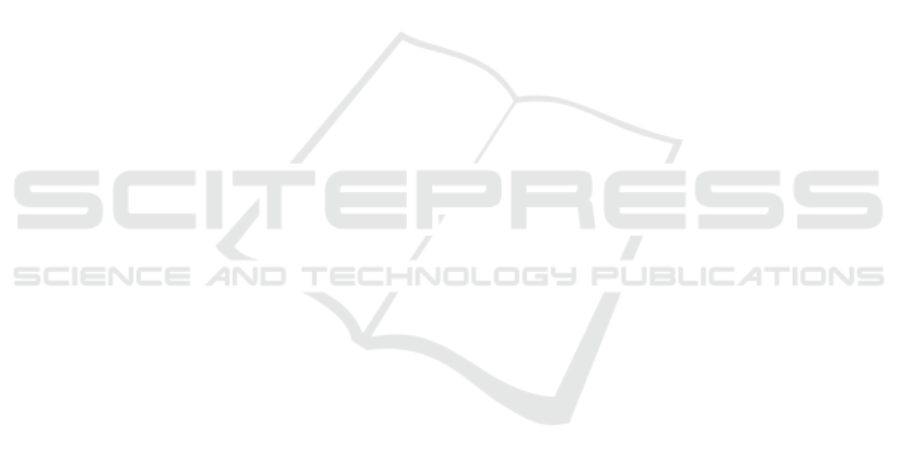
Li, N., Li, T., and Venkatasubramanian, S. (2007a).
t-closeness: Privacy beyond k-anonymity and l-
diversity. In 2007 IEEE 23rd International Confer-
ence on Data Engineering, pages 106–115.
Li, N., Li, T., and Venkatasubramanian, S. (2007b).
t-closeness: Privacy beyond k-anonymity and l-
diversity. In 2007 IEEE 23rd ICDE, pages 106–115.
Machanavajjhala, A., Kifer, D., Gehrke, J., and Venkita-
subramaniam, M. (2007). l-diversity: Privacy beyond
k-anonymity. ACM Transactions on Knowledge Dis-
covery from Data (TKDD), 1(1):3.
McCallister, E., Grance, T., and Scarfone, K. (2010). Guide
to protecting the confidentiality of personally identifi-
able information (pii). NIST Special Publication 800-
122, National Institute of Standards and Technology,
2010.
McSherry, F. and Talwar, K. (2007). Mechanism design via
differential privacy. In Foundations of Computer Sci-
ence, 2007. FOCS’07. 48th Annual IEEE Symposium
on, pages 94–103. IEEE.
Meyerson, A. and Williams, R. (2004). On the complexity
of optimal k-anonymity. In Proceedings of the twenty-
third ACM SIGMOD-SIGACT-SIGART symposium on
Principles of database systems, pages 223–228. ACM.
Party, T. A. . D. P. W. (2014). Opinion 05/2014 on anonymi-
sation techniques.
Podlesny, N. J., Kayem, A. V., and Meinel, C. (2019a).
Attribute compartmentation and greedy ucc discovery
for high-dimensional data anonymization. In Proceed-
ings of the Ninth ACM Conference on Data and Appli-
cation Security and Privacy, pages 109–119. ACM.
Podlesny, N. J., Kayem, A. V., and Meinel, C. (2019b).
Towards identifying de-anonymisation risks in dis-
tributed health data silos. In International Confer-
ence on Database and Expert Systems Applications.
Springer.
Podlesny, N. J., Kayem, A. V., von Schorlemer, S., and
Uflacker, M. (2018). Minimising information loss
on anonymised high dimensional data with greedy in-
memory processing. In International Conference on
Database and Expert Systems Applications, pages 85–
100. Springer.
Podlesny, N. J., Kayem, A. V. D. M., and Meinel, C.
(2019c). Identifying data exposure across distributed
high-dimensional health data silos through bayesian
networks optimised by multigrid and manifold. In
2019 IEEE Intl Conf on Dependable, Autonomic and
Secure Computing, Intl Conf on Pervasive Intelligence
and Computing, Intl Conf on Cloud and Big Data
Computing, Intl Conf on Cyber Science and Technol-
ogy Congress, DASC/PiCom/CBDCom/CyberSciTech
2019, Fukuoka, Japan, August 5-8, 2019, pages 556–
563.
Project, . G. 1000 genomes project.
Rubinstein, I. and Hartzog, W. (2015). Anonymization and
risk.
Sakpere, A. and Kayem, A. (2014). A state-of-the-art re-
view of data stream anonymization schemes. In Infor-
mation Security in Diverse Computing Environments,
pages 24–50. IGI Global.
Samarati, P. (2001). Protecting respondents identities in mi-
crodata release. IEEE transactions on Knowledge and
Data Engineering, 13(6):1010–1027.
Samarati, P. and Sweeney, L. (1998). Protecting privacy
when disclosing information: k-anonymity and its
enforcement through generalization and suppression.
Technical report, Technical report, SRI International.
Schadt, E. and Chilukuri, S. (2015). The role of big data in
medicine.
Sweeney, L. (2000). Simple demographics often identify
people uniquely. Health (San Francisco), 671:1–34.
Sweeney, L. (2002a). Achieving k-anonymity privacy pro-
tection using generalization and suppression. In-
ternational Journal of Uncertainty, Fuzziness and
Knowledge-Based Systems, 10(05):571–588.
Sweeney, L. (2002b). k-anonymity: A model for protecting
privacy. International Journal of Uncertainty, Fuzzi-
ness and Knowledge-Based Systems, 10(05):557–570.
Vaidya, J. and Clifton, C. (2003). Privacy-preserving k-
means clustering over vertically partitioned data. In
Proceedings of the ninth ACM SIGKDD international
conference on Knowledge discovery and data mining,
pages 206–215. ACM.
Vaidya, J., Kantarc”ı”o”
˘
”g””lu, M., and Clifton, C. (2008).
Privacy-preserving naive bayes classification. The
VLDB Journal?The International Journal on Very
Large Data Bases, 17(4):879–898.
Wong, R. C.-W., Fu, A. W.-C., Wang, K., and Pei, J.
(2007a). Minimality attack in privacy preserving data
publishing. In Proceedings of the 33rd International
Conference on Very Large Data Bases, VLDB ?07,
page 543?554. VLDB Endowment.
Wong, R. C.-W., Fu, A. W.-C., Wang, K., and Pei, J.
(2007b). Minimality attack in privacy preserving data
publishing. In Proceedings of the 33rd International
Conference on Very Large Data Bases, VLDB ?07,
page 543?554. VLDB Endowment.
Wong, R. C.-W., Fu, A. W.-C., Wang, K., and Pei, J. (2009).
Anonymization-based attacks in privacy-preserving
data publishing. ACM Trans. Database Syst., 34(2).
Wong, R. C.-W., Fu, A. W.-C., Wang, K., Yu, P. S., and Pei,
J. (2011). Can the utility of anonymized data be used
for privacy breaches? ACM Trans. Knowl. Discov.
Data, 5(3).
Wong, R. C.-W., Li, J., Fu, A. W.-C., and Wang, K. (2006).
(?, k)-anonymity: An enhanced k-anonymity model
for privacy preserving data publishing. In Proceedings
of the 12th ACM SIGKDD International Conference
on Knowledge Discovery and Data Mining, KDD ?06,
page 754?759, New York, NY, USA. Association for
Computing Machinery.
SECRYPT 2021 - 18th International Conference on Security and Cryptography
714