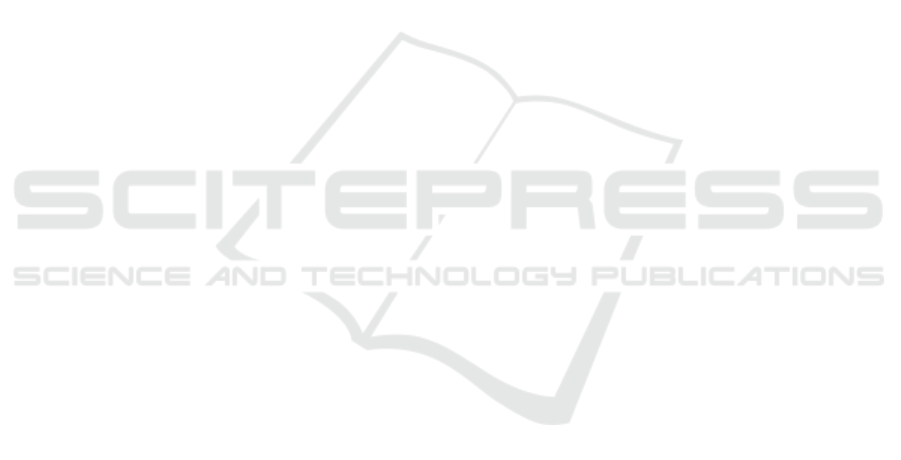
words, and linguistic summarization should provide
the mean to achieve the aforementioned objective.
ACKNOWLEDGEMENT
The authors would like to thank the Metropolitanraum
Z
¨
urich association and cividi GmbH for their support
in the development of this project.
REFERENCES
Albawi, S., Mohammed, T. A., and Al-Zawi, S. (2017).
Understanding of a convolutional neural network. In
2017 International Conference on Engineering and
Technology (ICET), pages 1–6. IEEE.
Barbier, G., Zafarani, R., Gao, H., Fung, G., and Liu,
H. (2012). Maximizing benefits from crowdsourced
data. Computational and Mathematical Organization
Theory, 18(3):257–279.
Chopra, S., Hadsell, R., and LeCun, Y. (2005). Learning
a similarity metric discriminatively, with application
to face verification. In 2005 IEEE Computer
Society Conference on Computer Vision and Pattern
Recognition (CVPR’05), volume 1, pages 539–546.
IEEE.
Deng, J., Dong, W., Socher, R., Li, L.-J., Li, K., and Fei-Fei,
L. (2009). Imagenet: A large-scale hierarchical image
database. In 2009 IEEE conference on computer
vision and pattern recognition, pages 248–255. Ieee.
Dilks, D. D., Baker, C. I., Liu, Y., and Kanwisher, N.
(2009). “referred visual sensations”: Rapid perceptual
elongation after visual cortical deprivation. Journal of
Neuroscience, 29(28):8960–8964.
Dubey, A., Naik, N., Parikh, D., Raskar, R., and
Hidalgo, C. A. (2016). Deep learning the city:
Quantifying urban perception at a global scale. In
European conference on computer vision, pages 196–
212. Springer.
Dunn, J. C. (1973). A fuzzy relative of the isodata process
and its use in detecting compact well-separated
clusters. Journal of Cybernetics, 3(3):32–57.
Estell
´
es-Arolas, E. and Gonz
´
alez-Ladr
´
on-De-Guevara, F.
(2012). Towards an integrated crowdsourcing
definition. Journal of Information science, 38(2):189–
200.
Gau, J. M. and Pratt, T. C. (2010). Revisiting broken
windows theory: Examining the sources of the
discriminant validity of perceived disorder and crime.
Journal of criminal justice, 38(4):758–766.
Goldhagen, S. W. and Gallo, A. (2017). Welcome to your
world: How the built environment shapes our lives.
Harper New York.
Herbrich, R., Minka, T., and Graepel, T. (2007).
Trueskill(tm): A bayesian skill rating system. In
Advances in Neural Information Processing Systems
20, pages 569–576. MIT Press.
Hudec, M., Vu
ˇ
ceti
´
c, M., and
ˇ
Cerm
´
akov
´
a, I. (2020). The
synergy of linguistic summaries, fuzzy functional
dependencies and land coverings for augmenting
informativeness in smart cities. In 2020 28th
Telecommunications Forum (TELFOR), pages 1–4.
IEEE.
Ilic, L., Sawada, M., and Zarzelli, A. (2019). Deep
mapping gentrification in a large canadian city using
deep learning and google street view. PLOS ONE,
14(3):1–21.
Lanier, J. (2003). Why gordian software has convinced me
to believe in the reality of cats and apples. https://
www.edge.org. Visited on Feb. 2021.
LeCun, Y., Bengio, Y., and Hinton, G. (2015). Deep
learning. nature, 521(7553):436–444.
Liu, L., Silva, E. A., Wu, C., and Wang, H. (2017). A
machine learning-based method for the large-scale
evaluation of the qualities of the urban environment.
Computers, Environment and Urban Systems, 65:113–
125.
Lynch, K. (1960). The image of the city, volume 11. MIT
press.
O’Shea, K. and Nash, R. (2015). An introduction
to convolutional neural networks. arXiv preprint
arXiv:1511.08458.
Salesses, P., Schechtner, K., and Hidalgo, C. A. (2013).
The collaborative image of the city: mapping
the inequality of urban perception. PloS one,
8(7):e68400.
Seresinhe, C. I., Preis, T., and Moat, H. S. (2017). Using
deep learning to quantify the beauty of outdoor places.
Royal Society open science, 4(7):170170.
Simonyan, K. and Zisserman, A. (2014). Very
deep convolutional networks for large-scale image
recognition. arXiv preprint arXiv:1409.1556.
Srivastava, N., Hinton, G., Krizhevsky, A., Sutskever, I.,
and Salakhutdinov, R. (2014). Dropout: a simple
way to prevent neural networks from overfitting. The
journal of machine learning research, 15(1):1929–
1958.
Wang, S.-C. (2003). Artificial Neural Network, pages 81–
100. Springer US, Boston, MA.
Zhang, R., Isola, P., Efros, A. A., Shechtman, E., and Wang,
O. (2018). The unreasonable effectiveness of deep
features as a perceptual metric. In Proceedings of
the IEEE conference on computer vision and pattern
recognition, pages 586–595.
ICEIS 2021 - 23rd International Conference on Enterprise Information Systems
818