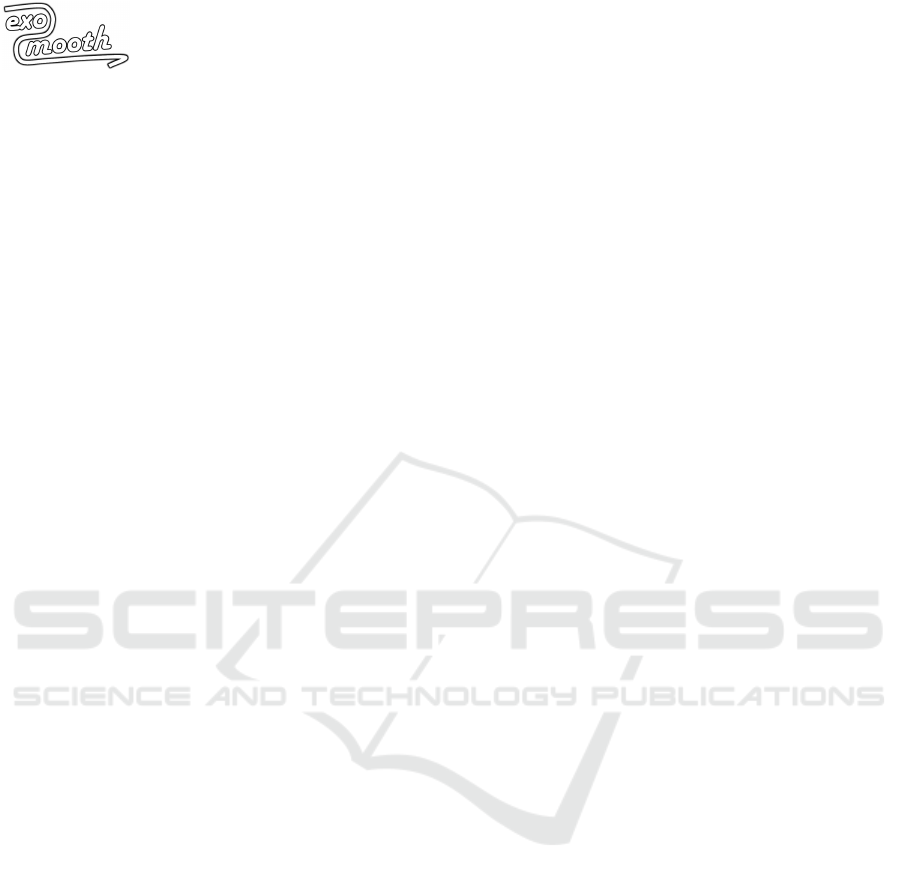
www.eurobench2020.eu) funded by
H2020 Topic ICT 27-2017 under
grant agreement number 779963.
REFERENCES
Afzal, T., Tseng, S.-C., Lincoln, J. A., Kern, M., Francisco,
G. E., and Chang, S.-H. (2020). Exoskeleton-assisted
gait training in persons with multiple sclerosis: a single-
group pilot study. Archives of physical medicine and
rehabilitation, 101(4):599–606.
Alexandrov, A. V., Lippi, V., Mergner, T., Frolov, A. A.,
Hettich, G., and Husek, D. (2017). Human-inspired
eigenmovement concept provides coupling-free sen-
sorimotor control in humanoid robot. Frontiers in
neurorobotics, 11:22.
Argall, B. D. (2013). Machine learning for shared control
with assistive machines. In Proceedings of ICRA Work-
shop on Autonomous Learning: From Machine Learn-
ing to Learning in Real world Autonomous Systems,
Karlsruhe, Germany.
Bergamasco, M., Salsedo, F., and Lucchesi, N. (2011). High
torque limited angle compact and lightweight actuator.
US Patent App. 12/989,095.
Broad, A., Murphey, T., and Argall, B. (2017). Learning
models for shared control of human-machine systems
with unknown dynamics. Robotics: Science and Sys-
tems Proceedings.
Bullinaria, J. A. (2009). Evolved dual weight neural archi-
tectures to facilitate incremental learning. In Proceed-
ings of the International Joint Conference on Compu-
tational Intelligence - Volume 1: ICNC, (IJCCI 2009),
pages 427–434. INSTICC, SciTePress.
Camardella, C., Porcini, F., Filippeschi, A., March-
eschi, S., Solazzi, M., and Frisoli, A. (2021).
Gait Phases Blended Control for Enhancing Trans-
parency on Lower-Limb Exoskeletons. RA-L.
10.1109/LRA.2021.3075368.
Chen, C., Liu, D., Wang, X., Wang, C., and Wu, X. (2017).
An adaptive gait learning strategy for lower limb ex-
oskeleton robot. In 2017 IEEE International Confer-
ence on Real-time Computing and Robotics (RCAR),
pages 172–177.
Goodworth, A. D. and Peterka, R. J. (2012). Sensorimo-
tor integration for multisegmental frontal plane bal-
ance control in humans. Journal of neurophysiology,
107(1):12–28.
Hagan, M. T. and Menhaj, M. B. (1994). Training feedfor-
ward networks with the marquardt algorithm. IEEE
transactions on Neural Networks, 5(6):989–993.
Hauser, H., Neumann, G., Ijspeert, A. J., and Maass, W.
(2007). Biologically inspired kinematic synergies pro-
vide a new paradigm for balance control of humanoid
robots. In 2007 7th IEEE-RAS International Confer-
ence on Humanoid Robots, pages 73–80. IEEE.
Hauser, H., Neumann, G., Ijspeert, A. J., and Maass, W.
(2011). Biologically inspired kinematic synergies en-
able linear balance control of a humanoid robot. Bio-
logical cybernetics, 104(4):235–249.
He, H. and Kiguchi, K. (2007). A study on emg-based
control of exoskeleton robots for human lower-limb
motion assist. In 2007 6th International special topic
conference on information technology applications in
biomedicine, pages 292–295. IEEE.
Hettich, G., Lippi, V., and Mergner, T. (2013). Human-like
sensor fusion mechanisms in a postural control robot.
In Londral, A. E., Encarnacao, P., and Pons, J. L., ed-
itors, Proceedings of the International Congress on
Neurotechnology, Electronics and Informatics. Vilam-
oura, Portugal, pages 152–160.
Hettich, G., Lippi, V., and Mergner, T. (2015). Human-like
sensor fusion implemented in the posture control of a
bipedal robot. In Neurotechnology, Electronics, and
Informatics, pages 29–45. Springer.
Hyun, D. J., Lim, H., Park, S., and Jung, K. (2017). De-
velopment of ankle-less active lower-limb exoskele-
ton controlled using finite leg function state machine.
International Journal of Precision Engineering and
Manufacturing, 18(6):803–811.
Kazerooni, H., Racine, J.-L., Huang, L., and Steger, R.
(2005). On the control of the berkeley lower extremity
exoskeleton (bleex). In Proceedings of the 2005 IEEE
international conference on robotics and automation,
pages 4353–4360. IEEE.
Kurkin, S. A., Pitsik, E. N., Musatov, V. Y., Runnova, A. E.,
and Hramov, A. E. (2018). Artificial neural networks
as a tool for recognition of movements by electroen-
cephalograms. In Proceedings of the 15th International
Conference on Informatics in Control, Automation and
Robotics - Volume 1: ICINCO,, pages 166–171. IN-
STICC, SciTePress.
Lippi, V. (2018). Prediction in the context of a human-
inspired posture control model. Robotics and Au-
tonomous Systems.
Lippi, V. and Ceccarelli, G. (2019). Incremental princi-
pal component analysis: Exact implementation and
continuity corrections. In Proceedings of the 16th
International Conference on Informatics in Control,
Automation and Robotics - Volume 1: ICINCO, pages
473–480. INSTICC, SciTePress.
Lippi, V., Mergner, T., Maurer, C., and Seel, T. (2020).
Performance indicators of humanoid posture control
and balance inspired by human experiments. In
The International Symposium on Wearable Robotics
(WeRob2020) and WearRAcon Europe.
Lippi, V., Mergner, T., Seel, T., and Maurer, C. (2019).
COMTEST project: A complete modular test stand
for human and humanoid posture control and balance.
In 2019 IEEE-RAS 19th International Conference on
Humanoid Robots (Humanoids) Toronto, Canada. Oc-
tober 15-17.
Lippi, V., Mergner, T., Szumowski, M., Zurawska, M. S.,
and Zieli
´
nska, T. (2016). Human-inspired humanoid
balancing and posture control in frontal plane. In
ROMANSY 21-Robot Design, Dynamics and Control,
pages 285–292. Springer, Cham.
Luger, T., Cobb, T. J., Seibt, R., Rieger, M. A., and Stein-
hilber, B. (2019). Subjective evaluation of a passive
lower-limb industrial exoskeleton used during simu-
Identification of Gait Phases with Neural Networks for Smooth Transparent Control of a Lower Limb Exoskeleton
177