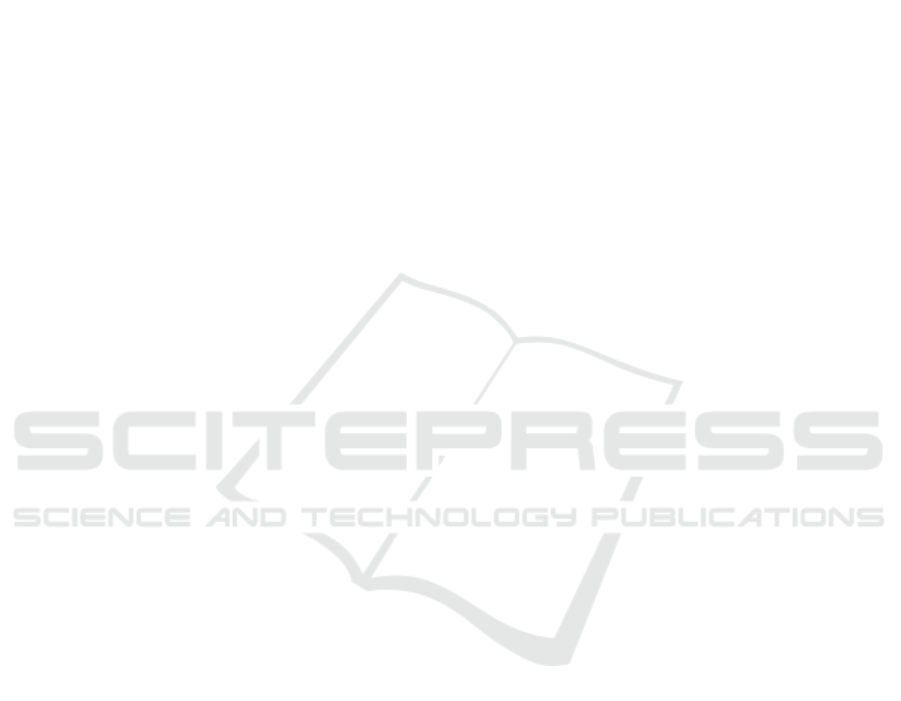
Three-year Trends in YouTube Video Content and Encoding
Feng Li
1
, Jae Won Chung
2
and Mark Claypool
3
1
Verizon Labs, 60 Sylvan Rd., Waltham, MA, U.S.A.
2
Viasat Inc., 300 Nickerson Rd., Marlborough, MA, U.S.A.
3
Worcester Polytechnic Institute, 100 Institute Rd., Worcester, MA, U.S.A.
Keywords:
YouTube, Internet Video, Internet Video Analysis, Video Crawler.
Abstract:
Despite the dominance of YouTube streaming traffic, there have been few studies focusing on characterizing
YouTube videos over time. Given the sheer volume of YouTube videos, we created a custom crawler which
took snapshots of popular YouTube channels and ran the crawler daily for the past 3 years. This provides
YouTube video trends from 2018–2020 for over 160k videos, considering media type, duration, bit rate, res-
olution, codec, encoding format, and popularity. Analysis of the data shows YouTube videos have increased
frame rates, resolutions and durations over this time, with the biggest clips consuming over 200 Mb/s and be-
ing over 3 hours long, accompanied by corresponding changes in encoding rates and codecs. Our analysis and
the resulting dataset we make public should be beneficial for traffic shaping or CDN deployment strategies.
1 INTRODUCTION
Video use on the Internet has grown tremendously
over the past decade, with video (business and con-
sumer) projected to consumed 79% of all Internet
traffic in 2020 (Cisco Inc, 2016), up from 63%
in 2015. Among the myriad video applications,
YouTube is perhaps the most successful with 2 billion
monthly users and 500 hours of video uploaded ev-
ery minute (MerchDope, 2020). On mobile networks,
YouTube makes up more than 22% of the traffic (Li
et al., 2018b). Understanding the video characteristics
of YouTube can help network traffic management, en-
gineering and optimization.
The increased deployment of end-to-end encryp-
tion, such as HTTP3/QUIC (Langley et al., 2017), has
made it harder for Internet Service Providers (ISPs) to
detect and manage traffic over their networks (Kakhki
et al., 2016). While various detection mechanisms
for encrypted traffic have been proposed (Dimopou-
los et al., 2016; Li et al., 2018a; Tsilimantos et al.,
2018), most require video flow data, such as duration
and data rate, for training. If designers of such al-
gorithms had longitudinal data – video characteristics
over time – they could develop algorithms that are re-
silient to the evolution video characteristics.
With this in mind, we established a “video
crawler” project that monitors video characteris-
tics mined from popular YouTube channel lists and
launched it several years ago. We expect to observe
and record the evolution of YouTube video technolo-
gies, provide “ground truth” data to improve video
detection algorithms, and capture some social char-
acteristics of popular videos based on their views.
To provide a better understanding of Internet
video over time, this paper presents an in-depth mea-
surement study on video statistics from the world’s
leading provider – YouTube – for three years (2018-
2020), with statistics for over 160,000 distinct videos,
accounting for 3.2 million media clips. Analysis
shows YouTube videos have changed significantly
from earlier studies (Cheng et al., 2008; Li et al.,
2005) in their durations, bitrates, and codecs used, af-
firming the need for more recent data. Analysis of
social use shows viral view patterns where a small set
of videos are viewed a lot more than others, indicating
opportunities for new caching strategies to enhance
YouTube service quality over edge networks.
The rest of the paper is organized as follows: Sec-
tion 2 presents related research; Section 3 depicts
our measurement architecture; Section 4 analyzes the
statistics collected; and Section 5 summarizes our
conclusion and presents possible future work.
2 RELATED WORK
While YouTube dominates Internet traffic in terms of
volume, most YouTube measurement work has fo-
cused on social aspects (Bärtl, 2018; Brodersen et al.,
Li, F., Chung, J. and Claypool, M.
Three-year Trends in YouTube Video Content and Encoding.
DOI: 10.5220/0010515800150022
In Proceedings of the 18th International Conference on Signal Processing and Multimedia Applications (SIGMAP 2021), pages 15-22
ISBN: 978-989-758-525-8
Copyright
c
2021 by SCITEPRESS – Science and Technology Publications, Lda. All rights reserved
15