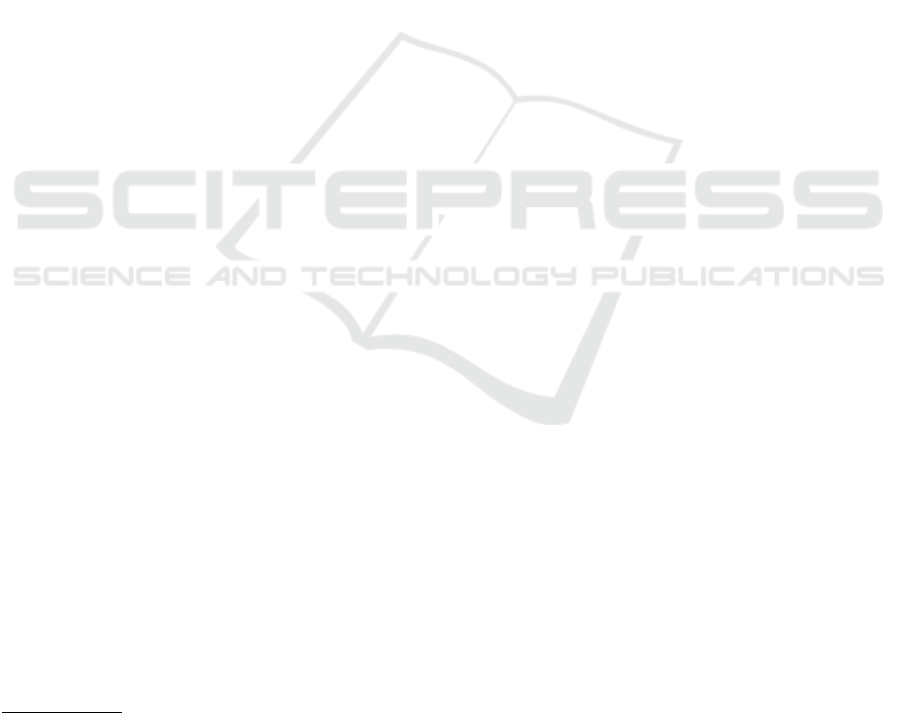
riod, also exposing the operators in charge themselves
to a high risk and requiring to sanitize frequently tools
and equipments.
So, thermal cameras have offered a potential miti-
gation facility to these latter problems, by providing a
contact-less mean for detecting people with fever-like
symptoms in high-traffic areas.
Anyway, in our experience, when also compared
to other experiences
6
, the evidence suggests ther-
mal cameras are still far from being a complete au-
tonomous solution, because several aspects, among
which a still limited accuracy, a high bias from a
wrong set up, the inability to recognise body parts,
and, finally raising data privacy concerns. The same
problem of data privacy was arisen with the introduc-
tion on smart app for implementing the back tracking
of infected or potentially infected people
7
.
Beyond privacy concerns, accuracy of measure-
ments can’t be neglected, because it negatively affects
the monitoring system, both when infected person
are not detected (incorrect false negative) and when
healthy people are detected as infected (incorrect false
positive); the first one produces free undisturbed cir-
culation of infected people and increase the spreading
of contagion; on the other hand, the second one can
lead the healthcare system to collapse, when starts re-
covering procedures needlessly.
High Accuracy performed by thermal cameras and
any other system, requires a precise and continuous
calibration, because the environmental conditions can
suddenly and quickly change. Furthermore, a fre-
quent re-tuning of such kind of systems requires a not
trivial effort by operators and organizations that have
to manage the monitoring systems.
Artificial intelligence and advanced machine
learning techniques can mitigate this aspect by pro-
viding mechanisms for improving accuracy of mea-
surements by enabling automatic tuning of systems,
according to preliminary learning process and an on
line inference process. In this work, we have pro-
posed a complete system for building a monitoring
system based on thermal cameras that exploit ma-
chine learning techniques to induce a different behav-
ior in the cameras, according to different environment
condition. we provided the full details about software
and hardware platform implemented for performing
preliminary experiments.
The lesson we learned by the experiments we per-
formed is that thermal cameras technology is not yet
6
https://theconversation.com/are-thermal-cameras-a-
magic-bullet-for-covid-19-fever-detection-theres-not-
enough-evidence-to-know-139377
7
https://www.webmd.com/lung/news/20200928/
privacy-concerns-hindering-digital-contact-tracing
mature enough to guarantee monitoring with a high
level of reliability on its own. The current technology
included in the thermal camera does not include, for
example, face recognition and, consequently, is un-
able to discard those measurements deriving from in-
significant parts (head, shoulders, ground and other
hot objects, etc.).
In the future, by combining thermal measurements
with more complex intelligence, as the one provided
by image recognition and processing, it might be
thought to indicate the position of the eyes to the ther-
mal camera for a more reliable measurement of body
temperature.
REFERENCES
Bardou, M., Seng, P., Meddeb, L., Gaudart, J., Honnorat,
E., and Stein, A. (2016). Modern approach to infec-
tious disease management using infrared thermal cam-
era scanning for fever in healthcare settings. Journal
of Infection.
Chiang, M.-F., Lin, P.-W., Lin, L.-F., Chiou, H.-Y., Chien,
C.-W., Chu, S.-F., and Chiu, W.-T. (2008). Mass
screening of suspected febrile patients with remote-
sensing infrared thermography: alarm temperature
and optimal distance. Journal of the Formosan Medi-
cal association, 107(12):937–944.
Chiu, W., Lin, P., Chiou, H., Lee, W., Lee, C., Yang, Y., Lee,
H., Hsieh, M., Hu, C., Ho, Y., et al. (2005). Infrared
thermography to mass-screen suspected sars patients
with fever. Asia Pacific Journal of Public Health,
17(1):26–28.
Ghassemi, P., Pfefer, T. J., Casamento, J. P., Simpson, R.,
and Wang, Q. (2018). Best practices for standard-
ized performance testing of infrared thermographs in-
tended for fever screening. PloS one, 13(9):e0203302.
Kang, J. K., Hoang, T. M., and Park, K. R. (2019). Person
re-identification between visible and thermal camera
images based on deep residual cnn using single input.
IEEE Access, 7:57972–57984.
Leach, K. C., Ellsworth, M. G., Ostrosky, L. Z., Bell, C. S.,
Masters, K., Calhoun, J., Ferguson, L., Distefano, S.,
and Chang, M. L. (2021). Evaluation of a telethermo-
graphic system for temperature screening at a large
tertiary-care referral hospital during the coronavirus
disease 2019 (covid-19) pandemic. Infection Control
& Hospital Epidemiology, 42(1):103–105.
Mercer, J. B. and Ring, E. F. J. (2009). Fever screening
and infrared thermal imaging: concerns and guide-
lines. Thermology International, 19(3):67–69.
Selent, M. U., Molinari, N. M., Baxter, A., Nguyen, A. V.,
Siegelson, H., Brown, C. M., Plummer, A., Higgins,
A., Podolsky, S., Spandorfer, P., et al. (2013). Mass
screening for fever in children: a comparison of 3 in-
frared thermal detection systems. Pediatric emergency
care, 29(3):305–313.
AI4EIoTs 2021 - Special Session on Artificial Intelligence for Emerging IoT Systems: Open Challenges and Novel Perspectives
352