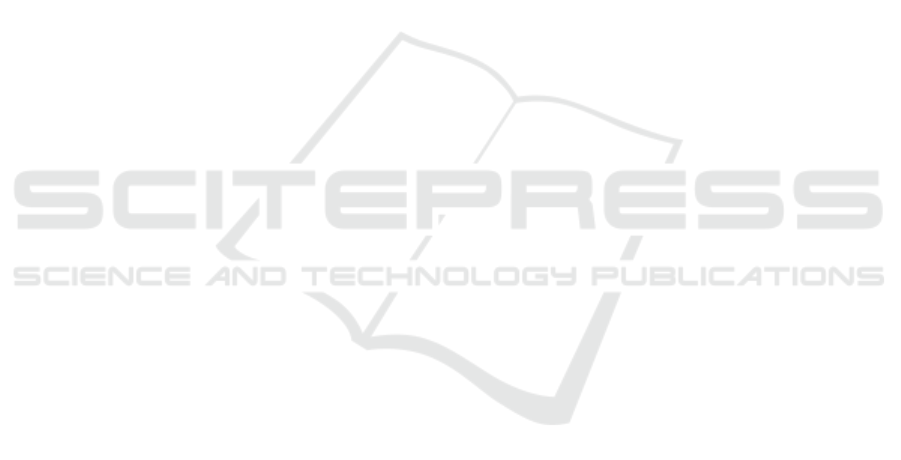
conference on Research and development in informa-
tion retrieval, pages 796–797.
Lawo, D., B
¨
ohm, L., and Esau, M. (2020). Supporting
plant-based diets with ingredient2vec. 7th Interna-
tional Conference on ICT for Sustainability.
Lawo, D., Litz, K., Gromov, C., Schw
¨
arzer, H., and Stevens,
G. (2019). Going vegan: The use of digital media
in vegan diet transition. Proceedings of Mensch und
Computer 2019, pages 503–507.
Lee, J., Sun, M., and Lebanon, G. (2012). A compara-
tive study of collaborative filtering algorithms. arXiv
preprint arXiv:1205.3193.
Lee, T. Q., Park, Y., and Park, Y.-T. (2008). A time-based
approach to effective recommender systems using im-
plicit feedback. Expert systems with applications,
34(4):3055–3062.
Linden, G., Smith, B., and York, J. (2003). Amazon. com
recommendations: Item-to-item collaborative filter-
ing. IEEE Internet computing, 7(1):76–80.
Liphoto, M., Du, C., and Ngwira, S. (2016). A survey on
recommender systems. In 2016 International Confer-
ence on Advances in Computing and Communication
Engineering (ICACCE), pages 276–280. IEEE.
Loebbecke, C. and Picot, A. (2015). Reflections on societal
and business model transformation arising from digiti-
zation and big data analytics: A research agenda. The
Journal of Strategic Information Systems, 24(3):149–
157.
McEachern, A. (2021). What is a repeat customer and why
are they profitable? https://learn.smile.io/blog/repeat-
customers-are-profitable-and-we-can-prove-it.
Miglautsch, J. R. (2000). Thoughts on rfm scoring. Journal
of Database Marketing & Customer Strategy Manage-
ment, 8(1):67–72.
Miranda, T., Claypool, M., Gokhale, A., Mir, T., Murnikov,
P., Netes, D., and Sartin, M. (1999). Combining
content-based and collaborative filters in an online
newspaper. In In Proceedings of ACM SIGIR Work-
shop on Recommender Systems. Citeseer.
Morrison, D. G. and Schmittlein, D. C. (1988). Generaliz-
ing the nbd model for customer purchases: What are
the implications and is it worth the effort? Journal of
Business & Economic Statistics, 6(2):145–159.
Nathanson, T., Bitton, E., and Goldberg, K. (2007). Eigen-
taste 5.0: constant-time adaptability in a recommender
system using item clustering. In Proceedings of
the 2007 ACM conference on Recommender systems,
pages 149–152.
¨
Ozekici, S. and Soyer, R. (2003). Bayesian analysis of
markov modulated bernoulli processes. Mathematical
methods of operations research, 57(1):125–140.
Park, Y. and Lee, T.-Q. (2006). Using temporal informa-
tion in collaborative filtering: An empirical study. In
CSREA EEE, page 316. Citeseer.
Pitts, S. B. J., Ng, S. W., Blitstein, J. L., Gustafson, A.,
and Niculescu, M. (2018). Online grocery shopping:
promise and pitfalls for healthier food and bever-
age purchases. Public health nutrition, 21(18):3360–
3376.
Poggi, N., Muthusamy, V., Carrera, D., and Khalaf, R.
(2013). Business process mining from e-commerce
web logs. In Business process management, pages 65–
80. Springer.
Sarwar, B., Karypis, G., Konstan, J., and Riedl, J. (2001).
Item-based collaborative filtering recommendation al-
gorithms. In Proceedings of the 10th international
conference on World Wide Web, pages 285–295.
Sarwar, B., Karypis, G., Konstan, J., and Riedl, J. (2002).
Incremental singular value decomposition algorithms
for highly scalable recommender systems. In Fifth in-
ternational conference on computer and information
science, volume 1, pages 27–8. Citeseer.
Sichel, H. (1982). Repeat-buying and the generalized in-
verse gaussian–poisson distribution. Journal of the
Royal Statistical Society: Series C (Applied Statis-
tics), 31(3):193–204.
Silver, M. (1989). Repeat-buying: Facts, theory and ap-
plications. Journal of the Royal Statistical Society:
Series A (Statistics in Society), 152(3):420–421.
Stevens, G., Bossauer, P., Neifer, T., and Hanschke, S.
(2017). Using shopping data to design sustainable
consumer apps. In 2017 Sustainable Internet and ICT
for Sustainability (SustainIT), pages 1–3. IEEE.
Talasu, N., Jonnalagadda, A., Pillai, S. S. A., and Rahul, J.
(2017). A link prediction based approach for recom-
mendation systems. In 2017 international conference
on advances in computing, communications and infor-
matics (ICACCI), pages 2059–2062. IEEE.
Tang, T. Y., Winoto, P., and Chan, K. C. (2003). Scaling
down candidate sets based on the temporal feature of
items for improved hybrid recommendations. In IJ-
CAI Workshop on Intelligent Techniques for Web Per-
sonalization, pages 169–186. Springer.
Thorat, P. B., Goudar, R., and Barve, S. (2015). Survey on
collaborative filtering, content-based filtering and hy-
brid recommendation system. International Journal
of Computer Applications, 110(4):31–36.
Tintarev, N. and Masthoff, J. (2006). Similarity for news
recommender systems. In Proceedings of the AH’06
Workshop on Recommender Systems and Intelligent
User Interfaces. Citeseer.
Trinh, G., Rungie, C., Wright, M., Driesener, C., and
Dawes, J. (2014). Predicting future purchases with
the poisson log-normal model. Marketing Letters,
25(2):219–234.
Van Meteren, R. and Van Someren, M. (2000). Using
content-based filtering for recommendation. In Pro-
ceedings of the Machine Learning in the New Informa-
tion Age: MLnet/ECML2000 Workshop, volume 30,
pages 47–56.
Xu, G., Li, L., Zhang, Y., Yi, X., and Kitsuregawa, M.
(2011). Modeling user hidden navigational behavior
for web recommendation. Web Intelligence and Agent
Systems: An International Journal, 9(3):239–255.
Zhang, Z., Kudo, Y., and Murai, T. (2017). Neighbor
selection for user-based collaborative filtering using
covering-based rough sets. Annals of Operations Re-
search, 256(2):359–374.
ICE-B 2021 - 18th International Conference on e-Business
36