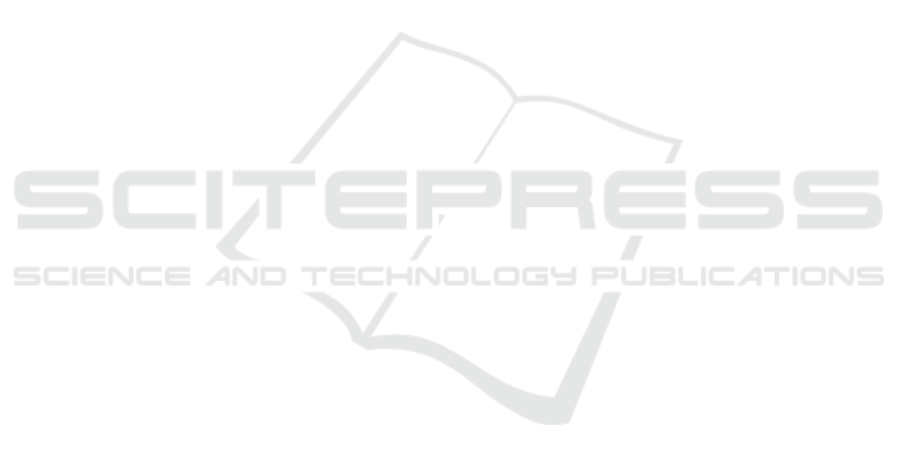
In Figure 5, the blocking probability is investi-
gated. From Figure 5a, as the arrival rate increases
the blocking probability for the arrival packets in-
creases. That is because, when the number of arriving
packets increases, the probability that the finite queue
size reaches its maximum value increases which in
turns increases the blocking probability. From Fig-
ure 5b, for low idle channel probabilities, the HTT
mode gives the highest blocking probability because
there is almost no opportunity to transmit any pack-
ets which consequently accumulate the data packets
in the queue till it reaches its maximum capacity and
blocks any further arrival packets. The blocking prob-
ability of the HTT mode decreases as the idle proba-
bility increases. However, when the idle probability is
greater than 0.5, the blocking probability of the HTT
mode starts to increase in a pattern similar to the other
modes.
5 CONCLUSIONS
In this paper, we applied a reinforcment learning ap-
proach to study a hybrid HTT/backscattering trans-
mission mode of an RF-powered CR network. The
average throughput and the blocking probability for
the SU are investigated for the incomplete informa-
tion channel case under the unknown environment pa-
rameters assumption. Numerical results showed that
the performance of the proposed hybrid mode is better
than that of using HTT and backscattering transmis-
sion modes especially for the case of heavy SU loads
or small PU idle probability. Finally, the proposed
model can be extended by considering the mode and
channel selection problem in a multi-channel RF-
powered cognitive radio networks composed of mul-
tiple SUs, where the optimization problem is more
challenging.
ACKNOWLEDGEMENTS
This work is supported by the National Telecom Reg-
ulatory Authority (NTRA) of Egypt under the project
entitled ”Security-Reliability Tradeoff in Spectrum
Sharing Networks with Energy Harvesting”. Ahmed
Y. Zakariya acknowledges also the support from the
Missions Sector of the Higher Education Ministry in
Egypt through Ph.D. scholarship.
REFERENCES
Anh, T. T., Luong, N. C., Niyato, D., Liang, Y.-C., and Kim,
D. I. (2019). Deep reinforcement learning for time
scheduling in RF-powered backscatter cognitive radio
networks. In IEEE Wireless Communications and Net-
working Conference (WCNC), pages 1–7.
Fahim, T. E., Zakariya, A. Y., and Rabia, S. I. (2018). A
novel hybrid priority discipline for multi-class sec-
ondary users in cognitive radio networks. Simulation
Modelling Practice and Theory, 84:69–82.
Gouda, A. E., Rabia, S. I., Zakariya, A. Y., and Omar, M.
(2018). Reactive spectrum handoff combined with
random target channel selection in cognitive radio net-
works with prioritized secondary users. Alexandria
engineering journal, 57(4):3219–3225.
Hoang, D. T., Niyato, D., Wang, P., and Kim, D. I. (2014).
Opportunistic channel access and RF energy harvest-
ing in cognitive radio networks. IEEE Journal on Se-
lected Areas in Communications, 32(11):2039–2052.
Liu, V., Parks, A., Talla, V., Gollakota, S., Wetherall, D.,
and Smith, J. R. (2013). Ambient backscatter: Wire-
less communication out of thin air. ACM SIGCOMM
Computer Communication Review, 43(4):39–50.
Lu, X., Wang, P., Niyato, D., and Hossain, E. (2014). Dy-
namic spectrum access in cognitive radio networks
with RF energy harvesting. IEEE Wireless Commu-
nications, 21(3):102–110.
Niyato, D., Wang, P., and Kim, D. I. (2014). Channel selec-
tion in cognitive radio networks with opportunistic RF
energy harvesting. In IEEE International Conference
on Communications (ICC), pages 1555–1560.
Park, S., Kim, H., and Hong, D. (2013). Cognitive radio
networks with energy harvesting. IEEE Transactions
on Wireless Communications, 12(3):1386–1397.
Sigaud, O. and Buffet, O. (2013). Markov decision pro-
cesses in artificial intelligence. John Wiley & Sons.
Van Huynh, N., Hoang, D. T., Lu, X., Niyato, D., Wang, P.,
and Kim, D. I. (2018a). Ambient backscatter commu-
nications: A contemporary survey. IEEE Communi-
cations Surveys & Tutorials, 20(4):2889–2922.
Van Huynh, N., Hoang, D. T., Nguyen, D. N., Dutkiewicz,
E., Niyato, D., and Wang, P. (2018b). Reinforce-
ment learning approach for RF-powered cognitive ra-
dio network with ambient backscatter. In IEEE Global
Communications Conference (GLOBECOM), pages
1–6.
Van Huynh, N., Hoang, D. T., Nguyen, D. N., Dutkiewicz,
E., Niyato, D., and Wang, P. (2019). Optimal and low-
complexity dynamic spectrum access for RF-powered
ambient backscatter system with online reinforcement
learning. IEEE Transactions on Communications,
67(8):5736–5752.
Wang, B. and Liu, K. R. (2010). Advances in cognitive
radio networks: A survey. IEEE Journal of Selected
Topics in Signal Processing, 5(1):5–23.
Weaver, L. and Tao, N. (2001). The optimal reward baseline
for gradient-based reinforcement learning. Proceed-
ings of the Seventeenth Conference on Uncertainty in
Artificial Intelligence, pages 538–545.
Dynamic Spectrum Access for RF-powered Ambient Backscatter Cognitive Radio Networks
229