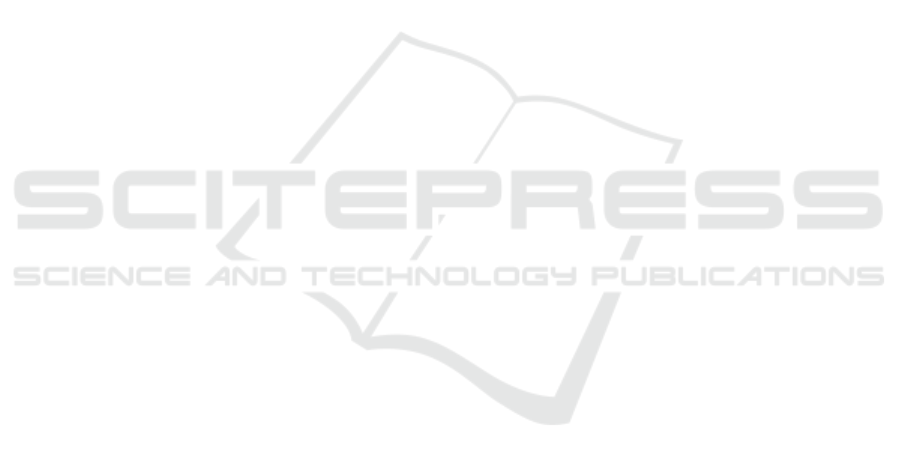
sessed through the noise density variation analysis.
Thereby, the glue level estimation accuracy is eval-
uated regarding different noise densities. In Figure
9 the regression models are applied in two different
noises, Salt and Pepper and Gaussian noises, regard-
ing three noise densities, 0.001, 0.005 and 0.01. In
Figures 9a and 9b the RMSE behaviors are presented
regarding the Salt and Pepper and Gaussian noises,
respectively. From Figures 9a and 9b it is possible
to verify the proposed approach effectiveness, even in
different noise densities.
5 CONCLUSION
In this paper, we addressed the problem of automatic
visual inspection for glue level control. Unlike other
state-of-the-art approaches, our method continuously
monitors the glue level during the glue injection pro-
cess in PCB manufacturing, aggregating more infor-
mation to production process.
Real-world and simulated experiments involving
different regression models and simulated noise types
have shown that the obtained glue level predictions
are reliable and accurate considering the obtained re-
sults. Additionally, the proposed approach demon-
strates robustness, even in presence of noise during
image acquisition, and feasibility to real time indus-
trial application, once the experiments were carried
out in real time scenario.
As future work, we intend to combine different
predictive methods to improve the glue level estima-
tion accuracy. We also intend to concentrate efforts
to extend the automatic visual inspection approach to
tackle other types of problems related to PCB manu-
facturing. The volume and position control of injected
glue is also a relevant problem we intend to investi-
gate and incorporate in production lines.
ACKNOWLEDGMENT
This work was developed with support from Cal-
Comp Eletronic through R&D project in Institute of
Exact Sciences and Technology of Federal University
of Amazonas, Itacoatiara, Amazonas.
REFERENCES
Abdul Rahman, N. N., Mohd Saad, N., and Abdullah, A. R.
(2018). Shape and level bottles detection using lo-
cal standard deviation and hough transform. Interna-
tional Journal of Electrical and Computer Engineer-
ing IJECE, 8:5032.
Breiman, L. (2001). Random forests. Machine Learning,
45(1):5–32.
Dong, X., Taylor, C. J., and Cootes, T. F. (2020). A
random forest-based automatic inspection system for
aerospace welds in x-ray images. IEEE Transactions
on Automation Science and Engineering, pages 1–14.
Forsyth, D. (2019). Applied Machine Learning. Springer
International Publishing.
Gonzalez, R. and Woods, R. (2017). Digital image process-
ing, 4th edn. isbn: 9780133356724.
Otsu, N. (1979). A threshold selection method from gray-
level histograms. IEEE Transactions on Systems,
Man, and Cybernetics, 9(1):62–66.
Rahman, N. N. S. A., Saad, N. M., Abdullah, A. R., and
Ahmat, N. (2019). A Review of Vision based De-
fect Detection using Image Processing Techniques for
Beverage Manufacturing Industry. Jurnal Teknologi,
81(3).
Remmach, H., Touahni, R., and Sbihi, A. (2020). Spatial-
color mode detection in uv pairwise projection of the
cie luv color space. In 2020 IEEE International Con-
ference on Informatics, IoT, and Enabling Technolo-
gies (ICIoT), pages 383–388.
Rocha, C. S., Menezes, M. A. G., and Oliveira, F. G.
(2016). Detecc¸
˜
ao autom
´
atica de microcomponentes
smt ausentes em placas de circuito impresso. In
Menotti, D. and Miranda, P., editors, Workshop on In-
dustry Applications (WIA) in the 29th Conference on
Graphics, Patterns and Images (SIBGRAPI’16), vol-
ume 1, S
˜
ao Jos
´
e dos Campos, SP, Brazil.
Saad, N. M., Rahman, N. N. A., Abdullah, A. R., and
Rahim, N. A. (2017). Real-time product quality in-
spection monitoring system using quadratic distance
and level classifier. Journal of Telecommunication,
Electronic and Computer Engineering, 9:57–62.
Suzuki, S. and be, K. (1985). Topological structural anal-
ysis of digitized binary images by border following.
Computer Vision, Graphics, and Image Processing,
30(1):32–46.
Thielen, N., Werner, D., Schmidt, K., Seidel, R., Reinhardt,
A., and Franke, J. (2020). A machine learning based
approach to detect false calls in smt manufacturing. In
2020 43rd International Spring Seminar on Electron-
ics Technology (ISSE), pages 1–6.
Vafeiadis, T., Dimitriou, N., Ioannidis, D., Wotherspoon, T.,
Tinker, G., and Tzovaras, D. (2018). A framework for
inspection of dies attachment on PCB utilizing ma-
chine learning techniques. Journal of Management
Analytics, 5(2):81–94.
Zhang, H., Li, X., Zhong, H., Yang, Y., Wu, Q. M. J., Ge,
J., and Wang, Y. (2018). Automated machine vision
system for liquid particle inspection of pharmaceutical
injection. IEEE Transactions on Instrumentation and
Measurement, 67(6):1278–1297.
Zhou, D., Zhang, G., and Guo, Y. (2017). Detecting multi-
interface from oil-water separation image based on
difference statistics method. In 2017 IEEE Inter-
national Conference on Smart Cloud (SmartCloud).
IEEE.
ICINCO 2021 - 18th International Conference on Informatics in Control, Automation and Robotics
738