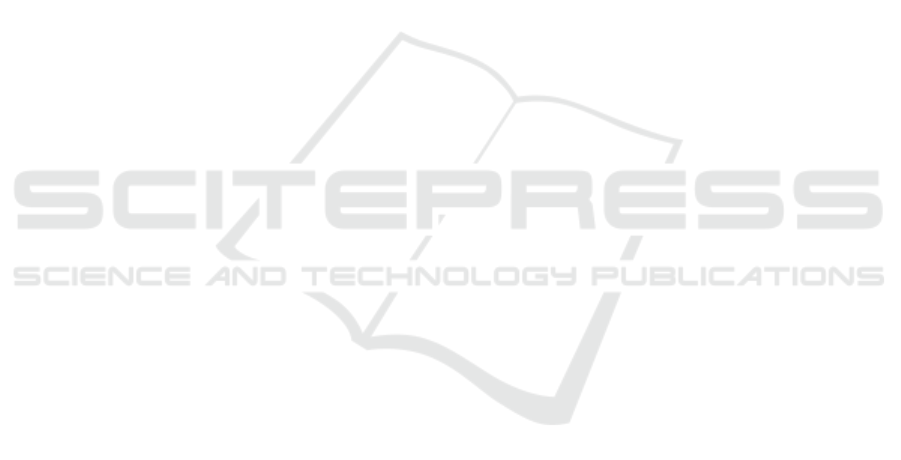
identification results (Fig.13) and prediction results
(Fig.14). It also allows to export an evaluation report
containing all user entries and statistics of different
results throughout the prediction process.
4 CONCLUSION
In this paper, the EPredictor platform, a research tool,
was described and its usability was presented in de-
tail for the community evolution prediction issue. To
the best of our knowledge, no other research tools,
that handle the entire community evolution predic-
tion process, were proposed for predicting commu-
nity evolution in dynamic social networks. We have
presented step by step all the options offered by the
platform in order to make visible the range of func-
tionalities which can be involved for one particular
network. Several experiments were conducted by stu-
dents for their Master dissertation, which proved the
usability of the current version of the EPredictor plat-
form for educational purposes. We meant, through
this paper, to present the EPredictor platform to the
researchers in the field of social network analysis in
order to prove its usability for research purposes. Yet,
the platform is still being developed and new func-
tionalities are constantly incorporated in order to en-
rich the set of methods proposed by the platform for
each step. The EPredictor platform is now available
for academic and other non-commercial purposes.
REFERENCES
Asur, S., Parthasarathy, S., and Ucar, D. (2009). An event-
based framework for characterizing the evolutionary
behavior of interaction graphs. ACM Transactions on
Knowledge Discovery from Data (TKDD), 3(4):1–36.
Br
´
odka, P., Saganowski, S., and Kazienko, P. (2013).
Ged: the method for group evolution discovery in so-
cial networks. Social Network Analysis and Mining,
3(1):1–14.
Dakiche, N., Benbouzid-Si Tayeb, F., Benatchba, K., and
Slimani, Y. (2021). Tailored network splitting for
community evolution prediction in dynamic social
networks. New Generation Computing, pages 1–38.
Dakiche, N., Benbouzid−Si Tayeb, F., Slimani, Y., and Be-
natchba, K. (2018). Tracking community evolution in
social networks: A survey. Information Processing &
Management.
Diakidis, G., Karna, D., Fasarakis-Hilliard, D., Vogiatzis,
D., and Paliouras, G. (2015). Predicting the evolution
of communities in social networks. In Proceedings of
the 5th International Conference on Web Intelligence,
Mining and Semantics, pages 1–6. ACM.
El Moussaoui, M., Agouti, T., Tikniouine, A., and El Ad-
nani, M. (2019). A comprehensive literature review on
community detection: Approaches and applications.
Procedia Computer Science, 151:295–302.
Hall, M., Frank, E., Holmes, G., Pfahringer, B., Reutemann,
P., and Witten, I. H. (2009). The weka data min-
ing software: an update. ACM SIGKDD explorations
newsletter, 11(1):10–18.
Holme, P. and Saram
¨
aki, J. (2012). Temporal networks.
Physics reports, 519(3):97–125.
˙
Ilhan, N. and
¨
O
˘
g
¨
ud
¨
uc
¨
u, S¸. G. (2016). Feature identification
for predicting community evolution in dynamic social
networks. Engineering Applications of Artificial In-
telligence, 55:202–218.
Pavlopoulou, M. E. G., Tzortzis, G., Vogiatzis, D., and
Paliouras, G. (2017). Predicting the evolution of com-
munities in social networks using structural and tem-
poral features. In 2017 12th International Workshop
on Semantic and Social Media Adaptation and Per-
sonalization (SMAP), pages 40–45. IEEE.
Rajita, B., Ranjan, Y., Umesh, C. T., and Panda, S. (2020).
Spark-based parallel method for prediction of events.
Arabian Journal for Science and Engineering, pages
1–17.
Saganowski, S., Br
´
odka, P., Koziarski, M., and Kazienko,
P. (2019). Analysis of group evolution prediction in
complex networks. PloS one, 14(10):e0224194.
Tabassum, S., Pereira, F. S., Fernandes, S., and Gama, J.
(2018). Social network analysis: An overview. Wiley
Interdisciplinary Reviews: Data Mining and Knowl-
edge Discovery, 8(5):e1256.
Takaffoli, M., Rabbany, R., and Za
¨
ıane, O. R. (2014). Com-
munity evolution prediction in dynamic social net-
works. In 2014 IEEE/ACM International Conference
on Advances in Social Networks Analysis and Mining
(ASONAM), pages 9–16. IEEE.
SIMULTECH 2021 - 11th International Conference on Simulation and Modeling Methodologies, Technologies and Applications
302