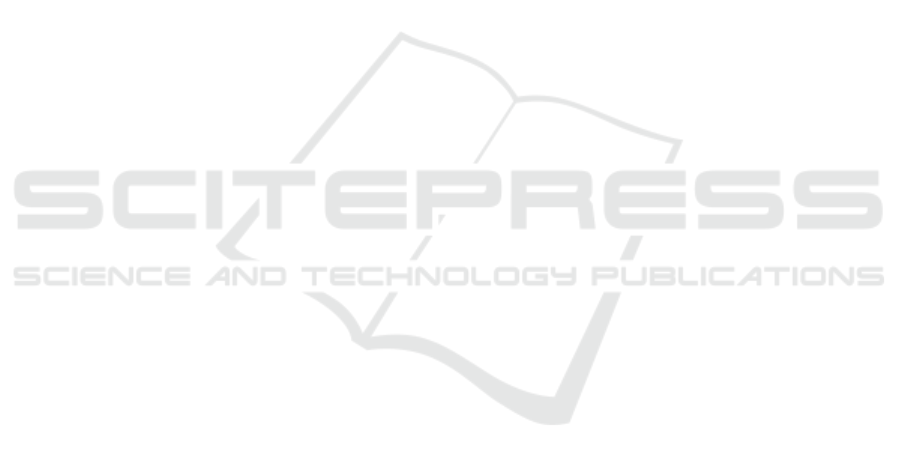
embedding, and to include the attractiveness of AD
groups.
ACKNOWLEDGEMENTS
We acknowledge the support of NVIDIA Corporation
with the donation of a Titan V GPU for this research.
We would also like to thank the Stratosphere team for
their support.
REFERENCES
Amizadeh, S., Matusevych, S., and Weimer, M. (2019).
Learning to solve circuit-SAT: An unsupervised dif-
ferentiable approach. In International Conference on
Learning Representations.
Berg, L. (2019). BlueHive.
Bettke, J. and Stewart, J. (2016). DCEPT: An Open-Source
Honeytoken Tripwire.
Case, D. U. (2016). Analysis of the cyber attack on the
ukrainian power grid. Electricity Information Sharing
and Analysis Center (E-ISAC), 388.
Cimpanu, C. (2020a). Fortune 500 company ntt discloses
security breach.
Cimpanu, C. (2020b). Hackers breached a1 telekom, aus-
tria’s largest isp.
Crabtree, J. (2020). Active directory attacks hit the main-
stream. darkreading.com.
de Barros, A. P. (2003). Res: Protocol anomaly detection
ids - honeypots.
Dowling, S., Schukat, M., and Barrett, E. (2018). Using
reinforcement learning to conceal honeypot function-
ality. In ECML/PKDD.
Faraglia, D. (2012). Faker.
Glorot, X. and Bengio, Y. (2010). Understanding the dif-
ficulty of training deep feedforward neural networks.
In Proceedings of the International Conference on Ar-
tificial Intelligence and Statistics (AISTATS’10).
Grimes, R. A. (2006). Honeypots for Windows. Apress.
Guan, S. and Loew, M. (2019). Evaluation of generative
adversarial network performance based on direct anal-
ysis of generated images. In 2019 IEEE Applied Im-
agery Pattern Recognition Workshop (AIPR).
Hagberg, A. A., Schult, D. A., and Swart, P. J. (2008). Ex-
ploring network structure, dynamics, and function us-
ing. In Varoquaux, G., Vaught, T., and Millman, J.,
editors, Proceedings of the 7th Python in Science Con-
ference, pages 11 – 15, Pasadena, CA USA.
Joyce, J. M. (2011). Kullback-Leibler Divergence, pages
720–722. Springer Berlin Heidelberg, Berlin, Heidel-
berg.
Kaluza, M., De Paolis, C., Amizadeh, S., and Yu, R. (2018).
A neural framework for learning dag to dag transla-
tion. In NeurIPS’2018 Workshop.
Karlin, A. R., Bradley, M., Baldwin, M., and Sagir, S.
(2018). What threats does ata look for?
Kingma, D. P. and Ba, J. (2014). Adam: A method for
stochastic optimization.
Kingma, D. P. and Welling, M. (2014). Auto-encoding vari-
ational bayes.
Leita, C., Mermoud, K., and Dacier, M. (2005). Scriptgen:
an automated script generation tool for honeyd. In
21st Annual Computer Security Applications Confer-
ence (ACSAC’05), pages 12 pp.–214.
Liao, R., Li, Y., Song, Y., Wang, S., Nash, C., Hamil-
ton, W. L., Duvenaud, D., Urtasun, R., and Zemel,
R. (2019). Efficient graph generation with graph re-
current attention networks. In NeurIPS.
Lin, T.-Y., Goyal, P., Girshick, R., He, K., and Doll
´
ar, P.
(2017). Focal loss for dense object detection.
Matsuda, W., Fujimoto, M., and Mitsunaga, T. (2018). De-
tecting apt attacks against active directory using ma-
chine leaning. In 2018 IEEE Conference on Applica-
tion, Information and Network Security (AINS). IEEE.
Metcalf, S. (2015). Red vs. blue: Modern active directory
attacks, detection, & protection.
Microsoft (2015). Advanced Threat Analytics documenta-
tion.
Nurfauzi, R. (2020). Active directory kill chain attack &
defense.
Provos, N. (2003). Honeyd a virtual honeypot daemon.
Simonovsky, M. and Komodakis, N. (2018). Graphvae: To-
wards generation of small graphs using variational au-
toencoders.
Tian, W., Ji, X.-P., Liu, W., Zhai, J., Liu, G., Dai, Y.,
and Huang, S. (2019). Honeypot game-theoretical
model for defending against apt attacks with limited
resources in cyber-physical systems. ETRI Journal,
41(5):585–598.
Valicek, M., Schramm, G., Pirker, M., and Schrittwieser, S.
(2017). Creation and integration of remote high inter-
action honeypots. In 2017 International Conference
on Software Security and Assurance (ICSSA), pages
50–55. IEEE.
Vazarkar, R. (2016). Sharphound.
Whittacker, Z. (2019). Hackers went undetected in citrix’s
internal network for six months.
You, J., Ying, R., Ren, X., Hamilton, W. L., and Leskovec,
J. (2018). Graphrnn: Generating realistic graphs with
deep auto-regressive models.
Zetter, K. (2014). Sony got hacked hard: What we know
and don’t know so far.
Deep Generative Models to Extend Active Directory Graphs with Honeypot Users
147