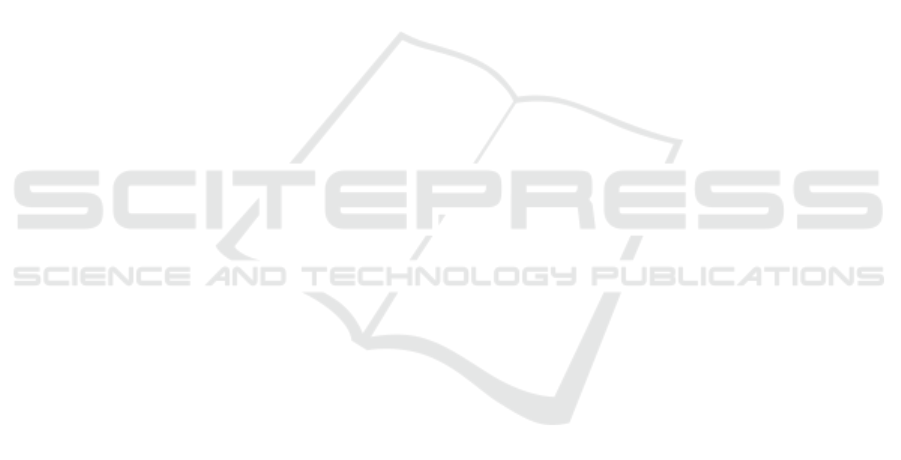
Joint Conference on Neural Networks (IJCNN), pages
1–7.
Chen, L., Papandreou, G., Kokkinos, I., Murphy, K., and
Yuille, A. L. (2018). Deeplab: Semantic image seg-
mentation with deep convolutional nets, atrous convo-
lution, and fully connected crfs. IEEE Trans. Pattern
Anal. Mach. Intell., 40(4):834–848.
Chen, Y., Li, W., Chen, X., and Gool, L. V. (2019). Learn-
ing semantic segmentation from synthetic data: A ge-
ometrically guided input-output adaptation approach.
In Proceedings of the IEEE/CVF Conference on Com-
puter Vision and Pattern Recognition (CVPR).
Choi, J., Kim, T., and Kim, C. (2019). Self-ensembling
with gan-based data augmentation for domain adapta-
tion in semantic segmentation. In Proceedings of the
IEEE/CVF International Conference on Computer Vi-
sion (ICCV).
Cordts, M., Omran, M., Ramos, S., Rehfeld, T., Enzweiler,
M., Benenson, R., Franke, U., Roth, S., and Schiele,
B. (2016). The cityscapes dataset for semantic urban
scene understanding. In Proc. of the IEEE Conference
on Computer Vision and Pattern Recognition (CVPR).
Dai, D. and Gool, L. (2018). Dark model adaptation: Se-
mantic image segmentation from daytime to night-
time. 2018 21st International Conference on Intel-
ligent Transportation Systems (ITSC), pages 3819–
3824.
Gatys, L. A., Ecker, A. S., and Bethge, M. (2015). A neural
algorithm of artistic style.
Gatys, L. A., Ecker, A. S., and Bethge, M. (2016). Im-
age style transfer using convolutional neural networks.
2016 IEEE Conference on Computer Vision and Pat-
tern Recognition (CVPR), pages 2414–2423.
Hoffman, J., Tzeng, E., Park, T., Zhu, J.-Y., Isola, P.,
Saenko, K., Efros, A. A., and Darrell, T. (2018).
Cycada: Cycle consistent adversarial domain adapta-
tion. In International Conference on Machine Learn-
ing (ICML).
Huang, X., Liu, M.-Y., Belongie, S., and Kautz, J. (2018).
Multimodal unsupervised image-to-image translation.
In ECCV.
Isola, P., Zhu, J.-Y., Zhou, T., and Efros, A. A. (2017).
Image-to-image translation with conditional adversar-
ial networks. CVPR.
Johnson, J., Alahi, A., and Fei-Fei, L. (2016). Perceptual
losses for real-time style transfer and super-resolution.
In European Conference on Computer Vision.
Kingma, D. P. and Ba, J. (2017). Adam: A method for
stochastic optimization.
Lee, D.-H. (2013). Pseudo-label : The simple and efficient
semi-supervised learning method for deep neural net-
works. ICML 2013 Workshop : Challenges in Repre-
sentation Learning (WREPL).
Liu, M.-Y., Breuel, T., and Kautz, J. (2017). Unsuper-
vised image-to-image translation networks. In Guyon,
I., Luxburg, U. V., Bengio, S., Wallach, H., Fer-
gus, R., Vishwanathan, S., and Garnett, R., editors,
Advances in Neural Information Processing Systems,
volume 30. Curran Associates, Inc.
Nizan, O. and Tal, A. (2020). Breaking the cycle - col-
leagues are all you need. In IEEE conference on com-
puter vision and pattern recognition (CVPR).
Odena, A., Dumoulin, V., and Olah, C. (2016). Deconvolu-
tion and checkerboard artifacts. Distill.
Pizzati, F., Charette, R. d., Zaccaria, M., and Cerri, P.
(2020). Domain bridge for unpaired image-to-image
translation and unsupervised domain adaptation. In
Proceedings of the IEEE/CVF Winter Conference on
Applications of Computer Vision (WACV).
Sakaridis, C., Dai, D., and Van Gool, L. (2019). Guided cur-
riculum model adaptation and uncertainty-aware eval-
uation for semantic nighttime image segmentation. In
The IEEE International Conference on Computer Vi-
sion (ICCV).
Sakaridis, C., Dai, D., and Van Gool, L. (2020).
Map-guided curriculum domain adaptation and
uncertainty-aware evaluation for semantic nighttime
image segmentation. IEEE Transactions on Pattern
Analysis and Machine Intelligence.
Simonyan, K. and Zisserman, A. (2015). Very deep con-
volutional networks for large-scale image recognition.
In Bengio, Y. and LeCun, Y., editors, 3rd Interna-
tional Conference on Learning Representations, ICLR
2015, San Diego, CA, USA, May 7-9, 2015, Confer-
ence Track Proceedings.
Srivastava, N., Hinton, G., Krizhevsky, A., Sutskever, I.,
and Salakhutdinov, R. (2014). Dropout: a simple way
to prevent neural networks from overfitting. The jour-
nal of machine learning research, 15(1):1929–1958.
Tarvainen, A. and Valpola, H. (2017). Mean teachers are
better role models: Weight-averaged consistency tar-
gets improve semi-supervised deep learning results. In
Guyon, I., Luxburg, U. V., Bengio, S., Wallach, H.,
Fergus, R., Vishwanathan, S., and Garnett, R., editors,
Advances in Neural Information Processing Systems,
volume 30. Curran Associates, Inc.
van Engelen, J. E. and Hoos, H. H. (2020). A sur-
vey on semi-supervised learning. Machine Learning,
109(2):373–440.
Verma, V., Lamb, A., Kannala, J., Bengio, Y., and Lopez-
Paz, D. (2019). Interpolation consistency training
for semi-supervised learning. In Proceedings of the
Twenty-Eighth International Joint Conference on Arti-
ficial Intelligence, IJCAI-19, pages 3635–3641. Inter-
national Joint Conferences on Artificial Intelligence
Organization.
Xie, Q., Hovy, E. H., Luong, M., and Le, Q. V. (2019). Self-
training with noisy student improves imagenet classi-
fication. CoRR, abs/1911.04252.
Yazici, Y., Foo, C., Winkler, S., Yap, K., Piliouras, G.,
and Chandrasekhar, V. (2019). The unusual effective-
ness of averaging in GAN training. In 7th Interna-
tional Conference on Learning Representations, ICLR
2019, New Orleans, LA, USA, May 6-9, 2019. Open-
Review.net.
Yu, F., Xian, W., Chen, Y., Liu, F., Liao, M., Madhavan,
V., and Darrell, T. (2018). BDD100K: A diverse driv-
ing video database with scalable annotation tooling.
CoRR, abs/1805.04687.
DeLTA 2021 - 2nd International Conference on Deep Learning Theory and Applications
46