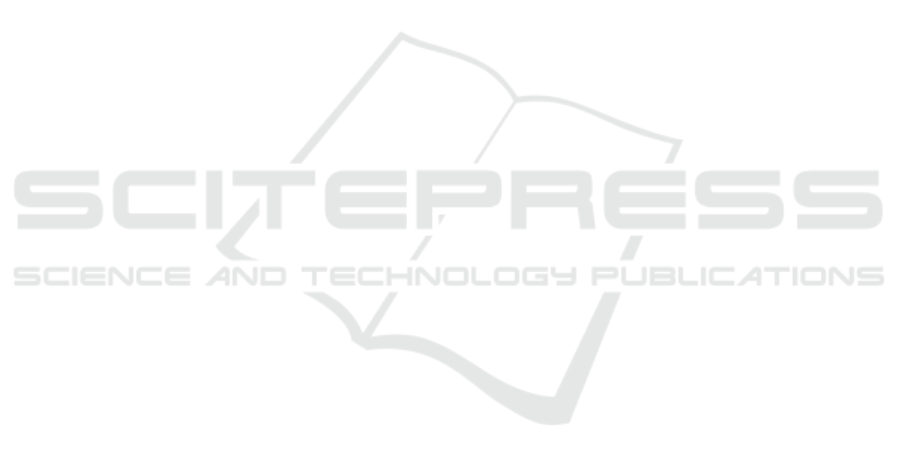
Conference on Web Intelligence and Intelligent Agent
Technology, volume 1, pages 220–226. IEEE.
Liu, H., Li, S., Zhou, G., Huang, C.-R., and Li, P. (2013).
Joint modeling of news reader’s and comment writer’s
emotions. In 51st Annual Meeting of the Association
for Computational Linguistics, pages 511–515.
Quan, X., Wang, Q., Zhang, Y., Si, L., and Wenyin,
L. (2015). Latent discriminative models for so-
cial emotion detection with emotional dependency.
ACM Transactions on Information Systems (TOIS),
34(1):1–19.
Ramya, R., Kishore, N., Sejal, D., Venugopal, K., Iyen-
gar, S., and Patnaik, L. (2019). Predicting social
emotions based on textual relevance for news docu-
ments. In 2019 IEEE International WIE Conference
on Electrical and Computer Engineering (WIECON-
ECE), pages 1–4. IEEE.
Ramya, R., Madhura, K., Sejal Venugopal, K., Iyengar, S.,
and Patnaik, L. (2020). EPNDR: Emotion prediction
for news documents based on readers’ perspectives.
International Journal of Scientific & Technology Re-
search, 9:531–539.
Rao, Y. (2015). Contextual sentiment topic model for adap-
tive social emotion classification. IEEE Intelligent
Systems, 31(1):41–47.
Rao, Y., Li, Q., Mao, X., and Wenyin, L. (2014a). Sentiment
topic models for social emotion mining. Information
Sciences, 266:90–100.
Rao, Y., Li, Q., Wenyin, L., Wu, Q., and Quan, X. (2014b).
Affective topic model for social emotion detection.
Neural Networks, 58:29–37.
Rao, Y., Xie, H., Li, J., Jin, F., Wang, F. L., and Li, Q.
(2016). Social emotion classification of short text via
topic-level maximum entropy model. Information &
Management, 53(8):978–986.
Scherer, K. R. and Wallbott, H. G. (1994). Evidence for
universality and cultural variation of differential emo-
tion response patterning. Journal of personality and
social psychology, 66(2):310.
Strapparava, C. and Mihalcea, R. (2007). Semeval-2007
task 14: Affective text. In Fourth International Work-
shop on Semantic Evaluations (SemEval-2007), pages
70–74.
Strapparava, C. and Mihalcea, R. (2008). Learning to iden-
tify emotions in text. In Proceedings of the 2008 ACM
symposium on Applied computing, pages 1556–1560.
Tang, D., Zhang, Z., He, Y., Lin, C., and Zhou, D. (2019).
Hidden topic–emotion transition model for multi-level
social emotion detection. Knowledge-Based Systems,
164:426–435.
Tang, Y.-j. and Chen, H.-H. (2011). Emotion modeling from
writer/reader perspectives using a microblog dataset.
In Workshop on Sentiment Analysis where AI meets
Psychology (SAAIP 2011), pages 11–19.
Tang, Y.-j. and Chen, H.-H. (2012). Mining senti-
ment words from microblogs for predicting writer-
reader emotion transition. In Eighth International
Conference on Language Resources and Evaluation
(LREC’12), pages 1226–1229.
Tokuhisa, R., Inui, K., and Matsumoto, Y. (2008). Emotion
classification using massive examples extracted from
the web. In 22nd International Conference on Com-
putational Linguistics (Coling 2008), pages 881–888.
Turney, P. D. (2002). Thumbs up or thumbs down? seman-
tic orientation applied to unsupervised classification
of reviews. In 40th Annual Meeting on Association
for Computational Linguistics, pages 417–424.
Vu, H. T., Neubig, G., Sakti, S., Toda, T., and Nakamura, S.
(2014). Acquiring a dictionary of emotion-provoking
events. In 14th Conference of the European Chapter of
the Association for Computational Linguistics, pages
128–132.
Wang, C. and Wang, B. (2020). An end-to-end topic-
enhanced self-attention network for social emotion
classification. In Web Conference 2020, pages 2210–
2219.
Wang, C., Wang, B., Xiang, W., and Xu, M. (2019). Encod-
ing syntactic dependency and topical information for
social emotion classification. In 42nd International
ACM SIGIR Conference on Research and Develop-
ment in Information Retrieval, pages 881–884.
Wang, Q., Wu, O., Hu, W., Yang, J., and Li, W. (2011).
Ranking social emotions by learning listwise prefer-
ence. In The First Asian Conference on Pattern Recog-
nition, pages 164–168. IEEE.
Xu, R., Ye, L., and Xu, J. (2013a). Reader’s emotion pre-
diction based on weighted latent dirichlet allocation
and multi-label k-nearest neighbor model. Journal of
Computational Information Systems, 9(6):2209–2216.
Xu, R., Zou, C., and Xu, J. (2013b). Reader’s emotion pre-
diction based on partitioned latent dirichlet allocation
model. In International Conference on Internet Com-
puting and Big Data, pages 133–137.
Yadollahi, A., Shahraki, A. G., and Zaiane, O. R. (2017).
Current state of text sentiment analysis from opinion
to emotion mining. ACM Computing Surveys (CSUR),
50(2):1–33.
Yang, Y., Cui, P., Zhu, W., and Yang, S. (2013). User inter-
est and social influence based emotion prediction for
individuals. In 21st ACM International Conference on
Multimedia, MM ’13, pages 785–788.
Yao, Y., Xu, R., Lu, Q., Liu, B., Xu, J., Zou, C., Yuan, L.,
Wang, S., Yao, L., and He, Z. (2014). Reader emotion
prediction using concept and concept sequence fea-
tures in news headlines. In International Conference
on Intelligent Text Processing and Computational Lin-
guistics, pages 73–84. Springer.
Ye, L., Xu, R.-F., and Xu, J. (2012). Emotion predic-
tion of news articles from reader’s perspective based
on multi-label classification. In 2012 international
conference on machine learning and cybernetics, vol-
ume 5, pages 2019–2024. IEEE.
Zhang, Y., Su, L., Yang, Z., Zhao, X., and Yuan, X. (2015).
Multi-label emotion tagging for online news by super-
vised topic model. In Asia-Pacific Web Conference,
pages 67–79. Springer.
DATA 2021 - 10th International Conference on Data Science, Technology and Applications
230