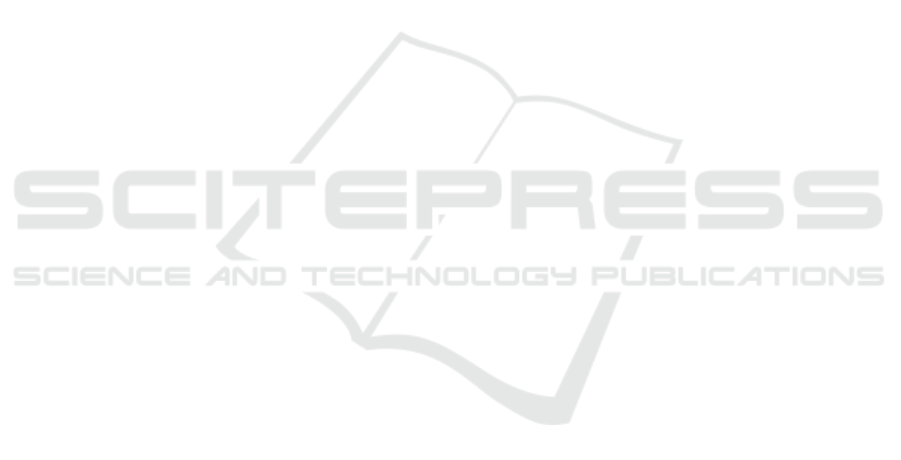
ACKNOWLEDGEMENTS
This work has been funded by the European Union
Horizon 2020 research and innovation programme,
grant agreement 856879.
REFERENCES
Arjovsky, M. and Bottou, L. (2017). Towards principled
methods for training generative adversarial networks.
arXiv preprint arXiv:1701.04862.
Arjovsky, M., Chintala, S., and Bottou, L. (2017). Wasser-
stein generative adversarial networks. In Interna-
tional conference on machine learning, pages 214–
223. PMLR.
Choi, Y., Choi, M., Kim, M., Ha, J.-W., Kim, S., and Choo,
J. (2018). Stargan: Unified generative adversarial net-
works for multi-domain image-to-image translation.
In Proceedings of the IEEE conference on computer
vision and pattern recognition, pages 8789–8797.
Ding, H., Sricharan, K., and Chellappa, R. (2018). Exprgan:
Facial expression editing with controllable expression
intensity. In Proceedings of the AAAI Conference on
Artificial Intelligence, volume 32.
Ebner, N. C., Riediger, M., and Lindenberger, U. (2010).
Faces—a database of facial expressions in young,
middle-aged, and older women and men: Develop-
ment and validation. Behavior research methods,
42(1):351–362.
Gauthier, J. (2014). Conditional generative adversarial nets
for convolutional face generation. Class Project for
Stanford CS231N: Convolutional Neural Networks for
Visual Recognition, Winter semester, 2014(5):2.
Goodfellow, I. J., Pouget-Abadie, J., Mirza, M., Xu, B.,
Warde-Farley, D., Ozair, S., Courville, A., and Ben-
gio, Y. (2014). Generative adversarial networks. arXiv
preprint arXiv:1406.2661.
Gulrajani, I., Ahmed, F., Arjovsky, M., Dumoulin, V., and
Courville, A. (2017). Improved training of wasser-
stein gans. arXiv preprint arXiv:1704.00028.
He, Z., Zuo, W., Kan, M., Shan, S., and Chen, X. (2019).
Attgan: Facial attribute editing by only changing what
you want. IEEE Transactions on Image Processing,
28(11):5464–5478.
Hong, Y., Hwang, U., Yoo, J., and Yoon, S. (2019).
How generative adversarial networks and their vari-
ants work: An overview. ACM Computing Surveys
(CSUR), 52(1):1–43.
Karras, T., Aila, T., Laine, S., and Lehtinen, J. (2017). Pro-
gressive growing of gans for improved quality, stabil-
ity, and variation. arXiv preprint arXiv:1710.10196.
Kossaifi, J., Tzimiropoulos, G., Todorovic, S., and Pantic,
M. (2017). Afew-va database for valence and arousal
estimation in-the-wild. Image and Vision Computing,
65:23–36.
Lang, P. J., Bradley, M. M., and Cuthbert, B. N. (1997).
Motivated attention: Affect, activation, and action. In
Lang, P. J., Simons, R. F., and Balaban, M. T., ed-
itors, Attention and orienting: Sensory and motiva-
tional processes, pages 97–135. Psychology Press.
Lin, J., Xia, Y., Qin, T., Chen, Z., and Liu, T.-Y. (2018).
Conditional image-to-image translation. In Proceed-
ings of the IEEE conference on computer vision and
pattern recognition, pages 5524–5532.
Liu, M.-Y., Breuel, T., and Kautz, J. (2017). Unsupervised
image-to-image translation networks. arXiv preprint
arXiv:1703.00848.
Mehrabian, A. (1995). Framework for a comprehensive de-
scription and measurement of emotional states. Ge-
netic, social, and general psychology monographs,
121(3):339–361.
Mirza, M. and Osindero, S. (2014). Conditional generative
adversarial nets. arXiv preprint arXiv:1411.1784.
Mollahosseini, A., Hasani, B., and Mahoor, M. H. (2019).
Affectnet: A database for facial expression, valence,
and arousal computing in the wild. IEEE Transactions
on Affective Computing, 10(1):18–31.
Radford, A., Metz, L., and Chintala, S. (2015). Unsu-
pervised representation learning with deep convolu-
tional generative adversarial networks. arXiv preprint
arXiv:1511.06434.
Royer, A., Bousmalis, K., Gouws, S., Bertsch, F., Mosseri,
I., Cole, F., and Murphy, K. (2020). Xgan: Unsuper-
vised image-to-image translation for many-to-many
mappings. In Domain Adaptation for Visual Under-
standing, pages 33–49. Springer.
Russell, J. A. and Barrett, L. F. (1999). Core affect, pro-
totypical emotional episodes, and other things called
emotion: dissecting the elephant. Journal of person-
ality and social psychology, 76(5):805.
Sandler, M., Howard, A., Zhu, M., Zhmoginov, A., and
Chen, L. (2018). Mobilenetv2: Inverted residuals and
linear bottlenecks. In 2018 IEEE/CVF Conference
on Computer Vision and Pattern Recognition, pages
4510–4520.
Wang, Y., Dantcheva, A., and Bremond, F. (2018). From
attributes to faces: a conditional generative network
for face generation. In 2018 International Conference
of the Biometrics Special Interest Group (BIOSIG),
pages 1–5. IEEE.
Yi, W., Sun, Y., and He, S. (2018). Data augmentation us-
ing conditional gans for facial emotion recognition. In
2018 Progress in Electromagnetics Research Sympo-
sium (PIERS-Toyama), pages 710–714. IEEE.
Continuous Emotions: Exploring Label Interpolation in Conditional Generative Adversarial Networks for Face Generation
139