wang, Y.-M., Yang, J.-B., Xu, D.-L., and Chin, K.-S.
(2009). The evidential reasoning approach for mul-
tiple attribute decision analysis using interval belief
degrees. European Journal of Operational Research,
175:35–66.
A Dempster–Shafer Big Data Readiness Assessment Model
585