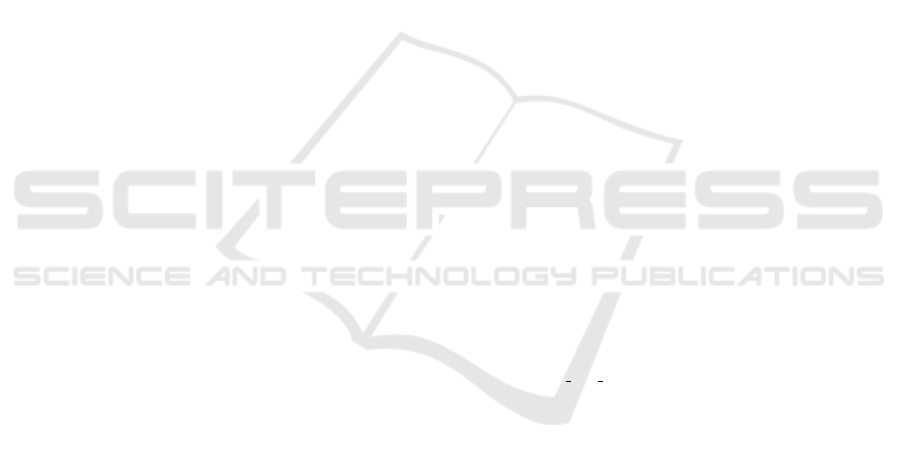
simulation, and it is re-assembled for visualization
and further numerical analysis. A simple 2D graphi-
cal representation of the assembled complexes is also
generated, where one can track the ssDNAs within
the complex as well as all the binding interactions.
Different from other computational modeling
frameworks for DNA strand assembly, we can modify
some of the systems parameters, such as the temper-
ature of the system, during the system simulation.
Thus, we can model also an entire annealing process
for the formation of a DNA structure. The underlining
computational modeling engine used by VDNA-Lab,
namely NFsim, allows also for other parameters to be
adjusted mid-simulation: e.g., we can define specific
binding/un-binding kinetic rates for each different
length-3 subsequence. Currently, VDNA-Lab does
not have these features implemented.
ACKNOWLEDGEMENTS
This work was partially supported by the Academy of
Finland, project 311371/2017, and by the Romanian
National Authority for Scientific Research and Inno-
vation, PED grant 2391.
REFERENCES
Ariga, K. and et al. (2008). Challenges and breakthroughs
in recent research on self-assembly. Science and Tech-
nology of Advanced Materials, 9(1):014109.
Blinov, M. L. and et al. (2004). BioNetGen: software for
rule-based modeling of signal transduction based on
the interactions of molecular domains. Bioinformat-
ics, 20(17):3289–3291.
Danos, V. and et al. (2009). Rule-based modelling and
model perturbation. In Transactions on Computa-
tional Systems Biology XI, pages 116–137. Springer.
Faeder, J. R., Blinov, M. L., and Hlavacek, W. S. (2009).
Rule-Based Modeling of Biochemical Systems with
BioNetGen, pages 113–167. Humana Press, NJ.
Gautam., V., Long., S., and Orponen., P. (2020). Ruledsd:
A rule-based modelling and simulation tool for dna
strand displacement systems. In Proceedings of the
13th International Joint Conference on Biomedical
Engineering Systems and Technologies - Volume 3:
BIOINFORMATICS,, pages 158–167.
Glotzer, S. C., Solomon, M. J., and Kotov, N. A. (2004).
Self-assembly: From nanoscale to microscale col-
loids. AIChE Journal, 50(12):2978–2985.
Harris, L. A. e. a. (2015). BioNetGen 2.2: Advances
in Rule-Based Modeling. arXiv e-prints, page
arXiv:1507.03572.
Jiang, S. and et al. (2017). Understanding the elemen-
tary steps in dna tile-based self-assembly. ACS Nano,
11(9):9370–9381.
Kamerlin, S. C. L. and Warshel, A. (2011). Multiscale
modeling of biological functions. Phys. Chem. Chem.
Phys., 13:10401–10411.
Krishnan, Y. and Simmel, F. C. (2011). Nucleic acid based
molecular devices. Angewandte Chemie International
Edition, 50(14):3124–3156.
Kuzyk, A., Laitinen, K. T., and T
¨
orm
¨
a, P. (2009). DNA
origami as a nanoscale template for protein assembly.
Nanotechnology, 20(23):235305.
Lakin, M. R. and et al. (2011). Visual dsd: a design
and analysis tool for dna strand displacement systems.
Bioinformatics, 27:3211–3213.
Li, J. and et al. (2011). Self-assembled multivalent dna
nanostructures for noninvasive intracellular delivery
of immunostimulatory cpg oligonucleotides. ACS
Nano, 5(11):8783–9.
Lund, K. and et al. (2010). Molecular robots guided by
prescriptive landscapes. Nature, 465(7295):206–209.
Mohammed, A., Czeizler, E., and Czeizler, E. (2017).
Computational modelling of the kinetic tile assembly
model using a rule-based approach. Theoretical Com-
puter Science, 701:203 – 215. At the intersection of
computer science with biology, chemistry and physics
- In Memory of Solomon Marcus.
Patitz, M. J. (2011). Simulation of self-assembly in the
abstract tile assembly model with ISU TAS. CoRR,
abs/1101.5151.
Poppleton, E. and et al. (2020). Design, optimization and
analysis of large DNA and RNA nanostruc through in-
teractive visualization, editing and molecular simula-
tion. Nucleic Acids Research, 48(12):e72–e72.
Seeman, N. (2003). Dna in a material world. Nature Cell
Biology, 421(6921):427–431.
Sneddon, M. and et al. (2011). Efficient modeling, simula-
tion and coarse-graining of biological complexity with
nfsim. Nat Methods, 8(2):177–183.
Spencer, F., Sanwal, U., and Czeizler, E. (2021). VDNA-
Lab. Available at https://github.com/Frankie-Spencer/
/virtual dna lab , version 1.0.
Sweeney, B. and et al. (2008). Exploring the parameter
space of complex self-assembly through virus capsid
models. Biophysical Journal, 94(3):772 – 783.
Thomas, M. and Schwartz, R. (2017). Quantitative compu-
tational models of molecular self-assembly in systems
biology. Physical Biology, 14(3):035003.
Yang, J. and Hlavacek, W. S. (2011). The efficiency of
reactant site sampling in network-free simulation of
rule-based models for biochemical systems. Physical
Biology, 8(5):055009.
SIMULTECH 2021 - 11th International Conference on Simulation and Modeling Methodologies, Technologies and Applications
294