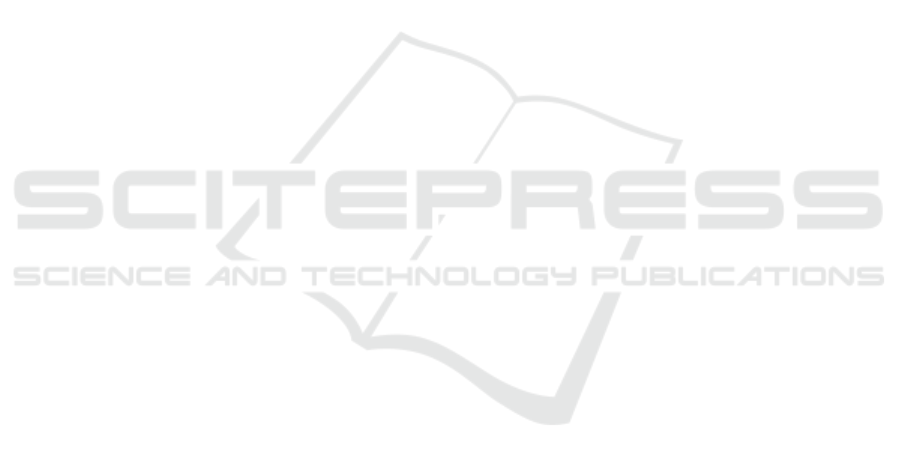
it is related to all the human activities that emit in the
atmosphere. Looking at a micro-scale area, new fac-
tors can be added: for example, the city architecture
can influence the wind direction and speed resulting
in different dispersion scenario or the implementation
of a green area can decrease local contamination.
In order to restrain the continuous pollution and to
try restoring some area to a better status, worldwide
regulations have been issued: most of them imposed
concentration limits both for outdoor air regarding
pollutants like carbon monoxide, BTEX (Benzene
- Toluene - Ethylbenzene - Xylene), nitrogen ox-
ides, particular matter, and limit for specific emitting
sources as industrial plants and human activities with
the use of organic solvents. The overall strategy is to
limit the emitting source where and when it is possi-
ble, and to check the air quality as result of the previ-
ous described factor. With the collected data, logged
from stationary and mobile station, there is the pos-
sibility to assess the air quality by the use of some
indicators based on the detected concentration of spe-
cific pollutant. A similar indicator is used in Europe,
namely European Air Quality Index (EAQI), as estab-
lished by 2008/50/CE directive. The hourly index is
based on concentration values for up to five key pollu-
tants and it reflects the potential impact of air quality
on health, driven by the pollutant for which concen-
trations are poorest due to associated health impacts.
The data are collected by stationary stations managed
by local authorities.
In Italy, the transposition of the European di-
rective took place with the enactment of law D.lgs.
155/2010 that has established a unified regulatory
framework for the assessment and management of
ambient air quality. Regions are assigned the respon-
sibility to assess this quality, to classify the regional
territory into zones and agglomerations, and to draw
up plans and programs to maintain ambient air quality
where it is good and to improve it in other cases. The
national law imposes limits to outdoor pollutants con-
centration and, since the private transport sector has
been identified as the major contributor to city pollu-
tion, in case of exceeding daily limits, the city admin-
istration restricts the access for private transport.
In this scenario, the implementation of forecasting
modeling systems have become increasingly impor-
tant in order to understand the future impacts of the
human activities and to manage local areas. Forecast-
ing can both be applied to the new emitting sources
in order to understand their relative impact on local
air, and directly to outdoor air quality to understand
its development. It is clear that in the first case the
problem resolution is easier since all the factors that
affect the final result are well known (characteristics
of the emitting source, pollutant concentration, plant
layout, etc.). In the second case, the factors that took
part in the game are various and not always so well
defined: indeed, the local impact detected by station-
ary stations is due to a series of events such as par-
ticular wind direction, local traffic, presence of a new
apartment blocks and so on. Hence, in the situation of
a micro-scale forecasting, the boundary between the
influence classes for the air quality is very blurred. To
help solve this problem, the use of machine learning
techniques seems to be a promising practice.
In the last years, many scholars have studied the
implementation of forecasting modeling with ma-
chine learning: the results may significantly vary, de-
pending on the used dataset and the implementation
made. The machine learning help is based on the as-
sumption of a black box mechanism for the air qual-
ity: the forecast is essentially based on the ’training’
on a specific dataset, which results in the extrapola-
tion of a statistical set of rules that can be applied to
the newly collected data. Globally, the shown trends
indicate an improvement in the forecast on an ex-
tended area, such as a region, or at national level, with
high level datasets. The forecast buffer time can also
vary according to the used mechanism.
In this paper, an application of a forecasting mod-
eling approach implemented by a machine learning
based technique is presented for an Italian city where
air quality is assessed by means of stationary stations
controlled by local authorities according to D.lgs.
155/2010. The aim of this research is to understand
if this application can lead to a good forecast on a
focused area with a few analyzing stations and local
weather stations in order to better manage the area be-
fore the limits imposed are exceeded. The main origi-
nal contribution is the application of this kind of anal-
ysis on combined official pollution and weather data
about Campania region: at the best of our knowledge,
no such analysis is available in literature. In addition,
at the moment as per practice the data collected from
the station are firstly validated by a third part before
they are used for forecasting purposes. Indeed, this
quality check and assurance (QC/QA) is an essential
phase and it is usually handmade by few technicians.
For these reasons, it could easily be affected by errors
and hence data loss. Consequently, for this research
we only used raw data in order to check how they per-
form without any preliminary screening.
After this section, the paper is organized as fol-
lows: next section presents related work, and a brief
background on this field is summarized; then the
case study and the used dataset are described; sub-
sequently, the methodology used to develop the fore-
casting model by means of machine learning; results
Applying Machine Learning to Weather and Pollution Data Analysis for a Better Management of Local Areas: The Case of Napoli, Italy
355