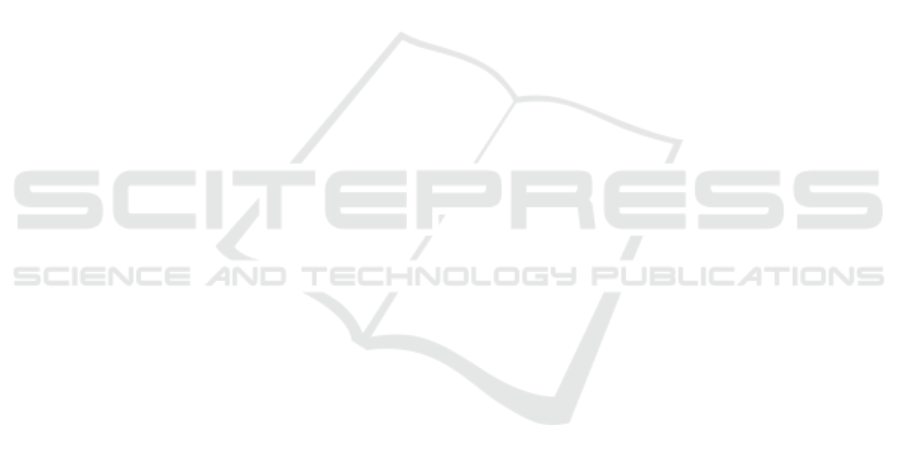
8 CONCLUSION AND FUTURE
WORK
In this paper, we addressed the problem of perform-
ing an efficient literature review considering the nu-
merous quantity of articles, papers and documents to
be retrieved and mined in, to filter them based on the
usefulness and retrieval of the other sources that refer
to or cited by the target document. The proposed so-
lution is able to identify the path of the linked sources
which can contribute to the research topic provided by
the researcher as an input in the form of a simplistic
sub-graph.
As the future works, we are currently working to
improve GRASPby:
• increasing the number of user study participants
to increase the robustness of the evaluations;
• a more in-depth analysis of the competitor solu-
tions;
• enhancing the way the sub-graph query is mapped
on the graph;
• considering more robust information retrieval
methods utilizing zone indexes and n-gram tok-
enizing;
• generating time-anchored graphs which show the
path through the years.
REFERENCES
Angles, R. and Gutierrez, C. (2008). Survey of graph
database models. CSUR.
Buttcher S., Clarke C. L. A., C. G. V. (2016). Information
Retrieval: Implementing and Evaluating Search En-
gines. MIT Press, Ltd.
Cesarini, M., Mercorio, F., Mezzanzanica, M., Moscato,
V., and Picariello, A. (2018). GraphDBLP Re-
leased: Querying the Computer Scientists Network as
a Graph. CEUR.
Dupret, G. (2011). Discounted cumulative gain and user
decision models. In Grossi, R., Sebastiani, F., and Sil-
vestri, F., editors, String Processing and Information
Retrieval, pages 2–13, Berlin, Heidelberg. Springer
Berlin Heidelberg.
Effendy, S., Jahja, I., and Yap, R. H. (2014). Relatedness
measures between conferences in computer science: a
preliminary study based on dblp. In WWW.
Francis, N., Green, A., Guagliardo, P., Libkin, L., Lin-
daaker, T., Marsault, V., Plantikow, S., Rydberg, M.,
Selmer, P., and Taylor, A. (2018). Cypher: An evolv-
ing query language for property graphs. In SIGMOD.
Hoang, D. T., Kaur, J., and Menczer, F. (2010). Crowd-
sourcing scholarly data. Proc. Web Science Con-
ference: Extending the Frontiers of Society On-Line
(WebSci).
Jarke, M., Neumann, B., Vassiliou, Y., and Wahlster, W.
(1989). Kbms requirements of knowledge-based sys-
tems. In Foundations of knowledge base management,
pages 381–394. Springer.
Khan, S., Liu, X., Shakil, K. A., and Alam, M. (2017). A
survey on scholarly data: From big data perspective.
IP&M.
Mercorio, F., Mezzanzanica, M., Moscato, V., and Sperl
`
ı,
G. (2019). A Tool for Researchers : Querying Big
Scholarly Data through Graph Databases. ECML
PKDD.
Ororbia, A. G., Wu, J., Khabsa, M., Williams, K., and Giles,
C. L. (2015). Big scholarly data in citeseerx: Informa-
tion extraction from the web. In WWW.
Robinson, I., Webber, J., and Eifrem, E. (2015). Graph
databases: new opportunities for connected data. ”
O’Reilly Media, Inc.”.
Sch
¨
afer, U. and Kiefer, B. (2011). Advances in deep parsing
of scholarly paper content. Lecture Notes in Computer
Science (including subseries Lecture Notes in Artifi-
cial Intelligence and Lecture Notes in Bioinformatics),
6699 LNCS:135–153.
Sch
¨
afer, U., Uszkoreit, H., Federmann, C., Marek, T., and
Zhang, Y. (2008). Extracting and querying relations
in scientific papers on language technology. LREC.
Sinha, A., Shen, Z., Song, Y., Ma, H., Eide, D., Hsu, B.-
J. P., and Wang, K. (2015). An overview of microsoft
academic service (mas) and applications. In WWW.
Tang, J., Zhang, J., Yao, L., Li, J., Zhang, L., and Su, Z.
(2008). Arnetminer: Extraction and mining of aca-
demic social networks. In SIGKDD.
Tran, D. H., Takeda, H., Kurakawa, K., and Tran, M. T.
(2012). Combining topic model and co-author net-
work for KAKEN and DBLP linking. Lecture Notes in
Computer Science (including subseries Lecture Notes
in Artificial Intelligence and Lecture Notes in Bioin-
formatics), 7198 LNAI.
Williams, K., Wu, J., Choudhury, S. R., Khabsa, M., and
Giles, C. L. (2014). Scholarly big data information
extraction and integration in the CiteSeerχ digital li-
brary. Proceedings - International Conference on
Data Engineering.
Xia, F., Wang, W., Bekele, T. M., and Liu, H. (2017). Big
scholarly data: A survey. IEEE Transactions on Big
Data.
Za, S. and Spagnoletti, P. (2013). Knowledge creation
processes in information systems and management:
Lessons from simulation studies. In Organizational
Change and Information Systems, pages 191–204.
Springer.
Zhao, D. and Strotmann, A. (2015). Analysis and visualiza-
tion of citation networks. Synthesis lectures on infor-
mation concepts, retrieval, and services, 7(1):1–207.
GRASP: Graph-based Mining of Scientific Papers
183