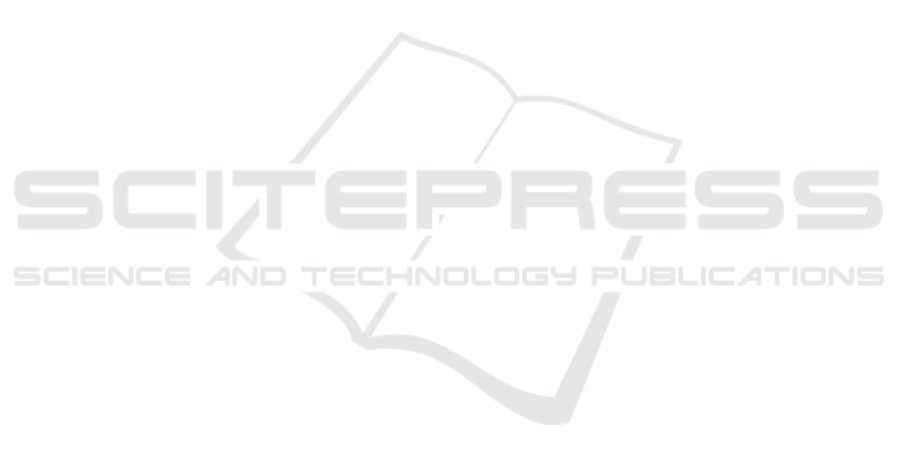
5 CONCLUSION AND OUTLOOK
In this paper, we presented DQ-MeeRKat, a tool
that implements a reference-data-profile-annotated
KG for automated DQ monitoring. We demonstrated
its applicability to (i) automatically learn RDPs for
heterogeneous data sources, and (ii) to calculate new
DPs on-the-fly to verify that newly inserted or up-
dated data continues to conform to the constraints
stored in the RDPs. We are currently working on and
planning the following extensions:
• A UI to enable RDP refinement for domain ex-
perts (cf. suggestion (3) by Tributech).
• As already incorporated in our vision, DQ-
MeeRKat aims to actively support the storage of
different versions per RDP.
• The overall vision for DQ-MeeRKat is to create
a comprehensive “AI-based surveillance state”,
which is capable of characterizing various kinds
of data to detect drifts and anomalies in DQ at the
earliest possible stage. Thus, we are going to en-
hance our RDPs with more complex statistics and
ML models, which are able to capture patterns in
the data (cf. suggestion (1) by Tributech). We will
focus on white-box models only since it is crucial
that statements about DQ are always explainable.
ACKNOWLEDGEMENTS
The research reported in this paper has been funded
by BMK, BMDW, and the Province of Upper Austria
in the frame of the COMET Programme managed by
FFG. The authors thank Patrick Lamplmair of Trib-
utech Solutions GmbH for providing the data streams.
REFERENCES
Abadi, D., Ailamaki, A., Andersen, D., Bailis, P., Balazin-
ska, M., Bernstein, P., Boncz, P., Chaudhuri, S., Che-
ung, A., Doan, A., et al. (2019). The Seattle Re-
port on Database Research. ACM SIGMOD Record,
48(4):44–53.
Abedjan, Z., Golab, L., Naumann, F., and Papenbrock, T.
(2019). Data Profiling. Synthesis Lectures on Data
Management, 10(4):1–154.
Bronselaer, A., De Mol, R., and De Tr
´
e, G. (2018). A
Measure-theoretic Foundation for Data Quality. IEEE
Transactions on Fuzzy Systems, 26(2):627–639.
Ehrlinger, L., Rusz, E., and W
¨
oß, W. (2019). A Survey
of Data Quality Measurement and Monitoring Tools.
CoRR, abs/1907.08138:1–30.
Ehrlinger, L., Werth, B., and W
¨
oß, W. (2018). Automated
Continuous Data Quality Measurement with QuaIIe.
International Journal on Advances in Software, 11(3
& 4):400–417.
Ehrlinger, L. and W
¨
oß, W. (2017). Automated Data Qual-
ity Monitoring. In Proceedings of the 22nd MIT In-
ternational Conference on Information Quality (MIT
ICIQ), pages 15.1–15.9, UA Little Rock, AR, USA.
Fischer, L., Ehrlinger, L., Geist, V., Ramler, R., Sobieczky,
F., Zellinger, W., Brunner, D., Kumar, M., and Moser,
B. (2021). AI System Engineering–Key Challenges
and Lessons Learned. Machine Learning and Knowl-
edge Extraction, 3(1):56–83.
Giebler, C., Gr
¨
oger, C., Hoos, E., Schwarz, H., and
Mitschang, B. (2019). Leveraging the Data Lake:
Current State and Challenges. In Big Data Analyt-
ics and Knowledge Discovery, pages 179–188, Cham.
Springer International Publishing.
Heidari, A., McGrath, J., Ilyas, I. F., and Rekatsinas, T.
(2019). Holodetect: Few-Shot Learning for Error De-
tection. In Intl. Conf. on Management of Data (SIG-
MOD’19), pages 829–846, New York, USA, ACM.
Heinrich, B., Hristova, D., Klier, M., Schiller, A., and
Szubartowicz, M. (2018). Requirements for Data
Quality Metrics. Journal of Data and Information
Quality, 9(2):12:1–12:32.
Hogan, A., Brickley, D., Gutierrez, C., Polleres, A., ,
and Zimmerman, A.(2019). (Re)Defining Knowl-
edge Graphs. In Knowledge Graphs: New Directions
for Knowledge Representation on the Semantic Web
(Dagstuhl Seminar 18371), pages 74–79, Germany.
Kiryakov, A., Ognyanov, D., and Manov, D. (2005).
OWLIM – A Pragmatic Semantic Repository for
OWL. In International Conf. on Web Information Sys-
tems Engineering – WISE 2005 Workshops, vol.3807,
pages 182–192. Springer.
Ledvinka, M. and K
ˇ
remen, P. (2019). A Comparison
of Object-Triple Mapping Libraries. Semantic Web,
pages 1–43. Preprint.
Meng, X., Bradley, J., Yavuz, B., Sparks, E., Venkataraman,
S., Liu, D., Freeman, J., Tsai, D., Amde, M., Owen,
S., et al. (2016). Mllib: Machine Learning in Apache
Spark. The Journal of Machine Learning Research,
17(1):1235–1241.
Naqvi, S. N. Z., Yfantidou, S., and Zim
´
anyi, E. (2017).
Time Series Databases and InfluxDB. Technical re-
port, Universit
´
e Libre de Bruxelles.
Pipino, L., Wang, R., Kopcso, D., and Rybolt, W. (2005).
Developing Measurement Scales for Data-Quality Di-
mensions. Information Quality, 1:37–52.
Scannapieco, M. and Catarci, T. (2002). Data Quality Under
a Computer Science Perspective. Archivi & Computer,
2:1–15.
Sebastian-Coleman, L. (2013). Measuring Data Quality for
Ongoing Improvement: A Data Quality Assessment
Framework. Elsevier, Waltham, MA, USA.
Stonebraker, M. and Ilyas, I. F. (2018). Data Integration:
The Current Status and the Way Forward. Bulletin of
the IEEE Computer Society Technical Committee on
Data Engineering, 41(2):3–9.
Wang, R. Y. and Strong, D. (1996). Beyond Accuracy:
What Data Quality Means to Data Consumers. Jour-
nal of Management Information Systems, 12(4):5–33.
DATA 2021 - 10th International Conference on Data Science, Technology and Applications
222