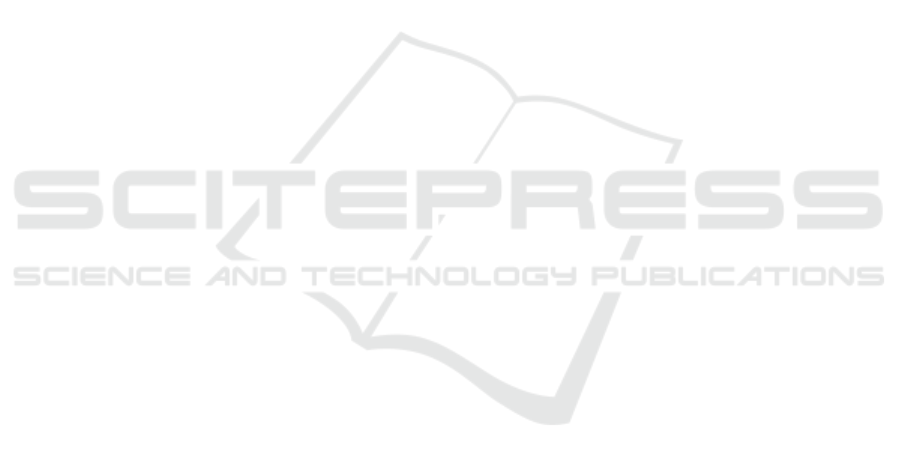
ACKNOWLEDGEMENTS
The work is carried out at Institute for Computer Sci-
ence and Control (SZTAKI), Hungary and the au-
thor would like to thank her colleague L
´
aszl
´
o Sp
´
or
´
as
for providing the infrastructure and technical sup-
port. This research was funded by Stipendium Hun-
garicum scholarship and China Scholarship Council.
The research was supported by the Hungarian Min-
istry of Innovation and Technology and the National
Research, Development and Innovation Office within
the framework of the National Lab for Autonomous
Systems.
REFERENCES
Barbedo, J. G. A. (2019). A review on the use of un-
manned aerial vehicles and imaging sensors for mon-
itoring and assessing plant stresses. Drones, 3:40.
Barekatain, M., Mart
´
ı, M., Shih, H., Murray, S., Nakayama,
K., Matsuo, Y., and Prendinger, H. (2017). Okutama-
action: An aerial view video dataset for concurrent
human action detection. In 2017 IEEE Conference on
Computer Vision and Pattern Recognition Workshops
(CVPRW), pages 2153–2160.
Bewley, A., Ge, Z., Ott, L., Ramos, F., and Upcroft, B.
(2016). Simple online and realtime tracking. 2016
IEEE International Conference on Image Processing
(ICIP), page 3464–3468.
Cao, Z., Simon, T., Wei, S.-E., and Sheikh, Y. (2017). Real-
time multi-person 2d pose estimation using part affin-
ity fields. In Proceedings of the IEEE Conference on
Computer Vision and Pattern Recognition (CVPR).
Carletti, V., Greco, A., Saggese, A., and Vento, M.
(2018). Multi-object tracking by flying cameras based
on a forward-backward interaction. IEEE Access,
6:43905–43919.
Carreira, J. and Zisserman, A. (2017). Quo vadis, action
recognition? a new model and the kinetics dataset.
In Proceedings of the IEEE Conference on Computer
Vision and Pattern Recognition (CVPR).
De Smedt, F., Hulens, D., and Goedeme, T. (2015). On-
board real-time tracking of pedestrians on a uav. In
Proceedings of the IEEE Conference on Computer Vi-
sion and Pattern Recognition (CVPR) Workshops.
Erdelj, M., Natalizio, E., Chowdhury, K. R., and Akyildiz,
I. F. (2017). Help from the sky: Leveraging uavs
for disaster management. IEEE Pervasive Computing,
16(1):24–32.
Gonc¸alves, J. and Henriques, R. (2015). Uav photogram-
metry for topographic monitoring of coastal areas. IS-
PRS Journal of Photogrammetry and Remote Sensing,
104:101 – 111.
Guo, G., Wang, H., Bell, D., Bi, Y., and Greer, K.
(2003). Knn model-based approach in classification.
In OTM Confederated International Conferences” On
the Move to Meaningful Internet Systems”, pages
986–996. Springer.
Hossain, S. and Lee, D.-J. (2019). Deep learning-based
real-time multiple-object detection and tracking from
aerial imagery via a flying robot with gpu-based em-
bedded devices. Sensors, 19:3371.
Hu, B. and Wang, J. (2018). Deep learning based hand ges-
ture recognition and uav flight controls.
Lawrence, C. (2021). reaktor/vzw-care-tf-pose-estimation.
Li, S. and Christensen, H. Wavetofly: Control a uav using
body gestures.
Lics
´
ar, A. and Szir
´
anyi, T. (2005). User-adaptive hand ges-
ture recognition system with interactive training. Im-
age and Vision Computing, 23(12):1102 – 1114.
Liu, W., Wang, Z., Liu, X., Zeng, N., Liu, Y., and Alsaadi,
F. E. (2017). A survey of deep neural network ar-
chitectures and their applications. Neurocomputing,
234:11 – 26.
Ma, Y., Liu, Y., Jin, R., Yuan, X., Sekha, R., Wilson, S.,
and Vaidyanathan, R. (2017). Hand gesture recogni-
tion with convolutional neural networks for the mul-
timodal uav control. In 2017 Workshop on Research,
Education and Development of Unmanned Aerial Sys-
tems (RED-UAS), pages 198–203.
Mavroforakis, M. E. and Theodoridis, S. (2006). A geo-
metric approach to support vector machine (svm) clas-
sification. IEEE Transactions on Neural Networks,
17(3):671–682.
Natarajan, K., Nguyen, T. D., and Mete, M. (2018). Hand
gesture controlled drones: An open source library.
In 2018 1st International Conference on Data Intel-
ligence and Security (ICDIS), pages 168–175.
Pal, M. (2005). Random forest classifier for remote sensing
classification. International journal of remote sensing,
26(1):217–222.
Park, K. and Ewing, R. (2017). The usability of unmanned
aerial vehicles (uavs) for measuring park-based physi-
cal activity. Landscape and Urban Planning, 167:157
– 164.
Perera, A. G., Law, Y. W., and Chahl, J. (2019). Drone-
action: An outdoor recorded drone video dataset for
action recognition. Drones, 3:82.
Perera, A. G., Wei Law, Y., and Chahl, J. (2018). Uav-
gesture: A dataset for uav control and gesture recog-
nition. In Proceedings of the European Conference on
Computer Vision (ECCV) Workshops.
Peschel, J. M. and Murphy, R. R. (2013). On the hu-
man–machine interaction of unmanned aerial system
mission specialists. IEEE Transactions on Human-
Machine Systems, 43(1):53–62.
Wojke, N., Bewley, A., and Paulus, D. (2017). Simple on-
line and realtime tracking with a deep association met-
ric. In 2017 IEEE International Conference on Image
Processing (ICIP), pages 3645–3649. IEEE.
Xia, G.-S., Bai, X., Ding, J., Zhu, Z., Belongie, S., Luo, J.,
Datcu, M., Pelillo, M., and Zhang, L. (2019). Dota:
A large-scale dataset for object detection in aerial im-
ages. arXiv:1711.10398 [cs].
Gesture Recognition for UAV-based Rescue Operation based on Deep Learning
187