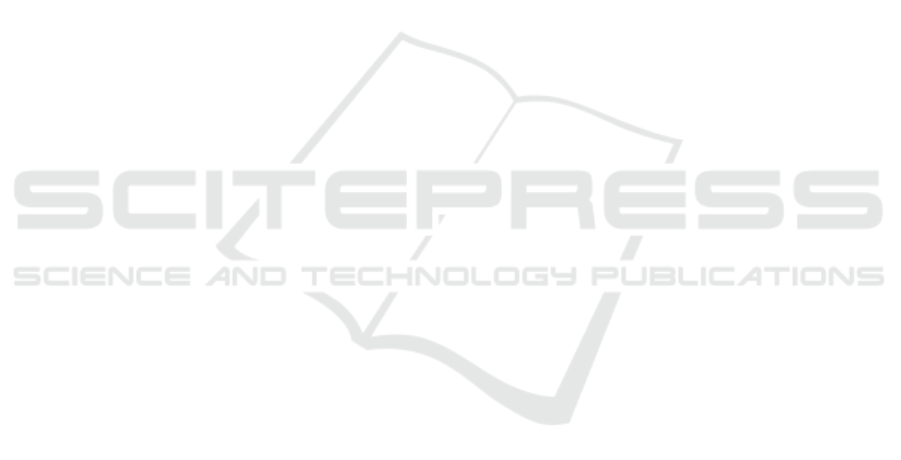
Egger, J., Pepe, A., Gsaxner, C., and Li, J. (2020). Deep
learning–a first meta-survey of selected reviews across
scientific disciplines and their research impact. arXiv
preprint arXiv:2011.08184.
Farhadi, A. and Redmon, J. (2018). Yolov3: An incremental
improvement. Computer Vision and Pattern Recogni-
tion.
Fukui, H., Hirakawa, T., Yamashita, T., and Fujiyoshi, H.
(2019). Attention branch network: Learning of atten-
tion mechanism for visual explanation. In Proc. of the
IEEE Conf. on Computer Vision and Pattern Recogni-
tion, pages 10705–10714.
Garay-Vitoria, N., Cearreta, I., and Larraza-Mendiluze, E.
(2019). Application of an ontology-based platform for
developing affective interaction systems. IEEE Ac-
cess, 7:40503–40515.
Gilda, S., Zafar, H., Soni, C., and Waghurdekar, K. (2017).
Smart music player integrating facial emotion recog-
nition and music mood recommendation. In Int. Conf.
on Wireless Communications, Signal Processing and
Networking, pages 154–158. IEEE.
Grassi, M. (2009). Developing heo human emotions on-
tology. In Fierrez, J., Ortega-Garcia, J., Esposito, A.,
Drygajlo, A., and Faundez-Zanuy, M., editors, Bio-
metric ID Management and Multimodal Communi-
cation, pages 244–251, Berlin, Heidelberg. Springer
Berlin Heidelberg.
Graterol, W., Diaz-Amado, J., Cardinale, Y., Dongo, I.,
Lopes-Silva, E., and Santos-Libarino, C. (2021).
Emotion detection for social robots based on nlp trans-
formers and an emotion ontology. Sensors, 21(4).
Katifori, A., Golemati, M., Vassilakis, C., Lepouras, G., and
Halatsis, C. (2007). Creating an ontology for the user
profile: Method and applications. pages 407–412.
Kaur, R. and Kautish, S. (2019). Multimodal sentiment
analysis: A survey and comparison. Int. Jrnl. of Ser-
vice Science, Management, Engineering, and Technol-
ogy, 10(2):38–58.
Knapp, M. L., Hall, J. A., and Horgan, T. G. (2013). Non-
verbal communication in human interaction, chapter
1: “Nonverbal Communication: Basic Perspectives”.
Cengage Learning, Boston, MA.
Kosti, R., Alvarez, J., Recasens, A., and Lapedriza, A.
(2019). Context based emotion recognition using
emotic dataset. IEEE Transactions on Pattern Anal-
ysis and Machine Intelligence.
Kosti, R., Alvarez, J. M., Recasens, A., and Lapedriza, A.
(2017). Emotion recognition in context. In IEEE Conf.
on Computer Vision and Pattern Recognition.
Lee, J., Kim, S., Kim, S., Park, J., and Sohn, K. (2019).
Context-aware emotion recognition networks. In The
IEEE Int. Conf. on Computer Vision.
Lhommet, M., Marsella, S. C., Calvo, R., D’Mello, S.,
Gratch, J., and Kappas, A. (2015). The Oxford Hand-
book of Affective Computing, chapter “Expressing
Emotion Through Posture and Gesture”.
Lin, R., Amith, M. T., Liang, C., Duan, R., Chen, Y., and
Tao, C. (2018a). Visualized emotion ontology: A
model for representing visual cues of emotions. BMC
Medical Informatics and Decision Making, 18.
Lin, R., Liang, C., Duan, R., Chen, Y., Tao, C., et al.
(2018b). Visualized emotion ontology: a model for
representing visual cues of emotions. BMC medical
informatics and decision making, 18(2):64.
Liu, K., Li, Y., Xu, N., and Natarajan, P. (2018). Learn
to combine modalities in multimodal deep learning.
arXiv preprint arXiv:1805.11730.
Mittal, T., Guhan, P., Bhattacharya, U., Chandra, R., Bera,
A., and Manocha, D. (2020). Emoticon: Context-
aware multimodal emotion recognition using frege’s
principle. In Proc. of the IEEE/CVF Conf. on Com-
puter Vision and Pattern Recognition, pages 14234–
14243.
Noroozi, F., Kaminska, D., Corneanu, C. A., Sapinski, T.,
Escalera, S., and Anbarjafari, G. (2018). Survey on
emotional body gesture recognition. IEEE Transac-
tions on Affective Computing.
Parkhi, O. M., Vedaldi, A., and Zisserman, A. (2015). Deep
face recognition. In British Machine Vision Conf.
Perez-Gaspar, L.-A., Caballero-Morales, S.-O., and
Trujillo-Romero, F. (2016). Multimodal emo-
tion recognition with evolutionary computation for
human-robot interaction. Expert Systems with Appli-
cations, 66:42–61.
Pinto-De la Gala, A., Cardinale, Y., Dongo, I., and Ticona-
Herrera, R. (2021). Towards an ontology for urban
tourism. In Proc. of the 36th Annual ACM Symp. on
Applied Computing, SAC ’21, New York, NY, USA.
ACM.
Plutchik, R. (1980). A general psychoevolutionary theory of
emotion. In Theories of emotion, pages 3–33. Elsevier.
Shi, L., Zhang, Y., Cheng, J., and Lu, H. (2019). Skeleton-
based action recognition with directed graph neural
networks. In The IEEE Conf. on Computer Vision and
Pattern Recognition.
Soleymania, M., Garcia, D., Jouc, B., Schuller, B., Chang,
S.-F., and Pantic, M. (2017). A survey of multimodal
sentiment analysis. Image and Vision Computing,
65:3–14.
Sun, K., Xiao, B., Liu, D., and Wang, J. (2019). Deep high-
resolution representation learning for human pose es-
timation. In The IEEE Conf. on Computer Vision and
Pattern Recognition.
Zadeh, M. M. T., Imani, M., and Majidi, B. (2019). Fast
facial emotion recognition using convolutional neural
networks and gabor filters. In 5th Conf. on Knowledge
Based Engineering and Innovation, pages 577–581.
IEEE.
Zhang, L., Wang, S., and Liu, B. (2018). Deep learning
for sentiment analysis: A survey. Wiley Interdisci-
plinary Reviews: Data Mining and Knowledge Dis-
covery, 8(4):e1253.
Zhang, S.-F., Zhai, J.-H., Xie, B.-J., Zhan, Y., and Wang,
X. (2019). Multimodal representation learning: Ad-
vances, trends and challenges. In Int. Conf. on Ma-
chine Learning and Cybernetics, pages 1–6. IEEE.
Zhang, X., Hu, B., Chen, J., and Moore, P. (2013).
Ontology-based context modeling for emotion recog-
nition in an intelligent web. World Wide Web,
16(4):497–513.
ICSOFT 2021 - 16th International Conference on Software Technologies
464