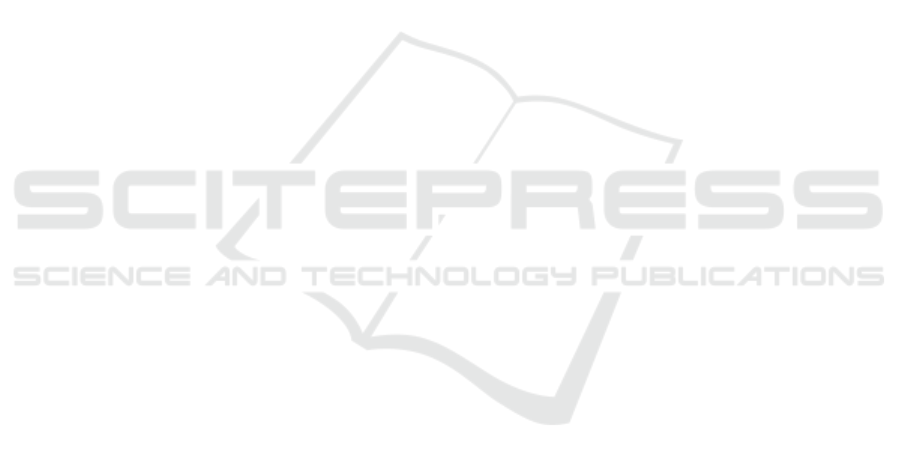
tive and explainable detection of android malware in
your pocket. In Ndss, volume 14, pages 23–26.
Azab, A. and Khasawneh, M. (2020). Msic: mal-
ware spectrogram image classification. IEEE Access,
8:102007–102021.
Bakhshinejad, N. and Hamzeh, A. (2017). A new compres-
sion based method for android malware detection us-
ing opcodes. In 2017 Artificial Intelligence and Signal
Processing Conference (AISP), pages 256–261. IEEE.
Canfora, G., Martinelli, F., Mercaldo, F., Nardone, V., San-
tone, A., and Visaggio, C. A. (2018). Leila: formal
tool for identifying mobile malicious behaviour. IEEE
Transactions on Software Engineering, 45(12):1230–
1252.
Enck, W., Gilbert, P., Han, S., Tendulkar, V., Chun, B.-
G., Cox, L. P., Jung, J., McDaniel, P., and Sheth,
A. N. (2014). Taintdroid: an information-flow track-
ing system for realtime privacy monitoring on smart-
phones. ACM Transactions on Computer Systems
(TOCS), 32(2):1–29.
Farrokhmanesh, M. and Hamzeh, A. (2019). Music classifi-
cation as a new approach for malware detection. Jour-
nal of Computer Virology and Hacking Techniques,
15(2):77–96.
Iadarola, G., Martinelli, F., Mercaldo, F., and Santone, A.
(2020). Evaluating deep learning classification relia-
bility in android malware family detection. In 2020
IEEE International Symposium on Software Relia-
bility Engineering Workshops (ISSREW), pages 255–
260. IEEE.
Iadarola, G., Martinelli, F., Mercaldo, F., and Santone, A.
(2021). Towards an interpretable deep learning model
for mobile malware detection and family identifica-
tion. Computers & Security, page 102198.
Jerome, Q., Allix, K., State, R., and Engel, T. (2014). Us-
ing opcode-sequences to detect malicious android ap-
plications. In 2014 IEEE International Conference on
Communications (ICC), pages 914–919.
Kim, H. M., Song, H. M., Seo, J. W., and Kim, H. K.
(2018). Andro-simnet: Android malware family clas-
sification using social network analysis. In 2018 16th
Annual Conference on Privacy, Security and Trust
(PST), pages 1–8. IEEE.
Michael, S., Florian, E., Thomas, S., Felix, C. F., and Hoff-
mann, J. (2013). Mobilesandbox: Looking deeper into
android applications. In Proceedings of the 28th In-
ternational ACM Symposium on Applied Computing
(SAC).
Nataraj, L., Karthikeyan, S., Jacob, G., and Manjunath,
B. S. (2011). Malware images: visualization and auto-
matic classification. In Proceedings of the 8th interna-
tional symposium on visualization for cyber security,
pages 1–7.
Nguyen, T., Mcdonald, J., Glisson, W., and Andel, T.
(2020). Detecting repackaged android applications us-
ing perceptual hashing. In Proceedings of the 53rd
Hawaii International Conference on System Sciences.
Popli, N. K. and Girdhar, A. (2019). Behavioural analy-
sis of recent ransomwares and prediction of future at-
tacks by polymorphic and metamorphic ransomware.
In Computational Intelligence: Theories, Applica-
tions and Future Directions-Volume II, pages 65–80.
Springer.
Vasan, D., Alazab, M., Wassan, S., Naeem, H., Safaei,
B., and Zheng, Q. (2020). Imcfn: Image-based
malware classification using fine-tuned convolutional
neural network architecture. Computer Networks,
171:107138.
Zhou, Y. and Jiang, X. (2012). Dissecting android mal-
ware: Characterization and evolution. In Proceed-
ings of 33rd IEEE Symposium on Security and Privacy
(Oakland 2012).
SECRYPT 2021 - 18th International Conference on Security and Cryptography
486