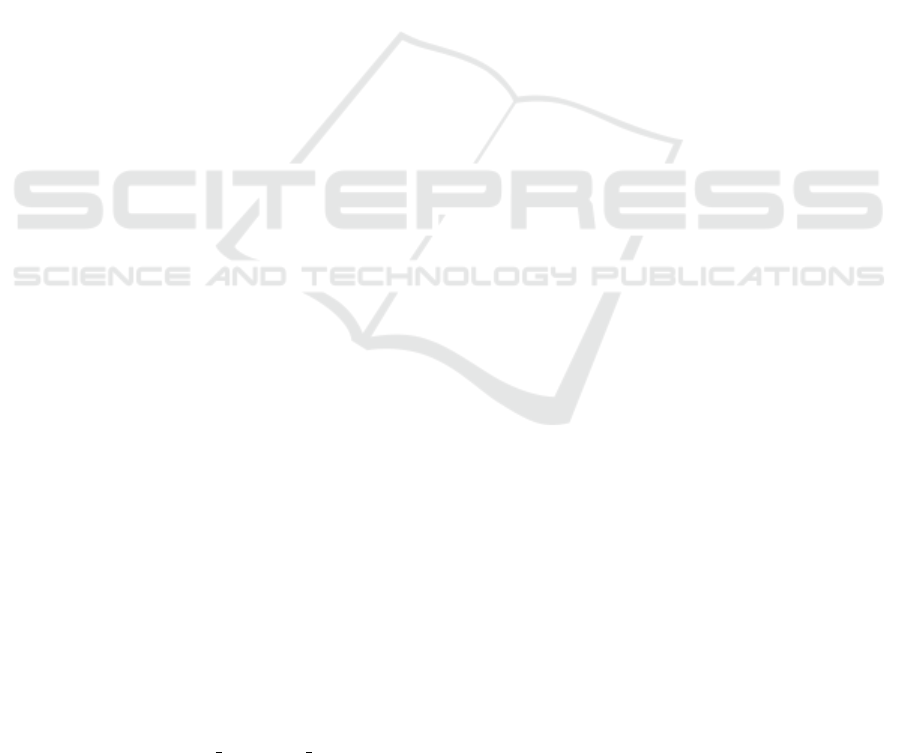
4.4 Orthogonal Collision
In this experiment the test subject and the robot is
on an orthogonal collision path. On fig. 8b the
graph shows the distance from the robot to the per-
son throughout the run. The horizontal lines at 1.2 m
and 0.45 m marks the intrusion of the personal and
intimate spaces respectively. The graph shows that
CASN never intrudes the personal space of the partic-
ipants and while the SNL method does.
With CASN (fig. 5) we force the robot to drive
behind the person, in the direction where the person
came from. In this way the robot and the persons
path will never collide. The SNL method will cre-
ate an inadequate robot movement during an orthog-
onal collision, where the robot often follows the path
of the person. On table 3 we see the results from the
experiment. We again cluster the participants speed
into three categories (slow, medium and fast) to see if
speed makes a difference in performance of the two
methods, and we also report all trials collected. Us-
ing CASN the robot keeps a mean minimum distance
of 1.70 m to the person and never intrudes the per-
sonal space. Using the SNL method the robot keeps a
mean minimum distance of 0.99 m to the person and
intrudes the personal space 12 times. We also see that
CASN keeps similar socially acceptable distance over
the three speeds. The CASN method’s mean min-
imum distances ranges between 1.69-1.72 while the
SNL method ranges between 0.73-1.21. This means
that the robot often drives into the participants per-
sonal space using SNL.
5 CONCLUSION
In this paper we present the method Context-Aware
Social robot Navigation (CASN) for putting mobility
constraints for robots navigating in the proximity of
humans, in the form of costs in costmaps. Inspired
by social space theory by (Hall, 1966) and conversa-
tional group theory by (Kendon, 1990) we put costs
around detected humans in the scene of the robot. We
extend this basic principle to also use the context of
the situation e.g, are the humans in motion, are there
any social interactions between detected humans, and
the task of the robot, in this paper avoiding humans in
its way. Our experiments show that CASN method
makes a mobile robot follow social convention, in
four different navigation scenarios, better than a ROS
open source method social navigation layer
ACKNOWLEDGEMENTS
This research was supported by the project Health-
CAT, funded by the European Fund for regional de-
velopment, and by the project SMOOTH (project
number 6158-00009B) by Innovation Fund Denmark.
REFERENCES
Bordallo, A., Previtali, F., Nardelli, N., and Ramamoorthy,
S. (2015). Counterfactual reasoning about intent for
interactive navigation in dynamic environments. In
2015 IEEE/RSJ International Conference on Intelli-
gent Robots and Systems (IROS), pages 2943–2950.
IEEE.
Hall, E. T. (1966). The hidden dimension.
IFR (2020). International Foundation of Robotics presents
World Robotics Report 2020.
Juel, W. K., Haarslev, F., Kr
¨
uger, N., and Bodenhagen, L.
(2020). An integrated object detection and tracking
framework for mobile robots. pages 513–520.
Kendon, A. (1990). Conducting interaction: Patterns of
behavior in focused encounters, volume 7. CUP
Archive.
Khambhaita, H. and Alami, R. (2020). Viewing robot navi-
gation in human environment as a cooperative activity.
In Robotics Research, pages 285–300. Springer.
Kirby, R., Simmons, R., and Forlizzi, J. (2009). Com-
panion: A constraint-optimizing method for person-
acceptable navigation. In RO-MAN 2009-The 18th
IEEE International Symposium on Robot and Human
Interactive Communication, pages 607–612. IEEE.
Lu, D. V., Hershberger, D., and Smart, W. D. (2014). Lay-
ered costmaps for context-sensitive navigation. In
2014 IEEE/RSJ International Conference on Intelli-
gent Robots and Systems, page 709–715. IEEE.
Marder-Eppstein, E., Berger, E., Foote, T., Gerkey, B., and
Konolige, K. (2010). The office marathon: Robust
navigation in an indoor office environment. In 2010
IEEE International Conference on Robotics and Au-
tomation, pages 300–307.
Mateus, A., Ribeiro, D., Miraldo, P., and Nascimento, J. C.
(2019). Efficient and robust pedestrian detection using
deep learning for human-aware navigation. Robotics
and Autonomous Systems, 113:23–37.
Mead, R. and Matari
´
c, M. J. (2017). Autonomous
human–robot proxemics: socially aware navigation
based on interaction potential. Autonomous Robots,
41(5):1189–1201.
Ram
´
ırez, O. A. I., Khambhaita, H., Chatila, R., Chetouani,
M., and Alami, R. (2016). Robots learning how and
where to approach people. In 2016 25th IEEE inter-
national symposium on robot and human interactive
communication (RO-MAN), pages 347–353. IEEE.
Riek, L. D. (2017). Healthcare robotics. Communications
of the ACM, 60(11):68–78.
Scandolo, L. and Fraichard, T. (2011). An anthropomorphic
navigation scheme for dynamic scenarios. In 2011
IEEE International Conference on Robotics and Au-
tomation, pages 809–814. IEEE.
Context-aware Social Robot Navigation
433