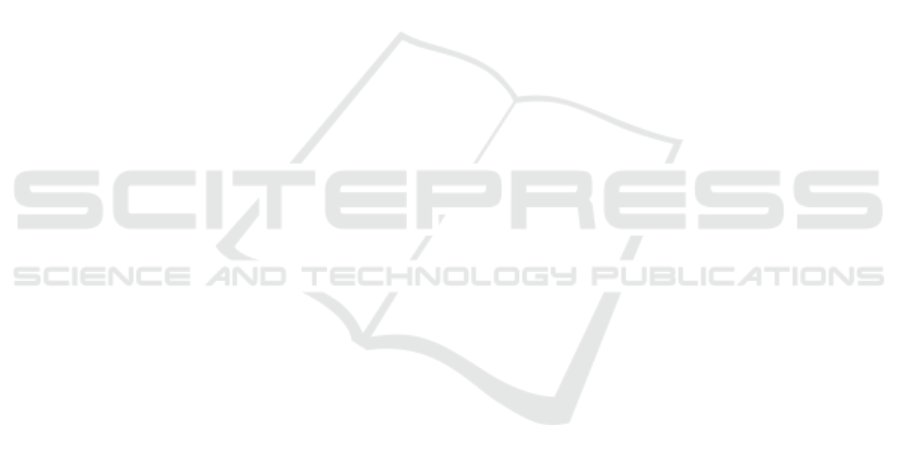
7 CONCLUSIONS AND
OUTLOOK
In this paper, we describe an approach for estimat-
ing the situation difficulty in driving scenarios by
combining important factors like traffic density, road
conditions, environmental factors, inside-vehicle dis-
tractions, driver capabilities and driver state. It is
based on a rule-based paradigm for difficulty esti-
mation which has several advantages. The rules are
reproducible and easily verifiable playing an impor-
tant role in safety relevant systems. Further, a holis-
tic architecture composed of various loosely-coupled
components is designed with the objective of allow-
ing the integration of heterogeneous data sources. Fi-
nally, we demonstrated our approach in three con-
crete situations where calculating DSDS including
personal characteristics could have a high impact on
the road safety. For simplicity, we have used dis-
crete values for the individual factors for a number of
difficulty dimensions that impact situation difficulty.
These could be extended to continuous-valued func-
tions, like a sigmoid function or could be learned if
sufficient training data is available.
As future work, we will work on including Fuzzy
Logic algorithms to provide classifications for a di-
mension’s observable difficulty levels. Next, we will
investigate adopting machine learning techniques re-
garding individual difficulty dimensions. Particularly
for cases where rules cannot be defined with sufficient
evidence and where enough data is available for train-
ing, learning based methods could be applied. An-
other direction of further analysis is related to the ma-
turity of sensors to determine the necessary parame-
ters and knowledge about their influence in the over-
all situation difficulty which can vary from domain to
domain. For example, much research has been done
for eye-gaze tracking and determining its influence in
driving, whereas in other areas like the quantification
and analysis of driver distraction by music, conver-
sation and others is less well known. How much in-
dividual capabilities and experience manifest them-
selves in day-to-day driving situations is subject of
current research (Kaber et al., 2012). But how these
are influenced by other factors like conversations, in-
fotainment or other distractions is unclear. Further-
more, we would like to learn individual capabilities,
how they change over time, and how they are influ-
enced by other factors.
REFERENCES
ASHA (2021). Loud noise dangers. https://www.
asha.org/public/hearing/loud-noise-dangers/.
Bazilinskyy, P., Petermeijer, S. M., Petrovych, V., Dodou,
D., and Winter, J. D. (2018). Take-over requests in
highly automated driving: A crowdsourcing survey
on auditory, vibrotactile, and visual displays. Trans-
portation Research Part F-traffic Psychology and Be-
haviour, 56:82–98.
Bengler, K., Dietmayer, K., F
¨
arber, B., Maurer, M., Stiller,
C., and Winner, H. (2014). Three decades of driver
assistance systems: Review and future perspectives.
IEEE Intell. Transport. Sys. Magazine, 6:6–22.
Berger, E., Neitzel, R., and Kladden, C.(2015).
Noise navigatortm sound level database with
over 1700 measurement values. https://multime
dia.3m.com/mws/media/888553O/noise-navigator-
sound-level-hearing-protection-database.pdf.
Beritelli, F., Capizzi, G., Sciuto, G. L., Napoli, C., and
Scaglione, F. (2018). Rainfall estimation based on the
intensity of the received signal in a lte/4g mobile ter-
minal by using a probabilistic neural network. IEEE
Access, 6:30865–30873.
Bier, L., Emele, M., Gut, K., Kulenovic, J., Rzany, D., Pe-
ter, M., and Abendroth, B. (2019). Preventing the risks
of monotony related fatigue while driving through
gamification. European Transport Research Review.
Braunagel, C., Geisler, D., Rosenstiel, W., and Kasneci, E.
(2017). Online recognition of driver-activity based on
visual scanpath classification. IEEE Intelligent Trans-
portation Systems Magazine, 9(4):23–36.
Fazio, P., Santamaria, A. F., Rango, F. D., Tropea, M., and
Serianni, A. (2016). A new application for analyz-
ing driving behaviour and environment characteriza-
tion in transportation systems based on a fuzzy logic
approach. In Unmanned Systems Technology XVIII,
volume 9837, pages 49 – 61. International Society for
Optics and Photonics, SPIE.
Heinzler, R., Schindler, P., Seekircher, J., Ritter, W., and
Stork, W. (2019). Weather influence and classification
with automotive lidar sensors. In 2019 IEEE Intelli-
gent Vehicles Symposium (IV), pages 1527–1534.
J
¨
agerbrand, A. and Sj
¨
obergh, J. (2016). Effects of weather
conditions, light conditions, and road lighting on ve-
hicle speed. SpringerPlus, 5.
Kaber, D., Zhang, Y., Jin, S., Mosaly, P., and Garner, M.
(2012). Effects of hazard exposure and roadway com-
plexity on young and older driver situation awareness
and performance. Transportation Research Part F-
traffic Psychology and Behaviour, 15:600–611.
Kita, H. (2000). Level-of-service measure of road traffic
based on the driver’s perception. Transportation re-
search circular, pages 53–62.
Li, B., Song, D., Li, H., Pike, A., and Carlson, P. (2018).
Lane marking quality assessment for autonomous
driving. In 2018 IEEE/RSJ International Conference
on Intelligent Robots and Systems (IROS).
Ma, W. and Qian, S. (2019). High-resolution traffic sensing
with autonomous vehicles.
Towards a Rule-based Approach for Estimating the Situation Difficulty in Driving Scenarios
729