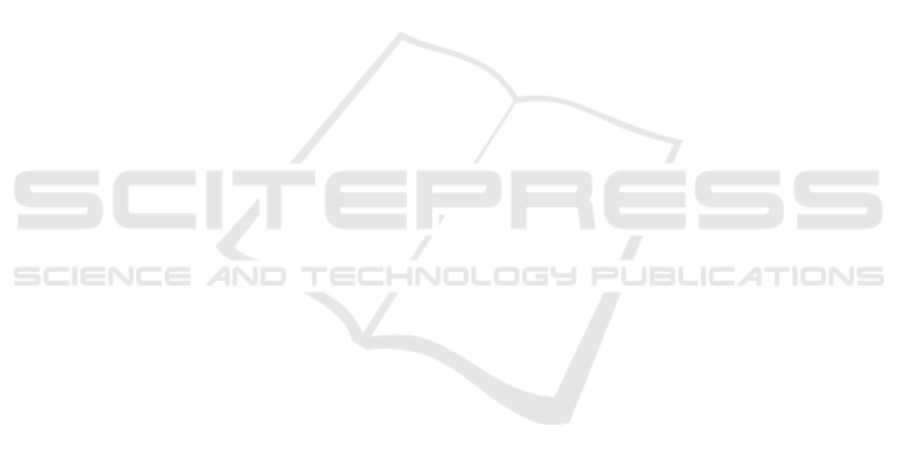
ACKNOWLEDGEMENTS
This work was supported in part by the Spanish
Government through the Project DPI 2016-78361-
R (AEI/FEDER, UE) ”Creaci
´
on de mapas mediante
m
´
etodos de apariencia visual para la navegaci
´
on de
robots”, and in part by the Generalitat Valenciana
through the Grant ACIF/2020/141 and the Project
AICO/2019/031 ”Creaci
´
on de modelos jer
´
arquicos y
localizaci
´
on robusta de robots m
´
oviles en entornos so-
ciales”.
REFERENCES
Alatise, M. B. and Hancke, G. P. (2020). A review on chal-
lenges of autonomous mobile robot and sensor fusion
methods. IEEE Access, 8:39830–39846.
Alcantarilla, P. F., Bartoli, A., and Davison, A. J. (2012).
KAZE features. In Fitzgibbon, A., Lazebnik, S., Per-
ona, P., Sato, Y., and Schmid, C., editors, Computer
Vision – ECCV 2012, pages 214–227, Berlin, Heidel-
berg. Springer Berlin Heidelberg.
Amor
´
os, F., Pay
´
a, L., Mayol-Cuevas, W., Jim
´
enez, L. M.,
and Reinoso, O. (2020). Holistic descriptors of om-
nidirectional color images and their performance in
estimation of position and orientation. IEEE Access,
8:81822–81848.
Aqel, M. O. A., Marhaban, M. H., Saripan, M. I., and Is-
mail, N. B. (2016). Review of visual odometry: types,
approaches, challenges, and applications. Springer-
Plus, 5(1):1897.
Bay, H., Ess, A., Tuytelaars, T., and Van Gool, L. (2008).
Speeded-Up Robust Features (SURF). Computer
Vision and Image Understanding, 110(3):346–359.
Similarity Matching in Computer Vision and Multi-
media.
Joshi, K. and Patel, M. I. (2020). Recent advances in lo-
cal feature detector and descriptor: a literature survey.
International Journal of Multimedia Information Re-
trieval, 9(4):231–247.
Matsuki, H., von Stumberg, L., Usenko, V., St
¨
uckler, J.,
and Cremers, D. (2018). Omnidirectional DSO: Di-
rect Sparse Odometry With Fisheye Cameras. IEEE
Robotics and Automation Letters, 3(4):3693–3700.
Rom
´
an, V., Pay
´
a, L., Cebollada, S., and Reinoso,
´
O. (2020).
Creating incremental models of indoor environments
through omnidirectional imaging. Applied Sciences,
10(18).
Rosten, E. and Drummond, T. (2006). Machine learning
for high-speed corner detection. In Leonardis, A.,
Bischof, H., and Pinz, A., editors, Computer Vision
– ECCV 2006, pages 430–443, Berlin, Heidelberg.
Springer Berlin Heidelberg.
Rublee, E., Rabaud, V., Konolige, K., and Bradski, G.
(2011). ORB: An efficient alternative to SIFT or
SURF. In 2011 International Conference on Com-
puter Vision, pages 2564–2571.
Scaramuzza, D. (2014). Omnidirectional Camera, pages
552–560. Springer US, Boston, MA.
Scaramuzza, D. and Fraundorfer, F. (2011). Visual odom-
etry [tutorial]. IEEE Robotics Automation Magazine,
18(4):80–92.
Tuytelaars, T. and Mikolajczyk, K. (2008). Local in-
variant feature detectors: A survey. Foundations
and Trends
R
in Computer Graphics and Vision,
3(3):177–280.
Valiente, D., Pay
´
a, L., Jim
´
enez, L. M., Sebasti
´
an, J. M.,
and Reinoso,
´
O. (2018). Visual information fusion
through bayesian inference for adaptive probability-
oriented feature matching. Sensors, 18(7).
Valiente Garc
´
ıa, D., Fern
´
andez Rojo, L., Gil Aparicio, A.,
Pay
´
a Castell
´
o, L., and Reinoso Garc
´
ıa, O. (2012). Vi-
sual odometry through appearance-and feature-based
method with omnidirectional images. Journal of
Robotics, 2012.
Williams, C. K. and Rasmussen, C. E. (2006). Gaussian
processes for machine learning, volume 2. MIT press
Cambridge, MA.
Wirth, S., Carrasco, P. L. N., and Codina, G. O. (2013).
Visual odometry for autonomous underwater vehi-
cles. In 2013 MTS/IEEE OCEANS-Bergen, pages 1–6.
IEEE.
Yu, G. and Morel, J.-M. (2011). ASIFT: An Algorithm for
Fully Affine Invariant Comparison. Image Processing
On Line, 1:11–38.
Zhang, Z., Rebecq, H., Forster, C., and Scaramuzza, D.
(2016). Benefit of large field-of-view cameras for vi-
sual odometry. In 2016 IEEE International Confer-
ence on Robotics and Automation (ICRA), pages 801–
808.
Evaluating the Influence of Feature Matching on the Performance of Visual Localization with Fisheye Images
441