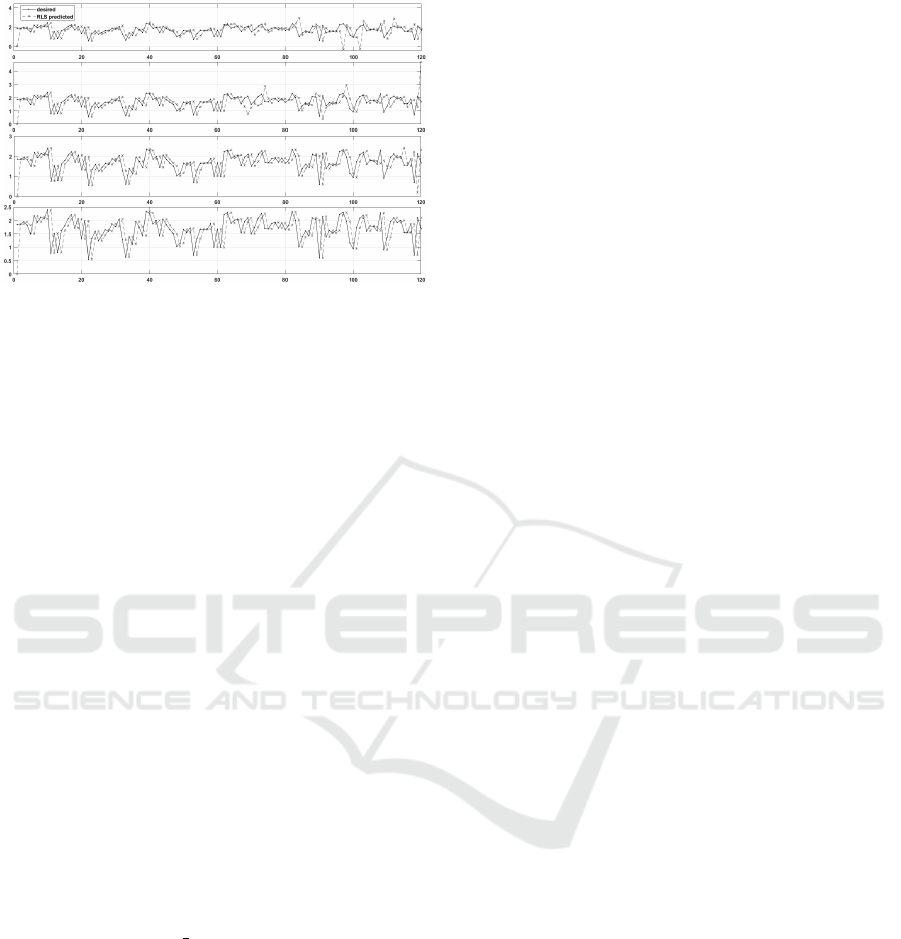
Figure 5: Entropy samples prediction with RLS for K=1,
β=0.1, 0.3, 0.5, 0.7 (number of samples on the x-axis and
entropy values on the y-axis).
In order to select the proper value of K, we take
into account the Partial Auto-Correlation Function
(PACF), defined as the autocorrelation between Et(T )
and Et(T − K) with the linear dependence of Et(T )
on Et(T − 1) through Et(T − K + 1) removed (Box
et al., 2015).
After the analysis of different sets of entropy sam-
ples, we can state that the PACF correlogram, like the
one depicted in Figure 4b helps to select the best val-
ues of K, for which the prediction error is minimised.
In our examples, several largest spikes are obtained:
that is, for each combination of simulation parame-
ters (T , avg speed, l, mobility model and other) a set
K
∗
= {K
1
,K
2
,...,K
r
} of lag values can be obtained,
for which the PACF function has a local maximum.
Additional benefit can be seen that regardless of the
chosen combination of simulation parameters, each
correlogram has a spike for K = 1, that is the entropy
process can always be considered also as a K=1-order
Auto Regressive Process (ARP(1)).
In the next step, we chose K = 1 to confirm that
the RLS algorithm can predict the entropy trend with
an acceptable error. Figure 5 shows the results ob-
tained by considering 120 samples of Et(n
T
k
), with
T = 5s, l = 30m, avg speed = 13.9 m/s. It can be
seen how, in general, the RLS can evaluate future
samples with high accuracy.
5 CONCLUSION AND FUTURE
WORKS
In this paper, we presented an in-depth analysis of
the entropy concept related to mobility in MANETs.
In particular, we underlined the key factors that in-
fluence its trend during host mobility inside a geo-
graphical region. A new way of approaching mo-
bility entropy evaluation has been presented, and a
closed form has been obtained for the description of
its average values, in function of several system pa-
rameters. Also, we provided instructions to predict
future entropy values, obtaining beneficial results re-
garding prediction error. Future works will regard the
application of this analysis to forwarding operations
in MANETs, such as packet routing, novel metrics
definition, system stability analysis and predictive re-
laying, and considering the possibility of using novel
routing approaches based on social networks such as
in (Socievole et al., 2013; Socievole et al., 2014).
REFERENCES
(2019). Openstreetmap. http://www.openstreetmap.org.
Box, G. E., Jenkins, G. M., Reinsel, G. C., and Ljung, G. M.
(2015). Time series analysis: forecasting and control.
John Wiley & Sons.
Cerasoli, C. and Dimarogonas, J. (2008). The general-
ization of information entropy to manet metrics. In
MILCOM 2008-2008 IEEE Military Communications
Conference, pages 1–9. IEEE.
Coon, J. P., Badiu, M.-A., and G
¨
und
¨
uz, D. (2018). On
the conditional entropy of wireless networks. In 2018
16th International Symposium on Modeling and Op-
timization in Mobile, Ad Hoc, and Wireless Networks
(WiOpt), pages 1–6. IEEE.
De Rango, F. and Fotino, M. (2009). Energy efficient
olsr performance evaluation under energy aware met-
rics. In 2009 International Symposium on Perfor-
mance Evaluation of Computer & Telecommunication
Systems, volume 41, pages 193–198. IEEE.
De Rango, F., Iera, A., Molinaro, A., and Marano, S.
(2003). A modified location-aided routing proto-
col for the reduction of control overhead in ad-hoc
wireless networks. In 10th International Conference
on Telecommunications, 2003. ICT 2003., volume 2,
pages 1033–1037. IEEE.
De Rango, F. and Tropea, M. (2009). Swarm intelligence
based energy saving and load balancing in wireless
ad hoc networks. In Proceedings of the 2009 work-
shop on Bio-inspired algorithms for distributed sys-
tems, pages 77–84.
Elujide, I. and Liu, Y. (2020). An entropy-based wlan
channel allocation using channel state information. In
2020 16th International Conference on Wireless and
Mobile Computing, Networking and Communications
(WiMob)(50308), pages 74–79. IEEE.
Fazio, P., Tropea, M., De Rango, F., and Voznak, M. (2016).
Pattern prediction and passive bandwidth manage-
ment for hand-over optimization in qos cellular net-
works with vehicular mobility. IEEE Transactions on
Mobile Computing, 15(11):2809–2824.
Fazio, P., Tropea, M., and Marano, S. (2013). A distributed
hand-over management and pattern prediction algo-
rithm for wireless networks with mobile hosts. In
2013 9th International Wireless Communications and
Routing Optimization in Dynamic Networks based on a New Entropy Metric
107