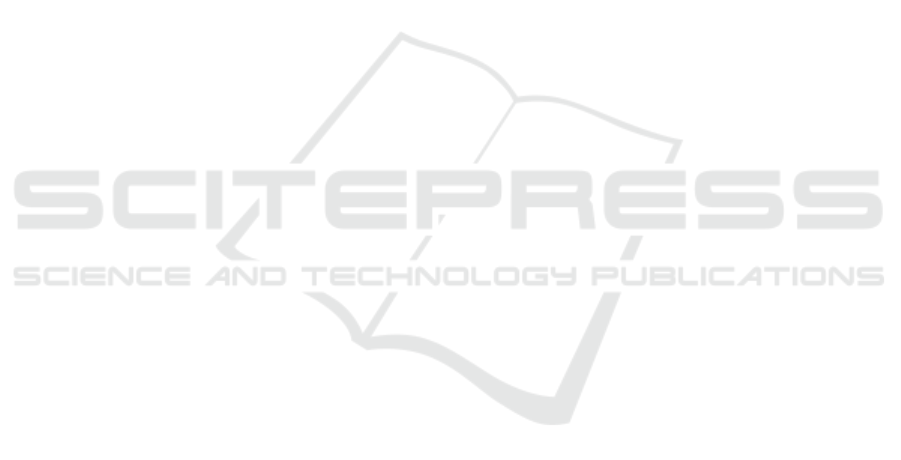
Javed, M., Abgaz, Y. M., and Pahl, C. (2013). Ontology
change management and identification of change pat-
terns. J. Data Semant., 2(2-3):119–143.
Kenny, C. and Pahl, C. (2005). Automated tutoring for a
database skills training environment. In 36th Tech-
nical Symposium on Computer Science Education,
SIGCSE, pages 58–62. ACM.
Kleiman, R. and Page, D. (2019). Aucµ: A performance
metric for multi-class machine learning models. In
Intl Conference on Machine Learning, pages 3439–
3447.
Mahdavinejad, M. S., Rezvan, M., Barekatain, M., Adibi,
P., Barnaghi, P., and Sheth, A. P. (2018). Machine
learning for internet of things data analysis: a sur-
vey. Digital Communications and Networks, 4(3):161
– 175.
Melia, M. and Pahl, C. (2009). Constraint-based valida-
tion of adaptive e-learning courseware. IEEE Trans.
Learn. Technol., 2(1):37–49.
Murray, S., Ryan, J., and Pahl, C. (2003). Tool-mediated
cognitive apprenticeship approach for a computer en-
gineering course. In International Conference on Ad-
vanced Learning Technologies, pages 2–6. IEEE.
Nguyen, T. L. (2018). A framework for five big vs of big
data and organizational culture in firms. In Interna-
tional Conference on Big Data.
O’Brien, T., Helfert, M., and Sukumar, A. (2013). The value
of good data- a quality perspective a framework and
discussion. In International Conference on Enterprise
Information Systems.
Pahl, C., Barrett, R., and Kenny, C. (2004). Supporting
active database learning and training through interac-
tive multimedia. In 9th Conference on Innovation and
Technology in Computer Science Education, ITiCSE,
pages 27–31. ACM.
Pahl, C., Fronza, I., Ioini, N. E., and Barzegar, H. R. (2019).
A review of architectural principles and patterns for
distributed mobile information systems. In Interna-
tional Conference on Web Information Systems and
Technologies.
Pahl, C., Ioini, N. E., Helmer, S., and Lee, B. A. (2018).
An architecture pattern for trusted orchestration in iot
edge clouds. In Intl Conf on Fog and Mobile Edge
Computing. IEEE.
Plewczynski, D., Spieser, S. A. H., and Koch, U. (2006).
Assessing different classification methods for virtual
screening. Journal of Chemical Information and Mod-
eling, 46(3):1098–1106.
Saha, B. and Srivastava, D. (2014). Data quality: The other
face of big data. In 2014 IEEE 30th International
Conference on Data Engineering, pages 1294–1297.
IEEE.
Scolati, R., Fronza, I., Ioini, N. E., Samir, A., and Pahl,
C. (2019). A containerized big data streaming archi-
tecture for edge cloud computing on clustered single-
board devices. In Intl Conf on Cloud Computing and
Services Science.
Sicari, S., Rizzardi, A., Miorandi, D., Cappiello, C., and
Coen-Porisini, A. (2016). A secure and quality-aware
prototypical architecture for the internet of things. In-
formation Systems, 58:43 – 55.
Sridhar, V., Subramanian, S., Arteaga, D., Sundararaman,
S., Roselli, D. S., and Talagala, N. (2018). Model
governance: Reducing the anarchy of production ml.
In USENIX Annual Technical Conference.
Thatipamula, S. (2013). Data done right: 6 di-
mensions of data quality. https://smartbridge.com/
data-done-right-6- dimensions-of-data-quality/. Ac-
cessed on 2021-01-16.
von Leon, D., Miori, L., Sanin, J., Ioini, N. E., Helmer, S.,
and Pahl, C. (2018). A performance exploration of ar-
chitectural options for a middleware for decentralised
lightweight edge cloud architectures. In Intl Conf on
Internet of Things, Big Data and Security, pages 73–
84.
von Leon, D., Miori, L., Sanin, J., Ioini, N. E., Helmer, S.,
and Pahl, C. (2019). A lightweight container middle-
ware for edge cloud architectures. In Fog and Edge
Computing, Wiley Series on Parallel and Distributed
Computing, pages 145–170. Wiley.
Continuous Data Quality Management for Machine Learning based Data-as-a-Service Architectures
335