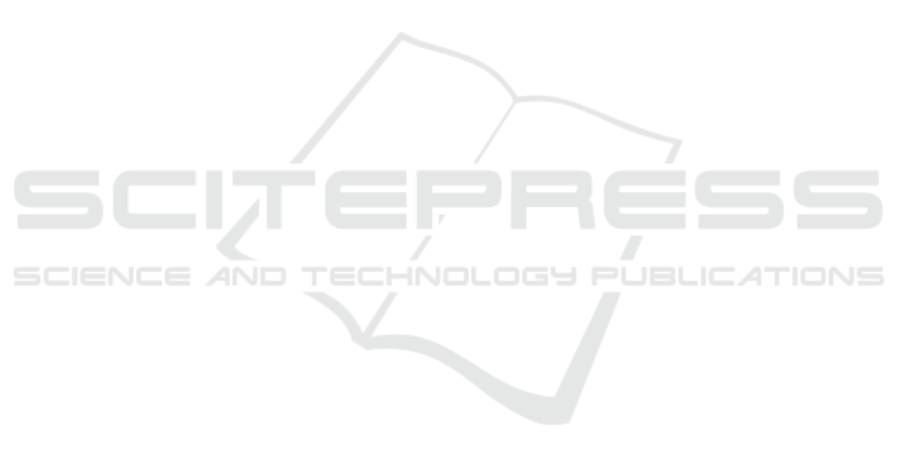
process and gain a better understanding of the overall
requirements.
Despite applying a high level of rigor, our research
is subject to several limitations. First, our study can-
not be free from researcher bias. The paper selec-
tion process during the SLR and the validation of our
model are subjective and were influenced by the re-
searchers’ experiences and backgrounds. Second, the
validation of our model is currently based on assign-
ing research topics. It lacks a practical evaluation in
the form of an application to a real-world develop-
ment project.
Based on our findings and limitations, we see
promising directions for future work. We plan to use
our model in different organizational settings to fur-
ther evaluate its validity. Specifically, we plan to use
DERM as part of a requirements engineering work-
shop in a development project for a machine-learning
application. It will hereby act as canvas, where the
participants can place the derived requirements and
ideas as sticky notes. The feedback from this work-
shop will help us extend or adapt our model to meet
the expectations of software and data engineers. Ad-
ditionally, we will follow up on some research topics
presented in Section 5 to create a deeper knowledge of
engineering data-intensive applications within these
areas.
ACKNOWLEDGMENTS
This work was funded by the Fraunhofer-Cluster of
Excellence »Cognitive Internet Technologies«.
REFERENCES
Alladi, B. S. and Prasad, S. (2018). Big data life cycle:
security issues, challenges, threat and security model.
International Journal of Engineering & Technology,
7(3):100–103.
Allanic, M., Hervé, P.-Y., Pham, C.-C., Lekkal, M., Durupt,
A., Brial, T., Grioche, A., Matta, N., Boutinaud, P.,
Eynard, B., et al. (2017). Biomist: A platform for
biomedical data lifecycle management of neuroimag-
ing cohorts. Frontiers in ICT, 3:35.
Alshboul, Y., Wang, Y., and Nepali, R. (2015). Big data
life cycle: threats and security model. In Americas
conference on information systems, pages 1–7.
Amadori, A., Altendeitering, M., and Otto, B. (2020). Chal-
lenges of data management in industry 4.0: A single
case study of the material retrieval process. In Interna-
tional Conference on Business Information Systems,
pages 379–390. Springer.
Bosch, J., Olsson, H. H., and Crnkovic, I. (2021). Engineer-
ing ai systems: a research agenda. In Artificial Intel-
ligence Paradigms for Smart Cyber-Physical Systems,
pages 1–19. IGI Global.
Bychkov, I., Demichev, A., Dubenskaya, J., Fedorov, O.,
Haungs, A., Heiss, A., Kang, D., Kazarina, Y., Ko-
rosteleva, E., Kostunin, D., Kryukov, A., Mikhailov,
A., Nguyen, M.-D., Polyakov, S., Postnikov, E., Shi-
garov, A., Shipilov, D., Streit, A., Tokareva, V.,
Wochele, D., Wochele, J., and Zhurov, D. (2018).
Russian–german astroparticle data life cycle initiative.
Data, 3(4):56.
Cao, H., Wachowicz, M., Renso, C., and Carlini, E. (2019).
Analytics everywhere: generating insights from the
internet of things. IEEE Access, 7:71749–71769.
Chen, J., Chen, Y., Du, X., Li, C., Lu, J., Zhao, S., and
Zhou, X. (2013). Big data challenge: a data man-
agement perspective. Frontiers of Computer Science,
7(2):157–164.
Cheng, X., Hu, C., Li, Y., Lin, W., and Zuo, H. (2013).
Data evolution analysis of virtual dataspace for man-
aging the big data lifecycle. In 2013 IEEE Interna-
tional Symposium on Parallel & Distributed Process-
ing, Workshops and Phd Forum, pages 2054–2063.
IEEE.
Christopherson, L., Mandal, A., Scott, E., and Baldin, I.
(2020). Toward a data lifecycle model for nsf large fa-
cilities. In Practice and Experience in Advanced Re-
search Computing, pages 168–175.
DAMA (2017). DAMA-DMBOK: Data Management Body
of Knowledge. Technics Publications.
Davenport, T. H. et al. (2006). Competing on analytics.
Harvard business review, 84(1):98.
El Arass, M., Ouazzani-Touhami, K., and Souissi, N.
(2020). Data life cycle: Towards a reference archi-
tecture. International Journal of Advanced Trends in
Computer Science and Engineering, 9(4):5645–5653.
El Arass, M., Tikito, I., and Souissi, N. (2017). Data life-
cycles analysis: towards intelligent cycle. In 2017 In-
telligent Systems and Computer Vision (ISCV), pages
1–8.
Elsayed, I., Muslimovic, A., and Brezany, P. (2008). In-
telligent dataspaces for e-science. In WSEAS Inter-
national Conference on Computational Intelligence,
Man-Machine Systems and Cybernetics, pages 94–
100.
Emam, I., Elyasigomari, V., Matthews, A., Pavlidis, S.,
Rocca-Serra, P., Guitton, F., Verbeeck, D., Grainger,
L., Borgogni, E., Del Giudice, G., Saqi, M., Houston,
P., and Guo, Y. (2019). Platformtm, a standards-based
data custodianship platform for translational medicine
research. Scientific data, page 149.
Fisher, T. (2009). The data asset: How smart companies
govern their data for business success, volume 24.
John Wiley & Sons.
Grunzke, R., Aguilera, A., Nagel, W. E., Krüger, J., Herres-
Pawlis, S., Hoffmann, A., and Gesing, S. (2015).
Managing complexity in distributed data life cycles
enhancing scientific discovery. In 2015 IEEE 11th In-
ternational Conference on e-Science, pages 371–380.
IEEE.
DATA 2021 - 10th International Conference on Data Science, Technology and Applications
174