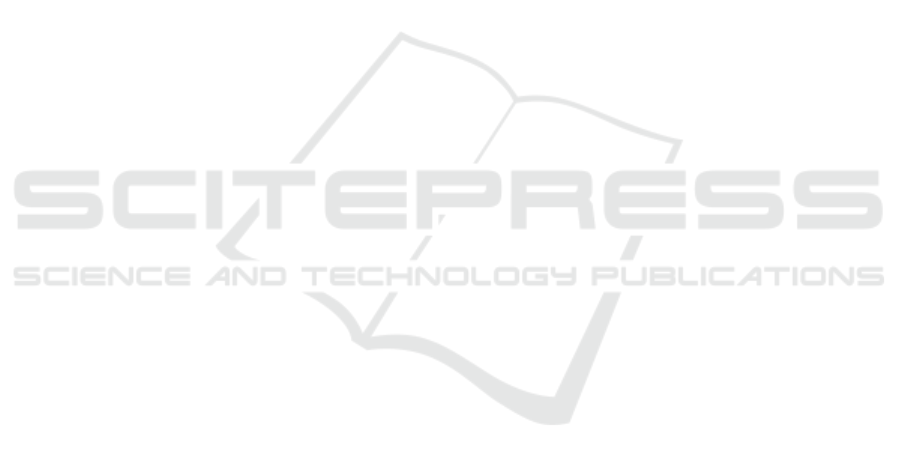
website traffic data. Journal of Artificial Intelligence
Research.
De Choudhury, M., Gamon, M., Counts, S., and Horvitz,
E. (2013). Predicting depression via social media.
In AAAI Conference on Weblogs and Social Media
(ICWSM).
Devlin, J., Chang, M., Lee, K., and Toutanova, K.
(2018). Bert: Pre-training of deep bidirectional trans-
formers for language understanding. arXiv preprint
arXiv:1810.04805.
Dredze, M. (2012). How social media will change public
health. IEEE Intelligent Systems.
Hinds, J. and Joinson, A. (2018). What demographic at-
tributes do our digital footprints reveal? a systematic
review. PloS one.
Ikawa, Y., Enoki, M., and Tatsubori, M. (2012). Location
inference using microblog messages. In International
Conference on World Wide Web.
Jørgensen, A., Hovy, D., and Søgaard, A. (2015). Chal-
lenges of studying and processing dialects in social
media. In Proceedings of the Workshop on Noisy
User-generated Text.
Jungherr, A., Schoen, H., and J
¨
urgens, P. (2016). The medi-
ation of politics through twitter: An analysis of mes-
sages posted during the campaign for the german fed-
eral election 2013. Journal of Computer-Mediated
Communication.
Karami, A. and Bennett, L.and He, X. (2018). Mining pub-
lic opinion about economic issues: Twitter and the us
presidential election. International Journal of Strate-
gic Decision Sciences (IJSDS).
Kim, S., Xu, Q., Qu, L., Wan, S., and Paris, C. (2017). De-
mographic inference on twitter using recursive neural
networks. In Annual Meeting of the Association for
Computational Linguistics.
Kiros, R., Zhu, Y., Salakhutdinov, R., Zemel, R., Urtasun,
R., Torralba, A., and Fidler, S. (2015). Skip-thought
vectors. In Advances in Neural Information Process-
ing Systems.
Levinson, D. (1986). A conception of adult development.
American psychologist.
Mislove, A., Lehmann, S., Ahn, Y., Onnela, J., and Rosen-
quist, J. (2011). Understanding the demographics of
twitter users. In AAAI Conference on Weblogs and So-
cial Media (ICWSM).
Nguyen, D., Gravel, R., and Trieschnigg, D.and Meder, T.
(2013). “ how old do you think i am?” A study of
language and age in twitter. In AAAI Conference on
Weblogs and Social Media (ICWSM).
Nguyen, D., Smith, N., and Rose, C. (2011). Author age
prediction from text using linear regression. In ACL-
HLT workshop on Language Technology for Cultural
Heritage, Social Sciences, and Humanities.
O’Connor, B., Balasubramanyan, R., Routledge, B., and
Smith, N. (2010). From tweets to polls: Linking text
sentiment to public opinion time series. In AAAI Con-
ference on Weblogs and Social Media (ICWSM).
Pennacchiotti, M. and Popescu, A. (2011). A machine
learning approach to twitter user classification. In
AAAI Conference on Weblogs and Social Media
(ICWSM).
Pennington, J., Socher, R., and Manning, C. (2014). Glove:
Global vectors for word representation. In Conference
on empirical methods in natural language processing
(EMNLP).
Peters, M., Neumann, M., Iyyer, M., Gardner, M., Clark,
C., Lee, K., and Zettlemoyer, L. (2018). Deep
contextualized word representations. arXiv preprint
arXiv:1802.05365.
Pokou, Y., Fournier-Viger, P., and Moghrabi, C. (2016). Au-
thorship attribution using small sets of frequent part-
of-speech skip-grams. In International Flairs Confer-
ence.
Preot¸iuc-Pietro, D. and Ungar, L. (2018). User-level race
and ethnicity predictors from twitter text. In Confer-
ence on Computational Linguistics.
Radford, A., Narasimhan, K., Salimans, T., and Sutskever,
I. (2018). Improving language understanding with un-
supervised learning. Technical report, OpenAI.
Rao, D., Paul, M., Fink, C., Yarowsky, D., Oates, T.,
and Coppersmith, G. (2011). Hierarchical bayesian
models for latent attribute detection in social media.
In AAAI Conference on Weblogs and Social Media
(ICWSM).
Rao, D., Yarowsky, D., Shreevats, A., and Gupta, M.
(2010). Classifying latent user attributes in twitter. In
International workshop on Search and Mining User-
generated Contents.
Raschka, S. and Mirjalili, V. (2017). Python Machine
Learning. Packt Publishing Ltd.
Reimers, N. and Gurevych, I. (2019). Sentence-bert: Sen-
tence embeddings using siamese bert-networks. In
Conference on Empirical Methods in Natural Lan-
guage Processing (EMNLP).
Rosenthal, S. and McKeown, K. (2011). Age prediction in
blogs: A study of style, content, and online behavior
in pre-and post-social media generations. In Associa-
tion for Computational Linguistics: Human Language
Technologies.
Sakaki, S., Miura, Y., Ma, X., Hattori, K., and Ohkuma, T.
(2014). Twitter user gender inference using combined
analysis of text and image processing. In Workshop
on Vision and Language.
Schler, J., Koppel, M., Argamon, S., and Pennebaker, J.
(2006). Effects of age and gender on blogging. In
Computational Approaches to Analyzing Weblogs.
Sinnenberg, L., Buttenheim, A., Padrez, K., Mancheno, C.,
Ungar, L., and Merchant, R. (2017). Twitter as a tool
for health research: A systematic review. American
Journal of Public Health.
Sloan, L., Morgan, J., Housley, W., Williams, M., Ed-
wards, A., Burnap, P., and Rana, O. (2013). Knowing
the tweeters: Deriving sociologically relevant demo-
graphics from twitter. Sociological Research Online.
Taniguchi, T., Sakaki, S., Shigenaka, R., Tsuboshita, Y.,
and Ohkuma, T. (2015). A weighted combination of
text and image classifiers for user gender inference. In
Workshop on Vision and Language.
A Comparative Analysis of Classic and Deep Learning Models for Inferring Gender and Age of Twitter Users
57