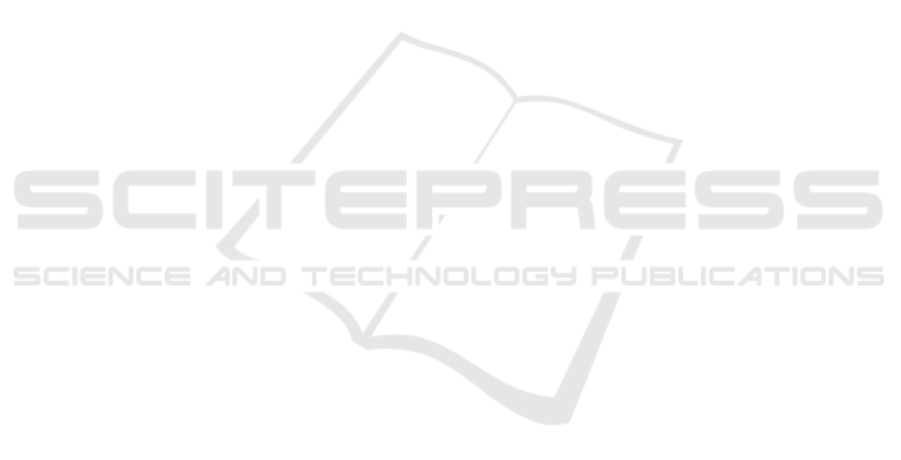
CAROL has three main advantages over vision
guided approaches: (1) higher grasp precision, which
is obtained by utilizing information about the object’s
geometry; (2) no sensory requirements (e. g. camera);
(3) easy adaptability to other domains.
Thus, CAROL provides highly precise grasps as
well as verified collision avoidance for geometrically
complex objects while requiring neither expensive
computing nor complex sensory hardware. In addi-
tion, the simple interchangeability of its major com-
ponents allows for independent further optimization
and adaptation as required in different settings and
target domains.
REFERENCES
Becker, P., Eichmann, C., Roennau, A., and Dillmann, R.
(2020). Automation of post-processing in additive
manufacturing with industrial robots. In 2020 IEEE
16th International Conference on Automation Science
and Engineering (CASE), pages 1578–1583.
Bohg, J. and Kragic, D. (2010). Learning grasping points
with shape context. Robotics and Autonomous Sys-
tems, 58(4):362–377.
Chen, Y., Sun, G., Lin, H., and Chen, S. (2018). Ran-
dom bin picking with multi-view image acquisition
and cad-based pose estimation. In 2018 IEEE Interna-
tional Conference on Systems, Man, and Cybernetics
(SMC), pages 2218–2223.
Depierre, A., Dellandr
´
ea, E., and Chen, L. (2018).
Jacquard: A Large Scale Dataset for Robotic Grasp
Detection. In 2018 IEEE/RSJ International Confer-
ence on Intelligent Robots and Systems (IROS), pages
3511–3516.
Dorn, S., Wolpert, N., and Sch
¨
omer, E. (2020). Voxel-
based General Voronoi Diagram for Complex Data
with Application on Motion Planning. In 2020 IEEE
International Conference on Robotics and Automation
(ICRA), pages 137–143.
Fontanals, J., Dang-Vu, B., Porges, O., Rosell, J., and Roa,
M. A. (2014). Integrated grasp and motion planning
using independent contact regions. In 2014 IEEE-RAS
International Conference on Humanoid Robots, pages
887–893.
Garg, V., Sharma, T., Kumar, A., and Rastogi, V. (2020).
Handaid: A seven DoF Semi-Autonomous Robotic
Manipulator. In 2020 5th International Conference
on Control and Robotics Engineering (ICCRE), pages
37–41.
Gatrell, L. B. (1989). CAD-based grasp synthesis utiliz-
ing polygons, edges and vertexes. In Proceedings,
1989 International Conference on Robotics and Au-
tomation, pages 184–189 vol.1.
Ghalamzan E., A. M., Mavrakis, N., Kopicki, M., Stolkin,
R., and Leonardis, A. (2016). Task-relevant grasp se-
lection: A joint solution to planning grasps and ma-
nipulative motion trajectories. In 2016 IEEE/RSJ In-
ternational Conference on Intelligent Robots and Sys-
tems (IROS), pages 907–914.
Goldfeder, C., Allen, P. K., Lackner, C., and Pelossof, R.
(2007). Grasp Planning via Decomposition Trees. In
Proceedings 2007 IEEE International Conference on
Robotics and Automation, pages 4679–4684.
Gonc¸alves, J. and Lima, P. (2019). Grasp Planning
with Incomplete Knowledge About the Object to
be Grasped. In 2019 IEEE International Confer-
ence on Autonomous Robot Systems and Competitions
(ICARSC), pages 1–6.
G
¨
orner, M., Haschke, R., Ritter, H., and Zhang, J. (2019).
MoveIt! Task Constructor for Task-Level Motion
Planning. In 2019 International Conference on
Robotics and Automation (ICRA), pages 190–196.
Heider, M., P
¨
atzel, D., and H
¨
ahner, J. (2020). Towards
a Pittsburgh-Style LCS for Learning Manufacturing
Machinery Parametrizations. In Proceedings of the
2020 Genetic and Evolutionary Computation Confer-
ence Companion, GECCO ’20, pages 127–128, New
York, NY, USA. Association for Computing Machin-
ery.
Hou, Y., Jia, Z., and Mason, M. T. (2018). Fast Planning
for 3D Any-Pose-Reorienting Using Pivoting. In 2018
IEEE International Conference on Robotics and Au-
tomation (ICRA), pages 1631–1638.
Huang, J., Zhang, F., Dong, X., Yang, R., Xie, J., and
Shang, W. (2020). Vision-guided Dynamic Object
Grasping of Robotic Manipulators. In 2020 IEEE In-
ternational Conference on Mechatronics and Automa-
tion (ICMA), pages 460–465.
Huh, J., Lee, B., and Lee, D. D. (2018). Constrained
sampling-based planning for grasping and manipu-
lation. In 2018 IEEE International Conference on
Robotics and Automation (ICRA), pages 223–230.
Kumra, S. and Kanan, C. (2017). Robotic grasp detec-
tion using deep convolutional neural networks. In
2017 IEEE/RSJ International Conference on Intelli-
gent Robots and Systems (IROS), pages 769–776.
Lee, S. and Lee, Y. (2020). Real-Time Industrial Bin-
Picking with a Hybrid Deep Learning-Engineering
Approach. In 2020 IEEE International Conference
on Big Data and Smart Computing (BigComp), pages
584–588.
Mahler, J., Matl, M., Liu, X., Li, A., Gealy, D., and Gold-
berg, K. (2018). Dex-Net 3.0: Computing Robust
Vacuum Suction Grasp Targets in Point Clouds Using
a New Analytic Model and Deep Learning. In 2018
IEEE International Conference on Robotics and Au-
tomation (ICRA), pages 5620–5627.
Meijer, J., Lei, Q., and Wisse, M. (2017). An empirical
study of single-query motion planning for grasp exe-
cution. In 2017 IEEE International Conference on Ad-
vanced Intelligent Mechatronics (AIM), pages 1234–
1241.
Miller, A. T. and Allen, P. K. (2004). Graspit! a versa-
tile simulator for robotic grasping. IEEE Robotics Au-
tomation Magazine, 11(4):110–122.
Miller, A. T., Knoop, S., Christensen, H. I., and Allen,
P. K. (2003). Automatic grasp planning using shape
CAD-based Grasp and Motion Planning for Process Automation in Fused Deposition Modelling
457